1. Introduction
Chronic Obstructive Pulmonary Disease (COPD) stands as a major global health challenge, recognized as a leading cause of both morbidity and mortality. Typically, the onset of COPD symptoms occurs after the age of 40, yet the clinical presentation of this disease is remarkably diverse. This variability is evident not only at the initial diagnosis but also throughout the progression of COPD and in its overall impact on patients at different life stages. To effectively manage and treat COPD, it’s crucial to understand the myriad factors that contribute to the disease burden in each individual patient [1, 2]. Current COPD treatment guidelines are largely shaped by extensive clinical trials. However, these trials often focus on a patient demographic that represents an ‘average’ case, potentially overlooking the complex interplay of co-existing conditions that significantly influence the health trajectory of COPD patients. Furthermore, pivotal studies that inform COPD pharmacological treatments are often limited to subjects with a mean age around 65 years, plus or minus eight years [3, 4, 5, 6, 7]. This age-centric selection in research may restrict the applicability of findings to a broader patient population, especially older individuals who might need tailored treatment approaches due to their unique health profiles.
A comprehensive understanding of COPD necessitates considering that a patient’s health status is frequently shaped by other underlying conditions. These comorbidities may not always correlate directly with the severity of airflow limitation as measured by spirometry but often advance concurrently with age [8, 9]. Indeed, it’s commonly observed in COPD patients that even with similar levels of Forced Expiratory Volume in 1 second (FEV1), there can be significant variations in functional impairment, clinical manifestations, frequency of exacerbations, and quality of life. This variability may stem from the inherent heterogeneity of COPD itself, possibly originating from different disease mechanisms and manifesting in diverse clinical phenotypes. However, population-based studies have increasingly shown that as individuals with COPD age, the likelihood of developing multiple chronic comorbidities rises, contributing to a more pronounced decline in their clinical condition [10, 11].
Beyond the accumulation of comorbidities, age itself is recognized as a factor that can influence the progression of COPD. While there are parallels between lung aging (the ‘senile lung’ concept) and COPD, the normal physiological changes associated with aging should not be automatically viewed as pathological conditions requiring medical intervention. Currently, there’s limited evidence to suggest that lung aging in isolation is a primary driver for healthcare needs or hospitalizations due to COPD exacerbations. In fact, it might only account for certain associated pathological changes, notably emphysema [12]. Regardless of the specific role aging plays in COPD pathogenesis, it is undeniable that as COPD patients get older, they exhibit distinct clinical characteristics that must be recognized. These age-related differences can significantly affect the course of COPD and its management.
Recent research has increasingly emphasized the importance of incorporating time, or aging, into the complex relationship between genetics, environment, and COPD development. The age at which genetic and environmental factors interact is critical, as are an individual’s cumulative exposures and even those of their parents. Adopting a genetic–environmental–temporal perspective offers deeper insights into lung function and helps explain the varied clinical presentations of COPD [13].
Our central hypothesis is that the clinical profile of COPD is not static; it evolves with patient age. This evolution leads to variations in the overall burden of the disease. This age-related factor should be a key consideration when designing clinical trials and developing targeted treatment strategies. Recognizing the age-dependent nature of COPD can pave the way for more personalized patient care focused on addressing specific treatable traits, potentially lessening the current impact of COPD.
The advent of big data analytics and artificial intelligence (AI) in healthcare provides powerful tools to handle and extract meaningful insights from the vast and complex datasets generated by electronic health records (EHRs). This technology allows for the evaluation of crucial indicators within clinical processes, minimizing biases beyond the scope of data recording. Big data has become indispensable, revolutionizing our approach to understanding complex phenomena across various domains, particularly in epidemiology and public health. In public health, the capability to collect and analyze massive data sets has been instrumental in early disease detection, monitoring epidemiological trends, and crafting more effective preventive measures.
Within epidemiology, big data facilitates the integration of diverse data sources, including EHRs, social media data, and wearable health devices. This integration enables a more holistic view of health patterns and disease spread. Furthermore, big data analytics allows for the customization of health interventions and policies, ensuring they are tailored to the specific needs of different populations. The synergy of big data and AI further amplifies our ability to manage health challenges. AI is essential for processing and analyzing the extensive datasets derived from big data. Advanced algorithms can identify patterns and correlations in real time, enabling quicker disease detection and responses to critical epidemiological situations. Machine learning, a subset of AI, enhances predictive models by continuously refining their accuracy as new data is integrated. Thus, the combination of big data and AI is a cornerstone for strengthening epidemiological resilience and promoting global health in today’s world.
The primary objective of this study is to investigate, under real-world clinical practice conditions, how the clinical profile of COPD varies with age and how these variations affect the overall burden of the disease. By doing so, we aim to gain a more refined understanding of the interplay between genes, environment, and time in COPD, ultimately contributing to the development of higher quality treatments and prevention strategies for this pervasive disease.
2. Materials and Methods
We conducted an observational, retrospective, and non-interventional study, utilizing secondary data extracted from the unstructured text of electronic medical records (EMRs). This research was based in the Castilla-La Mancha region of Spain, where the regional healthcare service (SESCAM) employs the Savana Manager v.3.0 tool. This system allowed us to analyze data retrospectively, dating back to January 1, 2011. In our methodology, we adhered to the Strengthening the Reporting of Observational Studies in Epidemiology (STROBE) guidelines [14]. The study population comprised all patients over 40 years of age diagnosed with COPD and treated between January 1, 2011, and January 24, 2021.
