Abstract
The integration of Artificial Intelligence (AI) into Traditional Chinese Medicine (TCM) heralds a transformative era in healthcare, merging the wisdom of ancient practices with the precision of modern technology. TCM, a holistic system with over 2000 years of empirical validation, employs unique diagnostic methods like observation, listening and smelling, questioning, and palpation. AI, mimicking human intelligence through computer systems, enhances these age-old techniques. This convergence is particularly impactful in diagnostic accuracy, treatment efficacy, and prognostic reliability. A key innovation in this synergy is the Acupuncture Diagnosis Machine, which exemplifies AI’s role in refining TCM diagnostics. These machines promise to enhance diagnostic precision by analyzing complex patterns, exemplified by AI’s improved accuracy in tongue image analysis for TCM syndrome differentiation. However, integrating AI, especially in the form of acupuncture diagnosis machines, into TCM presents challenges concerning data quality and ethical considerations. Standardized data sets and ethical guidelines are crucial for advancing AI’s understanding and application of TCM principles. The evolution of TCM through AI, particularly with acupuncture diagnosis machines, is pivotal for healthcare innovation. Collaborative efforts between technologists and TCM practitioners are essential to unlock innovative solutions, pushing medical science boundaries while honoring TCM’s legacy. This collaboration will pave the way for AI-augmented TCM practices, contributing to more systematic, effective, and accessible healthcare systems, with acupuncture diagnosis machines at the forefront of this evolution.
JMIR Med Inform 2024;12:e58491doi:10.2196/58491
Keywords
[acupuncture diagnosis machine], traditional Chinese medicine; TCM; artificial intelligence; AI; diagnosis
Introduction
Traditional Chinese Medicine (TCM), a dynamic and time-honored medical system, boasts a rich history of health and healing practices refined over millennia [1]. Rooted in Chinese philosophy and a deep understanding of the human body’s connection to the natural world, TCM has cultivated a unique array of diagnostic and therapeutic methods. These encompass herbal medicine, acupuncture, and syndrome pattern identification, all based on the fundamental principles of yin and yang and the five-element theory. This holistic approach addresses the body, mind, and spirit in health management. As a traditional system, TCM heavily relies on practitioner experience and often lacks standardized, systematic diagnostic and treatment protocols, potentially hindering its wider acceptance. The rapid advancements in artificial intelligence (AI) offer a significant opportunity to enhance the reliability and precision of TCM diagnostics, thereby boosting the effective application of TCM therapies for patients [2]. AI is increasingly used in modern diagnostics, adept at detecting anomalies in medical imaging, such as identifying early signs of cancer in lung scans [3]. These AI tools can assist physicians in initial diagnoses by analyzing vast amounts of imaging data to pinpoint potential cancer indicators and accurately characterize suspicious lesions, including their shape, size, and molecular characteristics. AI also shows promise in diagnosing mental health conditions, like major depressive disorder, by accurately distinguishing patients from healthy individuals through machine learning [4,5]. This precise characterization is crucial for determining appropriate treatment strategies and predicting disease progression. The integration of AI into TCM, particularly through innovations like acupuncture diagnosis machines, presents both opportunities and challenges. AI can refine the diagnostic process, enabling practitioners to make more accurate disease and patient constitution assessments by analyzing extensive TCM clinical data. It can also aid in creating personalized treatment plans tailored to individual patient needs. However, the incorporation of AI, including acupuncture diagnosis machines, into TCM raises critical concerns about patient privacy and data security. While AI aims to enhance objectivity in diagnosis, the establishment of classification criteria must be carefully refined to minimize subjectivity. Addressing these concerns requires establishing robust ethical standards and stringent privacy safeguards.
Successful AI integration into TCM, particularly for applications like acupuncture diagnosis machines, hinges on interdisciplinary collaboration across medicine, computer science, and data science. Building effective interdisciplinary teams and promoting clear communication are vital for driving innovation in this field. As technology advances, it holds the potential to revolutionize TCM practices, provided ethical implications and the needs of the TCM community are carefully considered. In TCM, diagnosis is paramount, involving detailed patient inquiry and comprehensive physical examinations. This process is crucial for gathering health-related data and facilitates an in-depth evaluation of the patient’s overall health, coupled with a nuanced understanding of the disease’s fundamental characteristics. TCM diagnosis adopts a holistic perspective, assessing both physiological and psychological health aspects, rather than relying solely on objective markers like molecular indicators. The four main TCM diagnostic methods are inspection, auscultation and olfaction, inquiry, and palpation, widely accepted by TCM practitioners globally. Highly skilled TCM physicians can derive diagnostic insights through visual inspection alone, often without needing detailed procedural explanations. Furthermore, nonrational thinking, encompassing intuition, inspiration, and imagination, is considered vital in TCM diagnosis and treatment [6]. Integrating the four diagnostic methods, practitioners assess and synthesize various clinical data to investigate evidence, identify disease root causes, establish treatment strategies, evaluate treatment efficacy, and predict healing progress [7]. AI integration into TCM diagnosis, especially in acupuncture diagnosis machines, respects and complements practitioner intuition and experience. It serves as a clinical research tool to evaluate and verify diagnostic results, not to replace human judgment. AI can analyze complex patient data patterns, augmenting traditional diagnostic methods. For instance, machine learning models can be trained to recognize subtle nuances in patient inquiry responses, enhancing the practitioner’s ability to identify disease roots and tailor treatment plans. AI systems, including components within acupuncture diagnosis machines, can be developed to embody the holistic approach inherent in TCM. By analyzing a broad spectrum of clinical data, AI can offer a more comprehensive health assessment aligning with TCM’s integrated view of a patient’s condition. This supports the diagnostic process and aids in predicting treatment efficacy and healing progress. Moreover, AI, particularly in acupuncture diagnosis machines, can personalize treatments based on individual patient needs and conditions, considering unique body states and responses to therapies. Leveraging AI’s ability to process and learn from large datasets unlocks the potential for personalized TCM treatment. The role of AI in TCM, especially within acupuncture diagnosis machines, is to enhance physician expertise, providing insights and analytics that support holistic and personalized healthcare at TCM’s core. By doing so, AI can improve patient care and ensure TCM’s rich heritage evolves in the modern healthcare landscape.
AI in TCM Diagnosis
Inspection
In TCM practice, inspection primarily gathers diagnostic information through direct observation, assessing patient conditions by scrutinizing physical changes. Unlike Western medicine’s focus on objective, empirical evidence, TCM often bases diagnostic conclusions on subjective practitioner interpretations [8]. Inspection in TCM is broad, encompassing craniofacial observations, with tongue and face diagnoses as primary methods. Tongue inspection, examining shape, size, color, and texture, aids in assessing organ function and medical conditions. Facial expression analysis, aligned with the theory of five zang organs, five elements, and colors, distinguishes facial color changes like green, red, yellow, white, and black, based on yin-yang and five-element principles [9]. Building upon this, technological advancements have enhanced TCM diagnostics. Chen’s neural network-based system [10] marked a significant step, automating TCM diagnosis and treatment with symptom analysis. This platform allows physicians to efficiently access crucial medical records, offering insights into TCM treatment effectiveness for similar conditions. It also streamlines prescription processes, enabling quick dispatch of precise electronic prescriptions to departments and patients. This AI integration improves diagnostic accuracy and facilitates expertise sharing, enhancing TCM care standards. AI is a major topic in medical imaging research, crucial for processing vast medical images and deciphering subtle disease features. AI-based facial and tongue diagnosis shows promising development for acupuncture diagnosis machines. Recently, Liu et al [11] reviewed AI methods in tongue diagnosis, identifying two main obstacles: (1) data set authority and (2) misconceptions about relying solely on single features in traditional tongue diagnosis [11]. AI integration overcomes TCM diagnosis subjectivity, providing a more objective, standardized tongue diagnosis approach, essential for acupuncture diagnosis machines. Advances like multiscale features and prior knowledge incorporation have improved AI-assisted tongue analysis accuracy and reliability. However, robust datasets and performance evaluations are still needed to address existing issues. Intelligent tongue diagnosis is promising, with potential breakthroughs in self-supervised methods, multimodal information fusion, and tongue pathology research, significantly impacting research and clinical practice, particularly for acupuncture diagnosis machines. Addressing these issues requires standardized tongue diagnosis datasets through collaborative efforts involving TCM experts. Leveraging multimodal data in AI is crucial for AI-driven TCM transformation, especially in the context of acupuncture diagnosis machines.
The future of AI in TCM inspection, particularly for acupuncture diagnosis machines, is set to transform traditional diagnostics through research and applications. A primary research direction is developing sophisticated AI algorithms that analyze and interpret tongue and facial diagnostics with detail exceeding human perception. Training these algorithms on high-quality, diverse datasets will enable AI systems to identify subtle patterns indicating underlying health conditions, complementing TCM practitioner expertise and enhancing the capabilities of acupuncture diagnosis machines.
Auscultation and Olfaction
Auscultation and olfaction in TCM use a physician’s hearing and smell to detect patient condition changes. Auscultation detects changes in voice and sounds, while olfaction detects odor changes. TCM theory posits that speech sounds and body odors reflect internal organ physiological and psychological states, making auscultation and olfaction highly valued in TCM. However, objective studies and literature are scarce, possibly due to complex sound acoustic properties (natural noises, similar signals) and diverse volatile organic compound chemical compositions in exhaled gases. These factors have hindered objective research in TCM auscultation and olfaction. Chiu et al [12] introduced quantifiable parameters for TCM auscultation, identifying nonvacuity, qi vacuity, and yin vacuity characteristics, enhancing TCM auscultation practice. More quantitative data on auscultation and further AI application advancements to analyze such data are needed for integration into acupuncture diagnosis machines. AI integration into auscultation and olfaction is facilitated by advanced sensor technologies and audio analysis algorithms. Digital stethoscopes can capture and record body sounds more clearly. AI algorithms then process these sounds, filtering background noise and enhancing relevant audio signals, which can be incorporated into acupuncture diagnosis machines for enhanced diagnostic capabilities. For objective olfactory analysis from a TCM perspective, recent studies exist. A recent study introduced a new odor map characterizing odor quality comparably to skilled human “sniffers” [13]. Algorithms in these odor detection tools have significant potential for quantifying olfactory diagnosis in TCM and could be integrated into future acupuncture diagnosis machines. AI algorithms are then applied to data from these devices to identify specific volatile organic compound profiles associated with different health conditions. This complex task, given the vast number of potential compounds and concentrations, can be addressed by machine learning models, which have shown the ability to handle such complexity and provide objective diagnostic data, potentially enhancing acupuncture diagnosis machines. Therefore, building an AI odor monitoring system, perhaps as a component of acupuncture diagnosis machines, should be a primary focus. Such systems can be developed by selecting specific biomarker reagents [14]. Developing diagnostic molecular biomarkers for olfaction diagnosis in TCM is also a substantial task. These biomarkers should more accurately quantify the TCM olfactory diagnostic process, potentially improving the olfactory diagnostic components of acupuncture diagnosis machines.
However, challenges remain in AI application to auscultation and olfaction, including data quality and standardization, especially for integration into acupuncture diagnosis machines. Auditory and olfactory data collection requires highly accurate sensors and devices. Data quality and standardization are critical for training accurate AI models. Inaccurate or inconsistent data can lead to AI system misjudgments, impacting the reliability of acupuncture diagnosis machines. Another challenge is recognizing extremely complex sound and smell patterns, which vary among individuals. AI needs to recognize and understand these complexities, demanding sophisticated algorithm design and training for effective use in acupuncture diagnosis machines. We need to explore and develop more advanced sensor technologies to improve data collection accuracy and consistency, and use deep learning techniques to enhance AI model ability to recognize complex sound and odor patterns, particularly for applications in acupuncture diagnosis machines.
Inquiry
Interrogation diagnosis, or inquiry diagnosis, involves directly questioning patients about their physiological and psychological feelings. This method gathers information about family history, primary complaints, living conditions, diet, sleep, and other physical condition characteristics. This process allows practitioners to comprehensively understand the patient’s overall health, including factors contributing to their current condition. Thorough patient understanding also helps avoid the influence of prior medical history on treatment decisions. Inquiry aims to provide a holistic patient view, considering not just physical symptoms but also lifestyle and environmental factors affecting health, which could be enhanced with AI-powered acupuncture diagnosis machines. TCM inquiries are mainly based on the “Ten Brief Inquiries,” but now also incorporate past history, allergies, and family history from modern medical records [15]. The GatorTron system, developed by Yang et al [16], enhances clinical narrative use in medical AI systems, improving healthcare delivery and outcomes. However, electronic health record (EHR) analysis for TCM inquiry is underdeveloped, primarily relying on natural language extraction techniques to extract EHR data for building traditional Chinese clinical case knowledge repositories. For example, AI systems can identify TCM-specific symptoms like “fatigue” and “dry mouth” from patient narratives, correlating these symptoms with internal organ imbalances. This advanced recognition aids physicians in assessing patient constitutions and creating personalized treatment plans, potentially integrated into acupuncture diagnosis machines. For chronic conditions, AI facilitates in-depth analysis by sifting through extensive health records to predict disease progression, providing physicians with a solid diagnostic foundation. AI also supports patients needing ongoing care by offering tailored diet and exercise advice, significantly improving quality of life and reducing relapse risks. Smart wearables further empower AI by enabling real-time health data collection, swiftly relayed for AI analysis. This system proactively alerts healthcare providers and patients to emerging health concerns, exemplifying AI’s potential in diagnostics and proactive patient care within TCM, including potential integration with acupuncture diagnosis machines.
Future efforts will focus on confirming large language model (LLM) accuracy, such as GPT-3.5 and GPT-4, in TCM diagnosis [17]. This requires a nuanced approach recognizing TCM terminology’s complexity and richness, particularly for applications in acupuncture diagnosis machines. The first step is collecting comprehensive patient data: symptoms, medical history, lifestyle, and other relevant information. This data must be preprocessed for AI analysis. AI models, especially LLMs, are trained in neurolinguistic programming to understand and interpret human language. In TCM, this involves training models to recognize and analyze specific terminology used in patient inquiries, potentially enhancing the inquiry component of acupuncture diagnosis machines. Subsequently, AI models must be trained to understand the context of TCM terms, recognizing relationships between symptoms and their overall health implications according to TCM principles. However, TCM is practiced globally, with patient inquiries in various languages and dialects. AI models need training on diverse datasets to handle different languages and cultural interpretations of TCM terms, crucial for global applicability of acupuncture diagnosis machines. Significant labeled data and expert input are required for validation. Collaboration with TCM practitioners for data annotation and validation can improve model accuracy, ensuring reliable performance in acupuncture diagnosis machines. In summary, while AI with LLMs shows promise for managing EHRs, TCM inquiry represents a unique knowledge system. Fine-tuning LLMs is essential to transform general-purpose models into specialized models adept at handling TCM EHRs [18]. Future efforts should construct a TCM diagnosis knowledge system. Fine-tuning LLMs for TCM diagnosis based on existing LLM data models will provide AI tools for case analysis in TCM diagnosis, potentially integrated into advanced acupuncture diagnosis machines. AI integration into TCM inquiry is complex, requiring careful consideration of TCM terminology and practice nuances. With the right approach, including ongoing research and TCM expert collaboration, AI can effectively analyze patient data and enhance TCM diagnostic processes, especially within acupuncture diagnosis machines.
Palpation
Pulse diagnosis is a cornerstone of TCM assessment. Palpating the pulse at three wrist positions (“cun,” “guan,” and “chi”) provides a comprehensive understanding of overall health and specific organ states. TCM pulse diagnosis includes approximately 29 pulse types with descriptors like floating and scattered pulses [19]. Pulse diagnosis and AI intersection presents two main challenges, particularly for acupuncture diagnosis machines aiming to incorporate pulse analysis. TCM pulse detection traditionally relies on manual artery palpation, lacking objective standards. AI-driven TCM pulse diagnosis requires addressing two critical issues: developing pulse measurement devices and standardizing pulse detection data. Lan et al [20] created a multipoint sensor device for pulse measurement. Due to pulse detection complexity, prior multipoint sensor methods offered limited information. Pulse measurement device development has seen significant technological advancements recently, mainly in sensor technology innovations and AI algorithm applications, crucial for enhancing acupuncture diagnosis machines. Photovoltaic volumetric pulse wave sensors, based on photoplethysmography, are common in pulse measurement devices. They measure pulse waves by detecting blood flow in microvasculature to obtain physiological parameters like heart rate [21]. Photoplethysmographic sensors are widely used in consumer electronics like smartwatches and fitness trackers. Some devices use pressure sensors for pulse wave measurement, especially in continuous blood pressure monitoring, often embedded in wearable devices for monitoring blood pressure changes over time, which could be integrated with acupuncture diagnosis machines for comprehensive patient data. To address pulse data normalization, AI algorithms preprocess data before analysis, including filtering, denoising, and normalization, ensuring data quality for acupuncture diagnosis machines. AI technology under development aims to improve algorithm cross-device compatibility, enabling consistent analysis of data from different manufacturers and models, promoting data standardization and interoperability, vital for reliable acupuncture diagnosis machines. TCM pulse diagnosis standardization is key to promoting AI technology use in TCM pulse measurements. Unified pulse data and diagnostic standards are needed, along with integrating more diagnostic methods like tongue diagnosis and observation, to develop an integrated TCM diagnostic platform and improve diagnostic comprehensiveness and accuracy, potentially culminating in sophisticated acupuncture diagnosis machines.
Future needs include more powerful multipoint sensing and multimodal detection devices for comprehensive pulse data examination and better quantification, enhancing the capabilities of acupuncture diagnosis machines. A persistent challenge is determining if pulse data from these detectors adequately reflects pulse diagnosis characteristics and improving pulse pattern classification within AI systems used by acupuncture diagnosis machines. To enhance AI precision in interpreting pulse data for future research and development, noncontact pulse measurement techniques show significant advancement, especially for acupuncture diagnosis machines intended for non-invasive assessment. These methods eliminate physical contact, crucial in sensitive situations. For example, polarized multispectral imaging for noncontact heart rate measurement has refined data acquisition accuracy by pioneering new methods for extracting palm pulse waves [22]. This innovation helps establish a standardized pulse data framework, facilitating seamless data sharing and comparisons across devices and systems, beneficial for developing standardized acupuncture diagnosis machines. However, high-quality data hinges on precise labeling, a labor-intensive and costly process. Electrocardiogram data annotation, for instance, requires specialized physicians, introducing variability as different professionals may offer conflicting assessments, complicating data preprocessing. Overcoming these obstacles requires refining data annotation protocols and investing in more efficient, accurate labeling tools. This ensures AI systems, especially those in acupuncture diagnosis machines, are trained on the most reliable data, improving diagnostic capabilities and contributing to AI advancement in healthcare. In summary, detection method development and quantification of detection-based data are bottlenecks in AI-driven pulse diagnosis, which need to be addressed for effective acupuncture diagnosis machines.
AI-Powered Tuina Massage Robot
Tuina massage (Chinese medical massage) is a traditional hands-on TCM manipulation treatment, widely used for conditions like knee osteoarthritis, chronic neck pain, and insomnia [23–25]. Tuina massage serves three primary functions: meridian circulation facilitation, qi and blood circulation harmonization, and immune system augmentation [26–28]. These are crucial for disease prevention, treatment, and overall well-being, and could be complemented by AI-driven diagnostic insights from acupuncture diagnosis machines. AI integration into tuina massage therapy is in its early stages. Efforts are underway to develop intelligent massage equipment and robotics based on TCM tuina to enhance effectiveness, focusing on improving massage robot comfort, intelligence, and safety [29]. Vibration and percussion are the main tuina massage robotics types. These devices offer acupressure techniques, but manual massage from experienced physiotherapists provides additional popular movements like light stroking, stretching, and advanced kneading, which machines currently cannot replicate. Therefore, massage robot development is a significant research focus for meeting greater healthcare demands. Challenges mainly exist in control, structure, and path planning, but ongoing efforts aim to optimize design and functionality, potentially guided by diagnostic data from acupuncture diagnosis machines. For example, incorporating a series-parallel hybrid structure may enhance flexibility while maintaining stiffness and precision [30]. Future research should focus on ergonomics to design high-performance massage robots integrating advanced AI technologies for better control, sensing, and essential functions, possibly informed by AI diagnostics.
In current TCM tuina practice, the Expert Manipulative Massage Automation (EMMA) electronic massager, developed by AiTreat Pte Ltd in Singapore, is widely used. EMMA uses advanced sensor-based technology to identify focus points and adjust pressure levels, delivering precise and effective massage based on muscle feedback. By detecting stiffness and resistance, EMMA pinpoints muscle knots and tension points, applying varying pressure levels based on feedback and user preferences, potentially enhanced by diagnostic insights from acupuncture diagnosis machines. EMMA also incorporates Internet of Things technology for remote control, programming, and updates, enhancing its functionality in “green” Internet of Things applications. EMMA technology uses machine learning algorithms, especially convolutional neural networks in deep learning, to identify and analyze muscle tension patterns, acupuncture point locations, and patient physiological responses. Through training, these algorithms can identify specific treatment points from sensor data to provide customized massage solutions, potentially informed by comprehensive diagnostics from acupuncture diagnosis machines. This pattern recognition capability allows the robotic massage therapist to pinpoint treatment areas, mimicking an experienced massage therapist’s diagnostic process, which could be further refined by integrating with acupuncture diagnosis machines. The robotic masseur can adjust massage intensity and speed based on real-time patient feedback. For example, if sensors detect patient discomfort at a certain pressure level, the AI system can immediately adjust intensity, ensuring treatment comfort and effectiveness, potentially optimizing treatments suggested by acupuncture diagnosis machines. Through advanced data analytics and learning, AI application in EMMA technology accurately identifies treatment points and adjusts massage intensity based on real-time patient feedback. The EMMA massager has shown high acceptability and satisfaction among healthy volunteers, demonstrating its feasibility [31]. Nonetheless, massage robot research still faces challenges, particularly regarding clinical effectiveness. Massage robots are categorized as class-I medical devices, not requiring Food and Drug Administration approval for US marketing [32]. Traditional medical device classification focuses on physical and biological characteristics, while AI device functionality relies more on software and algorithms. Therefore, new classification criteria are needed, considering AI technology specificities and potential risks. Future needs include more standardized regulations to oversee massage robot research [33]. Medical devices process and analyze large patient data amounts, requiring regulations to include stringent data security and privacy protection requirements. Medical device regulations need to incorporate specifications for data collection, storage, processing, and transmission to ensure patient information security and confidentiality, including AI algorithm validation and clinical testing, potentially informed by data from acupuncture diagnosis machines. AI medical device use involves collecting and analyzing large amounts of personal health information, potentially threatening patient privacy. Regulations must ensure patient information collection and use comply with privacy protection standards to prevent unauthorized access and data breaches. This entails validating medical device functionality and therapeutic efficacy to guarantee user safety and efficacy.
We propose the following perspective on tuina robot development, potentially enhanced by diagnostic data from acupuncture diagnosis machines. First, overall tuina technique stability involves variable mechanical parameter stability and resulting morphological changes in mechanical effects during technique operation. These factors include mechanical characteristics like force, speed, frequency, displacement, and kinematic features like limb range of motion, joint angles, and overall movement amplitude. For example, robotic arm dexterity is key to accurately simulating human masseur maneuvers but requires sophisticated mechanical design, including joint flexibility, end-effector versatility, and structural stability. The robot arm’s control system also needs to process large data amounts and make real-time decisions, including trajectory planning, motion control, and complex algorithms for force and position control. Safety mechanisms, like collision detection and response, also need implementation. Consequently, tuina has significant limitations and subjectivity, making objective quantification and accurate efficacy assessment difficult, areas where AI-driven acupuncture diagnosis machines could provide valuable objective data. AI offers unique advantages addressing this issue, primarily through tuina technique digitization (massage robot precision and flexibility). Addressing tuina technique accuracy is a prominent issue potentially requiring diverse AI algorithms to digitize massage techniques and analyze different massage method clinical effects. Chinese massage accuracy largely depends on precise acupoint positioning. Researchers are developing a human body model based on “bone degree and minutes” mechanism in Chinese medicine, realizing 3D acupoint coordinate value calculation through robotics and identifying/tracking human body features using sensors like depth cameras, achieving precise acupoint positioning, which could be integrated with diagnostic data from acupuncture diagnosis machines for enhanced treatment precision. Second, another AI advantage includes personalized healthcare services, potentially informed by comprehensive diagnostics from acupuncture diagnosis machines. Massage robot personalized parameter settings are core parameters for future tuina robots. Individual sensitivity and pressure tolerance vary significantly. People’s comfort and pain thresholds can differ, significantly affecting massage robot experience. AI can analyze user physical condition, health data, and personal preferences to design personalized massage programs, potentially leveraging diagnostic data from acupuncture diagnosis machines. Through advanced intelligent sensor integration, massage robots can monitor user physiological responses, like muscle tension and body temperature, in real-time. Based on this data, massage robots can adjust massage strength, speed, and focus area to overcome “subhealth pain problem” of accurate positioning and efficient massage. Currently, few studies exist on AI nudging robot clinical effectiveness. Patient self-reported pain level changes, pain relief duration, drug dependence reduction, and objective mobility measures (like gait analysis) will be important indicators for future effectiveness evaluation, potentially correlated with diagnostic data from acupuncture diagnosis machines. Considering safety and comfort factors, AI data recording and analysis can also measure tuina robot clinical efficacy.
AI-Directed Acupuncture Manipulation
Acupuncture, a TCM therapeutic technique practiced for millennia, has gained global acceptance and demonstrated significant efficacy for chronic diseases, especially pain-related conditions. This therapy stimulates specific body areas, acupoints, eliciting sensations like soreness, numbness, fullness, or heaviness, known as “De Qi” or achieving qi [34]. Due to inherent subjectivity and experience reliance in traditional acupuncture, parameter-based electroacupuncture is gaining interest to address these limitations [35]. Setting different parameters using electroacupuncture devices can enhance clinical efficacy, facilitating further research, and potentially integrating with diagnostic data from acupuncture diagnosis machines. However, acupuncture efficacy is not universally recognized [36], possibly due to two main reasons: (1) inadequate design and implementation of past clinical research methods leading to lack of clinical evidence; (2) unclear acupuncture mechanism, necessitating higher-quality evidence to elucidate biological mechanisms for informed clinical decision-making [37]. AI and acupuncture integration shows great potential for substantially improving acupuncture prescription and treatment technique precision, especially in acupuncture diagnosis machines. A bibliometric study by Zhou et al [38] demonstrated substantial progress in AI research within acupuncture over the past two decades, with significant contributions from the US and China. However, AI application in acupuncture lacks a clear framework, with scarce systematic research and organization of relevant technologies and application approaches, highlighting the need for focused development of acupuncture diagnosis machines.
Given AI’s unique characteristics and data mining importance in clinical acupuncture practice and manipulation, further research is needed [39]. Clinical trials are costly and limited, and most articles analyzing acupuncture safety and efficacy are low quality and lack comprehensive analyses. Standardized acupuncture point selection protocols are still lacking for many diseases [40]. Therefore, future efforts should focus on standardizing TCM while improving acupuncture randomized controlled trial quality to obtain more and higher-quality clinical data, providing a foundation for AI-based clinical data mining, potentially informing the development of acupuncture diagnosis machines. AI can analyze patient symptoms, signs, and physiological data, comparing them to a large medical knowledge base. Through machine learning and pattern recognition algorithms, AI can help clinicians interpret diagnostic data and provide potential pathological patterns or disease classifications, aiding acupuncturists in developing treatment strategies, and enhancing the diagnostic capabilities of acupuncture diagnosis machines. AI can then analyze large clinical data amounts and research literature to determine the most effective point selection for specific conditions or diseases. A recent study “linked” original studies and 332 systematic evidence evaluations in 20 disease areas using AI analysis techniques to comprehensively improve acupuncture therapy clinical evidence in the Epistemonikos database, constructing 77 evidence matrices [41]. This will facilitate machine learning framework development to predict acupuncture efficacy and patient prognosis, potentially integrated into acupuncture diagnosis machines to predict treatment outcomes. Acupuncture manipulation techniques are crucial for acupuncture therapy efficacy [42]. However, determining optimal stimulation intensity in clinical research is challenging due to technique selection, treatment duration, needling speed, and force [43–45]. Therefore, quantification and standardization of acupuncture manipulation, like needle insertion force, duration, and direction, are essential for achieving clinical efficacy and AI-guided acupuncture manipulation, especially for the development of automated acupuncture diagnosis machines. Addressing operation technique standardization problems, AI technologies, particularly machine learning models and sensor technologies, are used to capture and analyze manual needling operation nuances. Acupuncture robots under development can accurately gauge acupuncture point location by measuring height and sebum thickness, and use ultrasound sensors to control needling depth and speed. These sensors and machine learning models can identify key parameters like needling force, speed, and angle, ensuring treatment standardization and consistency in automated acupuncture diagnosis machines. Sensor technology application in acupuncture focuses on precise control and measurement of needling depth, force, and speed. Robots use ultrasound sensors to control needling depth and speed. By emitting ultrasonic waves and receiving echoes, ultrasonic sensors accurately measure the distance between needle tip and tissue surface, ensuring appropriate needle depth and avoiding unnecessary patient injury. Built-in mechanical sensors also allow robots to automatically adjust needle insertion process based on needle insertion resistance changes, ensuring safe needle insertion, crucial for reliable acupuncture diagnosis machines.
Acupuncture manipulation standardization forms the basis for AI use in acupuncture. With AI technology, we propose three ways to standardize acupuncture: (1) imaging recognition-based standardization of acupuncture practitioner techniques; (2) analysis of parameters derived from practitioner lifting and thrusting techniques using neural network image analysis systems; (3) spatiotemporal feature extraction from acupuncture operation video images using computer vision technology [46]. Additionally, a hybrid model combining 3D convolutional neural networks and neural networks is used to recognize and classify dynamic hand gestures in acupuncture operation videos, enabling quantitative analyses and technical inheritance research for various techniques, which could inform the design of automated acupuncture diagnosis machines. Another approach involves recording practitioner movements and mechanical parameters during acupuncture procedures using 3-axis posture sensors. Davis et al [47] developed force and motion sensor technology (acusensors) to quantify linear and rotational needle movements and force/torque generated during manual needle manipulation. A standardized TCM acupuncture manipulation database was established for motion and force pattern quantification. This data serves as a crucial tool for future AI applications in acupuncture, including acupuncture diagnosis machines. Finally, acupuncture parameters based on other electrophysiological signals have been recorded, showing significant electrophysiological signal differences between acupuncture points and nearby non-acupuncture points, highlighting acupoint electrical specificity [[48](#ref48],49]. This finding provides compelling evidence for TCM theory and parameters for acupuncture stimulation standardization, potentially enhancing the precision of acupuncture diagnosis machines. Furthermore, collaboration between AI experts, acupuncturists, and biomedical engineers is essential for developing and improving acupuncture-related technologies, particularly acupuncture diagnosis machines. AI expert data analysis and intelligent algorithms can help acupuncturists better understand treatment effects and optimize treatment plans. Acupuncturist clinical experience and theoretical knowledge can guide AI experts in developing intelligent systems better meeting clinical needs. Moreover, some prominent conditions or events outside normal circumstances exceed AI abilities. In such cases, acupuncturists can make judgments based on their experience and knowledge. Biomedical engineer technical support subsequently ensures these intelligent systems can be effectively applied in practice. Close collaboration among these three factors is key to promoting technological innovation in acupuncture, improving treatment outcomes, and standardizing and popularizing acupuncture techniques, especially through innovations like acupuncture diagnosis machines. Through this interdisciplinary collaboration, modern technology can better enhance traditional acupuncture medicine value and impact. AI technology can simulate acupuncture operations and provide learning and training support. For example, through virtual and augmented reality technologies, AI can create simulated acupuncture treatment scenarios, allowing learners to practice acupuncture techniques and decision-making processes in a virtual environment, enhancing learning efficiency and reducing risks in actual practice, and paving the way for easier operation of acupuncture diagnosis machines.
Outlook
The future is promising for AI integration into TCM diagnosis, particularly with the development of acupuncture diagnosis machines (Figure 1). TCM diagnosis core involves four fundamental methods: inspection, auscultation and olfaction, inquiry, and palpation. First, inspection can explore AI application in facial and tongue diagnosis. Leveraging image processing techniques, groundbreaking innovations are anticipated in these areas, especially for acupuncture diagnosis machines incorporating visual diagnostics. Due to low contrast and noisy ultrasound images, requiring extensive tongue structure knowledge and ultrasound data interpretation, accurate diagnosis can be challenging. To overcome these challenges, researchers have proposed new deep neural networks, BowNet models [50]. These models leverage encoder-decoder fully convolutional neural network global prediction capabilities and dilated convolution high-resolution extraction features. The models are designed to automatically extract and track tongue contours in real-time ultrasound data. By providing more accurate and objective tongue contour tracking, AI can assist TCM practitioners in making more informed diagnoses and treatment decisions, enhancing the capabilities of acupuncture diagnosis machines. However, digitizing valuable empirical data from TCM facial and tongue diagnosis remains a challenge, although unsupervised machine learning may offer solutions for acupuncture diagnosis machines to learn from unstructured visual data. Addressing TCM experience data digitization often requires seamless collaboration among diverse teams: TCM practitioners, AI experts, and healthcare institutions. This initiative involves partnering with medical facilities to meticulously gather and curate a rich array of TCM clinical case data, encompassing detailed patient complaints, symptomatic expressions, tongue diagnoses, and pulse assessments, which can be used to train and refine acupuncture diagnosis machines. Subsequently, synergy between TCM professionals and AI researchers extends to intricate knowledge structuring. Translating TCM theory, herbal formula, and medicinal property insights into a web-based knowledge graph can greatly enhance this ancient medical wisdom’s accessibility and utility. This graph facilitates nuanced understanding of intricate TCM relationships, underpins intelligent recommendation system development, and assists informed decision-making within TCM, all of which could be integrated into comprehensive acupuncture diagnosis machines. Through these collaborative efforts, the rich TCM experience data tapestry is meticulously incorporated into accessible digital formats, bridging ancient wisdom and modern technological advancements. These initiatives propel TCM modernization and globalization and significantly contribute to broader healthcare innovation, with acupuncture diagnosis machines playing a crucial role. Second, TCM diagnosis through auscultation and olfaction provides new development avenues, especially for enhancing acupuncture diagnosis machines. Specialized AI tools for auditory and olfactory systems can be developed to complement TCM diagnosis. Third, inquiry analysis in TCM diagnosis presents an exciting frontier. Western medicine has seen significant progress in this area with LLMs, and TCM EHRs contain unique TCM diagnosis-specific knowledge graphs. Therefore, fine-tuning is necessary for TCM EHR analysis, potentially leading to AI-powered inquiry modules within acupuncture diagnosis machines. Fourth, pulse diagnosis, a TCM diagnosis cornerstone, requires more advanced pulse detection tool development, crucial for comprehensive acupuncture diagnosis machines. Obtaining substantial pulse data allows for standardized objective pulse analysis. Leveraging machine learning and classification techniques, these data can be aligned with TCM pulse patterns, ultimately achieving AI-driven pulse diagnosis, a key component of future acupuncture diagnosis machines. Quantifiable metrics or benchmarks are instrumental in ensuring AI system high performance, establishing unambiguous objectives, and measuring AI technology integration advancements, particularly in acupuncture diagnosis machines. For example, metrics like diagnostic accuracy and generalization capability can assess AI system proficiency in managing patient data across regions, age groups, and sexes, ensuring efficacy across diverse populations, a critical aspect of widely applicable acupuncture diagnosis machines. Juxtaposing AI system outcomes with seasoned TCM expert diagnoses quantifies AI system precision in pinpointing specific conditions. This quantification is facilitated through statistical indicator computation like sensitivity (true positive rate), specificity (true negative rate), precision, and F1-score. These benchmarks serve as a foundation for continuous AI system refinement, ensuring optimal integration and application within TCM, and driving the improvement of acupuncture diagnosis machines. These development directions have the potential to revolutionize TCM diagnosis, enhancing its accuracy and efficiency, particularly through innovations like acupuncture diagnosis machines.
Figure 1. AI Development Strategies for TCM Diagnosis: AI development strategies based on TCM diagnosis, showcasing unimodal data acquisition and standardization, multimodal data integration, and GPU-powered comprehensive model for enhanced TCM diagnosis and prognosis predictions.
Challenges
Unique challenges exist in AI use in TCM, especially in the context of acupuncture diagnosis machines (Figure 2). First, data quality and availability for acupuncture diagnosis machines are crucial. Successful AI implementation in TCM relies on access to reliable, standardized datasets. High-quality data can potentially promote clinical diagnosis and treatment in precision TCM. However, TCM data collection and digitization can be challenging, and existing dataset quality and interoperability may vary. Varied interpretations of identical conditions among TCM practitioners can lead to divergent diagnostic criteria and terminological applications. Such disparities, coupled with potential errors and biases in manually entered data, can significantly impact AI system learning efficacy, affecting the reliability of acupuncture diagnosis machines. Consequently, establishing standardized TCM diagnostic criteria and terminology glossaries, alongside uniform data entry protocol implementation for TCM practitioners, is essential for mitigating inter-practitioner discrepancies and improving the data quality for training acupuncture diagnosis machines. Furthermore, advanced automated data collection technique use, including image recognition and natural language processing, is instrumental in enhancing collected data quality and precision, contributing to refined analytical capabilities of AI within TCM and improving the accuracy of acupuncture diagnosis machines. Second, bridging the gap between TCM and AI expertise is crucial for the development and effective implementation of acupuncture diagnosis machines. AI technology integration into TCM requires collaboration and communication among TCM practitioners and AI experts. Bridging the gap between these domains is crucial for developing AI algorithms aligning with TCM principles and meeting specific clinical needs, especially in the design of acupuncture diagnosis machines. Measures could be taken to promote collaboration between TCM organizations and technology companies, as well as higher-education institutions, to facilitate AI-driven TCM diagnostic and therapeutic tool development, including acupuncture diagnosis machines. These collaborations will foster innovation and create platforms for TCM students and practitioners to perform technology industry internships. In addition, scholarship and research grant provision is critical for incentivizing and sustaining interdisciplinary scholarship. By allowing students and researchers to delve into TCM and AI convergence, we can accelerate TCM knowledge digitization and the development of innovative technologies like acupuncture diagnosis machines. Third, TCM theories must be interpreted in a computational context for AI applications, including acupuncture diagnosis machines. TCM theories are often complex, based on holistic and individualized perspectives. Translating this knowledge into AI algorithms and computational models is a significant challenge, requiring careful consideration of cultural, philosophical, and theoretical aspects. Many Chinese medicine concepts, like qi, yin and yang, and five elements, are abstract and ambiguous, difficult to describe in precise mathematical language. Therefore, ambiguous logic can be used to address ambiguous TCM concepts, and Bayesian networks can simulate causality and uncertainty in Chinese medicine theory for AI systems, including acupuncture diagnosis machines, to better understand and apply TCM principles. Fourth, notable ethical and safety considerations exist, as with any AI implementation in healthcare, particularly concerning acupuncture diagnosis machines used directly on patients. Ensuring patient privacy, data security, and algorithm decision-making transparency is essential for building trust and ethical practices in AI-supported TCM. Informed patient consent must be obtained before collecting and using patient data, especially data used to train and operate acupuncture diagnosis machines. This includes fully explaining data collection purpose, usage, storage duration, and potential risks. To protect patient privacy, all datasets used for machine learning, particularly in the context of acupuncture diagnosis machines, should be anonymized by removing or encrypting personally identifiable information. During AI system development and deployment, ethical review committees need to be established to ethically review AI system design, implementation, and evaluation, ensuring all activities meet ethical standards, especially for patient-facing technologies like acupuncture diagnosis machines. Fifth, AI integration into TCM necessitates clear regulatory frameworks and policies governing its implementation, including issues related to data protection, algorithm validation, and clinical decision-making, particularly for medical devices like acupuncture diagnosis machines. Sixth, TCM function and efficacy are broadly accepted worldwide; however, the underlying mechanism remains enigmatic, limiting public confidence in TCM and AI-learned precision therapy, including therapies guided by acupuncture diagnosis machines. In summary, implementing and validating AI tools in clinical settings, especially acupuncture diagnosis machines, requires careful planning and rigorous execution. Representative, actionable clinical environments are selected to develop pilot projects. These projects should focus on specific TCM diagnostic tasks, like tongue analysis, pulse recognition, and symptom assessment, relevant to the diagnostic capabilities of acupuncture diagnosis machines. Clinical trials need design and execution to evaluate AI tool performance in real clinical settings. This includes randomized controlled trials and prospective cohort studies to assess AI tool impact on patient outcomes, and specifically evaluate the clinical effectiveness of acupuncture diagnosis machines. Finally, results and experiences will be published and shared through academic journals and conferences to promote communication and learning within the industry and advance the field of AI in TCM, including the development and application of acupuncture diagnosis machines.
Figure 2. Challenges of AI in TCM Diagnosis: Summary of key challenges in integrating AI into TCM diagnosis, including data quality, interdisciplinary expertise gap, TCM theory interpretation, ethical considerations, regulatory frameworks, and the need for mechanistic understanding.
Conclusions
In conclusion, AI integration into TCM shows immense promise for improving diagnosis, including inspection, auscultation and olfaction, inquiry, and palpation, particularly through innovations like acupuncture diagnosis machines. Successful AI integration into TCM is evident in advancements in tongue diagnosis image analysis, intelligent tuina massage systems, and machine learning application to refine treatment protocols based on individual patient data, with acupuncture diagnosis machines representing a significant step forward. Addressing data quality challenges, data set standardization, interdisciplinary collaboration, TCM theory interpretation, ethical considerations, and regulatory frameworks is crucial for responsible and successful AI implementation in TCM, including the development and deployment of acupuncture diagnosis machines. By overcoming these challenges, we can leverage AI power to enhance patient care, personalize treatments, and advance TCM understanding, with acupuncture diagnosis machines playing a central role. Moreover, developing more precise AI models tailored to TCM can create a positive cycle of problem-solving and progress, ultimately leading to better patient care, especially with specialized tools like acupuncture diagnosis machines. Combining TCM wisdom with AI technology can improve patient outcomes and promote TCM integration into modern healthcare systems. Further research into AI’s ability to decode complex diagnostic patterns inherent in TCM is imperative, particularly for enhancing the diagnostic accuracy of acupuncture diagnosis machines. Validating AI-enhanced TCM treatment methods through clinical trials is essential to ensure safety and efficacy, providing empirical support for widespread adoption, including the clinical validation of acupuncture diagnosis machines. As we advance AI integration into TCM, upholding ethical standards prioritizing patient rights, cultural integrity, and data privacy is vital, especially for patient-facing technologies like acupuncture diagnosis machines. Responsible AI use will ensure technological advancements align with TCM principles and practices, safeguarding patient well-being and respecting this ancient medical system’s cultural significance. AI and TCM fusion has the potential to bridge traditional and modern medical practices, enrich global health, and foster cultural exchange. By integrating these two domains, we can create a more comprehensive healthcare system that is both innovative and respectful of historical practices, with acupuncture diagnosis machines as a key example of this integration.
Finally, a call to action is made to all stakeholders (practitioners, researchers, policymakers, and investors) to collaborate and support AI integration into TCM, including the development and refinement of acupuncture diagnosis machines. Through collective efforts, we can harness AI to transform patient care, broaden our understanding of TCM globally, and identify new healthcare horizons that are both deeply rooted in tradition and boldly futuristic, with acupuncture diagnosis machines leading the way.
Acknowledgments
This research was funded by grants from the Ministry of Science and Technology of China and the National Key R&D Program of China (2023YFC2506802). The images in Figures 1 and 2 were created using BioRender [52].
Authors’ Contributions
All the authors participated in the conceptualization, methodology, validation, and writing of the manuscript.
Conflicts of Interest
None declared.
References
[1] World Health Organization. WHO traditional medicine strategy: 2014-2023. World Health Organization; 2013. [CrossRef]
[2] Jiang F, Jiang YZ, Jiang X, Zhang H, Hu Y, Ye C, et al. Artificial intelligence in healthcare: past, present and future. Stroke Vasc Neurol 2017 Dec;2(4):230-243 [CrossRef] [Medline]
[3] Esteva A, Kuprel B, Novoa RA, Ko J, Swani SM, Blau HM, et al. Dermatologist-level classification of skin cancer with deep neural networks. Nature 2017 Feb 02;542(7639):115-118 [CrossRef] [Medline]
[4] Cicero RA, Bielajew C, Marinescu V, Lin JJ, Brodersen KH, Nour MM. Machine learning in psychiatry: promises and pitfalls. Transl Psychiatry 2021 Feb 05;11(1):83 [CrossRef] [Medline]
[5] Yang H, Pasaniuc B, Zaitlen N. Advantages and pitfalls of machine learning in genetics and genomics. Nat Rev Genet 2024 Feb;25(2):105-118 [CrossRef] [Medline]
[6] Liu J. Nonrational thinking in traditional Chinese medicine: a conceptual analysis. J Integr Med 2022 Mar;20(2):105-111 [CrossRef] [Medline]
[7] MacPherson H, Altman DG, Hammerton AC, спонсор STRICTA. STandards for Reporting Interventions in Clinical Trials of Acupuncture (STRICTA): revised recommendations. Acupunct Med 2010 Jun;28(2):83-93 [CrossRef] [Medline]
[8] Barnes LL. Needles, herbs, gods, and ghosts: china, healing, and the west to 1848. Harvard University Press; 2005.
[9] Zhang D, Yang Y, Wang Y, Zhou M, Wang C, Liu L, et al. Facial diagnosis in traditional Chinese medicine: a review. J Tradit Chin Med 2020 Dec;40(6):1032-1040 [Medline]
[10] Chen YW. Neural network-based traditional Chinese medicine diagnosis and treatment system. In: Proceedings of the 2017 2nd International Conference on Robotics and Automation Engineering (ICRAE). 2017 Presented at: 2017 2nd International Conference on Robotics and Automation Engineering (ICRAE); November 18-20, 2017; Shanghai, China p. 181-185. [CrossRef]
[11] Liu J, Gu J, Yuan H, Zhou Z. Artificial intelligence in tongue diagnosis: current status and future prospects. J Tradit Chin Med 2022 Dec;42(6):985-996 [CrossRef] [Medline]
[12] Chiu CW, Chang CC, Huang WF, Lin YC, Tseng PT, Chang KC, et al. Quantifiable parameters for traditional Chinese medicine auscultation: a pilot study. J Tradit Complement Med 2019 Oct;9(4):313-318 [CrossRef] [Medline]
[13] Lee SH, Park S, Kim D, Choi J, Kim JS, Jeon S, et al. Odor map of volatile organic compounds for human olfactory perception. Sci Adv 2023 Dec 08;9(49):eadh6944 [CrossRef] [Medline]
[14] D’Amico A, Pennazza G, Santonico M, Felli M, Sergi M, Biondi R, et al. Electronic nose for disease detection. Sensors (Basel) 2020 Dec 24;21(1):2 [CrossRef] [Medline]
[15] Zou Y, Qian J, Xu Z, Zhang Y, Wang Y, Li Y, et al. Application of artificial intelligence in traditional Chinese medicine inquiry diagnosis. Comput Math Methods Med 2022;2022:5189816 [CrossRef] [Medline]
[16] Yang X, Zhang Y, Chen Q, Lin C, Liu S, Zhang S, et al. GatorTron: cross-domain generalization of large language models for healthcare. arXiv Preprint posted online July 20, 2022. 2022 [FREE Full text] [CrossRef]
[17] Cascella M, Montomoli J, Folino F, Nocerino E, Cuomo A, Della Corte F, et al. Evaluating the feasibility of using large language models, such as ChatGPT, in emergency medicine. Intern Emerg Med 2023 Dec;18(8):2551-2553 [CrossRef] [Medline]
[18] Wang L, Liu S, Chen Z, Jiang J, Liu X, Zhang Y, et al. A literature review of large language models in traditional Chinese medicine. J Integr Bioinform 2024 Jan 26;21(1):20230037 [CrossRef] [Medline]
[19] Kaptchuk TJ. The web that has no weaver: understanding Chinese medicine. McGraw-Hill/Contemporary; 2000.
[20] Lan L, Li Q, Xu D, Zhao Y, Jiang H, Zhang D. Design of a wearable pulse acquisition device based on multipoint measurement. IEEE Sens J 2018 May 15;18(10):4265-4273 [CrossRef]
[21] Elgendi M. Photoplethysmography for health monitoring. Sensors (Basel) 2012 Dec 28;12(12):16347-16376 [CrossRef] [Medline]
[22] Lee S, Park C, Kim B, Lee S, Park K. Noncontact measurement of heart rate using polarized multispectral imaging. Biomed Opt Express 2021 Jan 01;12(1):511-525 [CrossRef] [Medline]
[23] Zhang Y, Guo JJ, Wang X, Shi D, Zeng K, Chen H, et al. Tuina combined with conventional rehabilitation for knee osteoarthritis: a systematic review and meta-analysis. Clin Rehabil 2021 Jan;35(1):3-16 [CrossRef] [Medline]
[24] Liu L, Guan X, Li M, Chen L, Zhang C, Wang L, et al. Tuina for chronic neck pain: a systematic review and meta-analysis of randomized controlled trials. Complement Ther Med 2015 Dec;23(6):745-755 [CrossRef] [Medline]
[25] Yeung WF, Chung KF, Poon MM, Ho FY, Chow LW, Wong VT. Acupressure, reflexology, and auricular acupressure for insomnia: a systematic review and meta-analysis. Sleep Med Rev 2012 Apr;16(2):231-241 [CrossRef] [Medline]
[26] Wang J, Tian J, Wang Y, Zhao Y, Ren J, Zhao J, et al. Effects of Tuina on the immune function of patients with rheumatoid arthritis: a randomized controlled trial. J Tradit Chin Med 2018 Oct;38(5):675-683 [CrossRef] [Medline]
[27] Li T, Li Z, Yang J, Li Y, Liu Y, Zhang L, et al. Tuina for functional constipation: a systematic review and meta-analysis. Complement Ther Med 2019 Aug;45:157-165 [CrossRef] [Medline]
[28] Chen Y, Wang Z, Shang X, Gao Y, Zhang J, Li Y, et al. Efficacy of Tuina for post-stroke shoulder pain: a systematic review and meta-analysis. Acupunct Med 2020 Jun;38(3):147-156 [CrossRef] [Medline]
[29] Guo Y, Li P, Li Y, Chen Y, Wang Z, Li Y, et al. Development and application of massage robots based on traditional Chinese medicine Tuina: a systematic review. Front Robot AI 2023 Jul 27;10:1154069 [CrossRef]
[30] Li C, Huang J, Wang L, Zhao J. Design and analysis of a novel series-parallel hybrid massage robot mechanism. J Bionic Eng 2023 Dec;20(6):1215-1229 [CrossRef]
[31] Teo J, Ng WM, Kumar D, Wang L, Lim EC. Acceptability and satisfaction of a novel massage robot for healthy volunteers. Int J Adv Robot Syst 2017 Jul 27;14(4):1-10 [CrossRef]
[32] US Food & Drug Administration. Class I and II exempt medical devices. URL: https://www.fda.gov/medical-devices/classify-your-medical-device/class-i-and-ii-exempt-medical-devices [accessed 2024-06-25]
[33] Zhao Y, Wu X, Xue X, Zhang Y, Wang H. Regulatory considerations for artificial intelligence-based medical devices. J Med Regul Res 2023;3(2):57-63 [CrossRef]
[34] Zhang RX, Lao L, Ren K, Berman BM. Mechanisms of acupuncture-electroacupuncture on persistent pain. Anesthesiology 2014 Aug;121(2):482-503 [CrossRef] [Medline]
[35] He W, Zhao L, Zhang Y, Tian X, Li Y, Chang X, et al. The acupuncture parameters-based electroacupuncture for chronic pain: an evidence-based review of clinical trials. Pain Res Manag 2020;2020:8327437 [CrossRef] [Medline]
[36] Langevin HM, Schnyer R, MacPherson H, Davis R, Harris RE, Napadow V, et al. Acupuncture for chronic pain: what we know and what we don’t know. Evid Based Complement Alternat Med 2011;2011:742943 [CrossRef] [Medline]
[37] Lu L, Zhang X, Tian C, Lu T. The biological basis of acupuncture: evidence from animal models. Front Neuroanat 2024 May 09;18:1385378 [CrossRef] [Medline]
[38] Zhou J, Zhang Y, Yang J, Li Z, Li P, Zhou K, et al. Research trends and hotspots of artificial intelligence in acupuncture: a bibliometric analysis. Acupunct Med 2023 Dec;41(6):451-461 [CrossRef] [Medline]
[39] Li P, Zhou K, Li Z, Zhou J, Li Y, Zhang Y, et al. Application of data mining techniques in clinical acupuncture practice and manipulation: a systematic review. Acupunct Med 2023 Dec;41(6):462-472 [CrossRef] [Medline]
[40] Zhang Y, Chen H, Zhang J, Qin Z, Chang X, Zhao L. Acupuncture point selection strategies for knee osteoarthritis: a systematic review of randomized controlled trials. Complement Ther Med 2021 Jun;59:102720 [CrossRef] [Medline]
[41] Cornejo-Garrido J, Vaswani A, Pérez D, Muñoz L, Rada G. Artificial intelligence to improve clinical evidence for acupuncture therapy: linking primary studies with systematic reviews in Epistemonikos database. Acupunct Med 2023 Dec;41(6):473-480 [CrossRef] [Medline]
[42] Kong J, Gollub R, Polich G, Kirsch I, Lavrov A, Vangel M, et al. A pilot study of fMRI brain activation during true and sham acupuncture needling. Pain 2000 Dec 15;89(1):5-12 [CrossRef] [Medline]
[43] Takakura N, Horiuchi T, Nakai Y, Ryu S, Yamamoto H, Kawashima K, et al. A new acupuncture needle with a force sensor for quantification of needle manipulation. Conf Proc IEEE Eng Med Biol Soc 2009;2009:6776-6779 [CrossRef] [Medline]
[44] Kim J, Lee H, Park J, Lee H, Kim J, Park H, et al. Development of an acupuncture robot with force feedback. IEEE/ASME Trans Mechatron 2010 Jun;15(3):475-482 [CrossRef]
[45] Qin Z, Rong P, Zhang X, Wang J, Park K, Huang C, et al. An acupuncture robot for precise acupoint injection with automatic depth control and safety assurance. IEEE/ASME Trans Mechatron 2016 Jun;21(3):1674-1684 [CrossRef]
[46] Li P, Zhou K, Zhang Y, Li Z, Yang J, Zhou J, et al. Computer vision technology in acupuncture manipulation recognition: a systematic review. Acupunct Med 2023 Dec;41(6):481-490 [CrossRef] [Medline]
[47] Davis RT, Osada K, Schnyer RN, Langevin HM. Novel sensor technology to quantify acupuncture needle manipulation: first-in-human safety and reliability study. J Altern Complement Med 2015 Dec;21(12):771-780 [CrossRef] [Medline]
[48] Takayama S, Watanabe M, Kobayashi T, Fuse M, Nakazawa T, Yajima H. Electrical properties of acupoints and classical acupuncture meridians: a systematic review. J Acupunct Meridian Stud 2010 Dec;3(4):193-201 [CrossRef] [Medline]
[49] Dung HC, Chen HC, Yeh CH, Chang ST, Hsieh CL. Skin impedance at acupuncture points. Am J Acupunct 2009;31(1-2):5-21 [Medline]
[50] Xie Y, Xia Y, Zhang L, Zhang X, Li C, Wang J, et al. BowNet: boundary optimized wavelet CNN for tongue contour tracking in ultrasound images. IEEE Trans Med Imaging 2024 Jun;43(6):2002-2014 [CrossRef] [Medline]
[51] Gerke S, Minssen T, Cohen IG. Ethical and legal challenges of artificial intelligence-driven healthcare. J Law Biosci 2020 Jan 01;7(1):e10115 [CrossRef] [Medline]
[52] BioRender. URL: https://biorender.com/ [accessed 2024-06-25]
Abbreviations
AI: artificial intelligence |
---|
EHR: electronic health record |
EMMA: Expert Manipulative Massage Automation |
LLM: large language model |
TCM: traditional Chinese medicine |
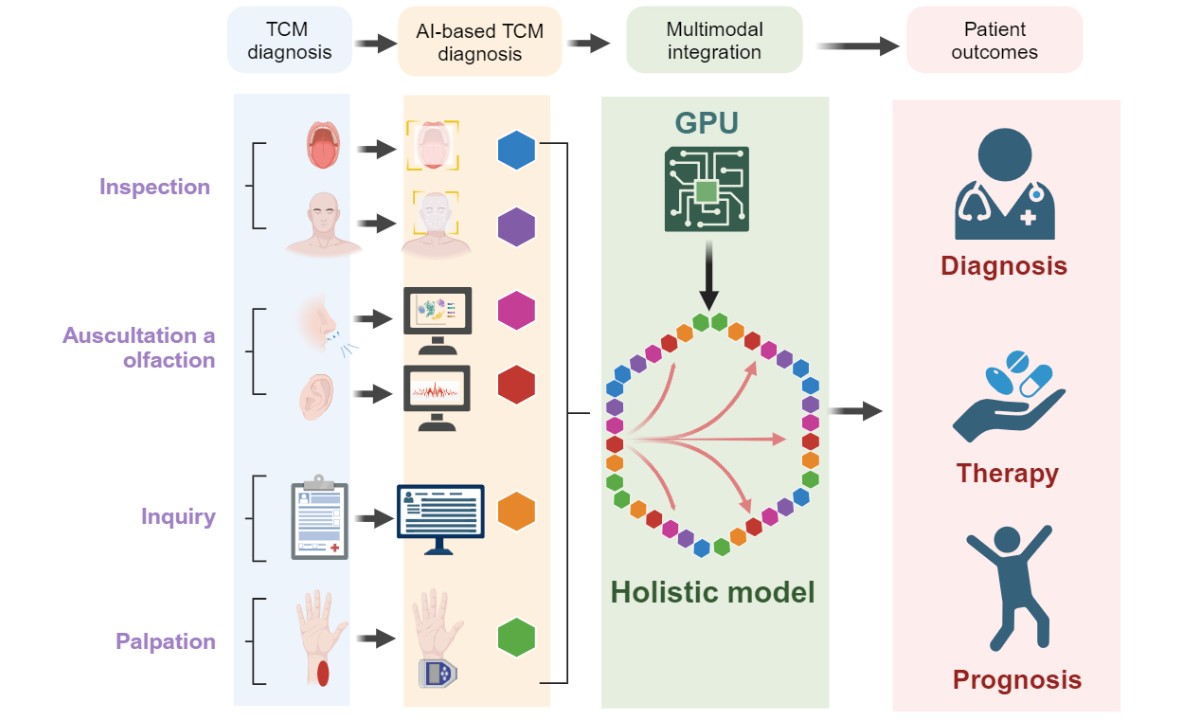
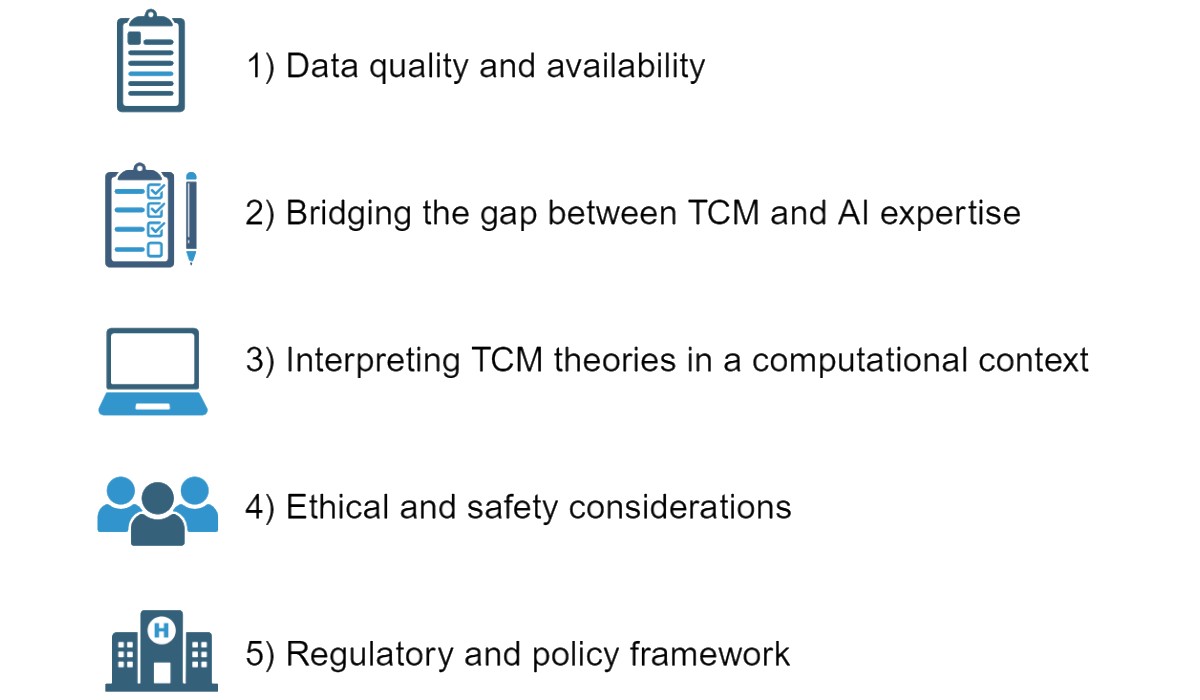
Edited by G Eysenbach, A Castonguay; submitted 18.03.24; peer-reviewed by J Sun, X Zhang; comments to author 05.04.24; revised version received 10.05.24; accepted 31.05.24; published 28.06.24.
Copyright ©Linken Lu, Tangsheng Lu, Chunyu Tian, Xiujun Zhang. Originally published in JMIR Medical Informatics (https://medinform.jmir.org), 28.06.2024.
This is an open-access article distributed under the terms of the Creative Commons Attribution License (https://creativecommons.org/licenses/by/4.0/), which permits unrestricted use, distribution, and reproduction in any medium, provided the original work, first published in JMIR Medical Informatics, is properly cited. The complete bibliographic information, a link to the original publication on https://medinform.jmir.org/, as well as this copyright and license information must be included.