The methodology employed in this study has been detailed in prior publications [15, 16, 17, 18]. Savana Manager is a data extraction system that leverages artificial intelligence (specifically, natural language processing, or NLP) and big data techniques. This technology is designed to extract unstructured clinical information—natural language or free text—from electronic health records (EHRs) and convert it into structured, reusable data for research purposes, all while maintaining patient anonymity. Through sophisticated computational linguistic techniques, the system scientifically identifies and validates comprehensive clinical content using the SNOMED CT [19] coding system, drawing data from the EHRs of the SESCAM specialized care network (including hospitalization, emergency, outpatient consultations) and primary care services.
Data Management and Protection: Hospital IT services are responsible for the initial data processing and anonymization. They ensure that Savana receives only non-identifiable data. Furthermore, during data extraction, an algorithm is used to randomly insert confounding information per patient, while recovering only a portion of individual information. This process culminates in a completely de-identified and anonymous patient database. Consequently, all study reports contain only aggregated data, ensuring that neither patients nor physicians can be identified. According to the European Data Protection Authority, once clinical records are anonymized to this extent, they fall outside the scope of the General Data Protection Regulation. This study received ethical approval from the Research Ethics Committee (Comité de Ética de la Investigación, or CEIm) of the Guadalajara public healthcare area (reference number 1/2023, dated January 17, 2023).
Information Extraction Assessment: For this study, the variable ‘COPD’ was identified within the free text using a named-entity recognition approach. To refine accuracy, layers of negation and temporality detection were applied. The negation detection model utilizes a combination of rule-based logic and a binary convolutional neural network. This network, trained on real Spanish EHRs and validated against comprehensive reference standards, classifies each clinical entity as either affirmative or non-affirmative based on its lexical and semantic context. Temporality detection is performed by an NLP module composed of several layers that work in conjunction to assign dates to clinical entities. The first layer is a named-entity detection engine that identifies any date mentions in the EHR free text. Subsequently, a relationship model based on a Bi-LSTM network determines if a detected date is associated with a detected clinical entity. A normalization layer then converts various date formats found in EHR free text into a standardized format. The final NLP processing step involves quality control operations and integrates the outputs from different NLP modules into a cohesive database.
Following cNLP processing, three authors validated the tool’s results and technology performance. This evaluation aimed to confirm the reliability of the EHRead® technology in identifying records mentioning ‘COPD’ and related variables. A set of 560 documents was manually verified to establish a gold standard for accuracy. The performance of Savana was assessed against this gold standard, measuring the accuracy in identifying records with COPD and related variables. Performance metrics included precision (P), recall (R), and the F-score, which is the harmonic mean of precision and recall.
Precision, indicating the reliability of retrieved information, was calculated as P = tp/(tp + fp). Recall, measuring the amount of information retrieved, was calculated as R = tp/(tp + fn). The F-score, an overall performance indicator, was calculated as F = 2 × precision × recall/(precision + recall). In these calculations, true positives (tp) represented correctly identified records, false negatives (fn) were unidentified records, and false positives (fp) were incorrectly retrieved records.
Previous evaluations showed that these metrics exceeded 0.9, confirming the diagnosis was sufficiently accurate for identifying the study population. The F-scores for the analyzed terms ranged from 0.92 to 0.97.
Statistical Analysis: All variables were analyzed using SPSS software (version 25.0; IBM, Armonk, NY, USA) and OpenEpi (https://www.OpenEpi.com, accessed February 6, 2023). Standard descriptive statistical analyses were employed. Qualitative variables are presented as absolute frequencies and percentages, while quantitative variables are expressed as means, 95% confidence intervals, and standard deviations. For numerical variables, the Student’s t-test for independent measures was used, and the Chi-squared test was used to assess associations and compare proportions between qualitative variables. To determine if variables were related to the selected population, significance was evaluated using a Chi-squared 2 × 2 contingency table, controlling for sex and age biases. A p-value of less than 0.05 was considered statistically significant. Savana ranks events by odds ratio (observed vs. expected frequency). In all cases, differences with a p-value less than 0.05 from the contrast test were deemed significant.
3. Results
Over the study period from January 1, 2011, to January 14, 2021, a total of 73,901 patients diagnosed with COPD received treatment from Castilla-La Mancha Public Healthcare Services (SESCAM). The average age of these patients was 73 years (95% CI: 72.9–73.1), with a significant majority, 76.8% or 56,763 individuals, being male. Figure 1 illustrates the patient inclusion process in a flowchart.
Figure 1.
Flowchart depicting the inclusion of patients in the COPD study, detailing the screening and selection process from the initial dataset to the final study population.
Table 1 provides a summary of the key clinical and demographic characteristics of the study population.
Table 1.
Baseline characteristics of the COPD study population, segmented by sex, highlighting differences in age and prevalence of various comorbidities.
Male COPD Population (n = 56,763) | Female COPD Population (n = 17,138) | p | |
---|---|---|---|
Age, years (95% CI) | 72.9 (72.8–73) | 72.3 (72.1–72.5) | |
Comorbidities | |||
Arterial hypertension (%) | 70.0 | 72.3 | |
Dyslipidemia (%) | 49.6 | 52.5 | |
Diabetes (%) | 37.9 | 38.5 | |
Smoking (%) | 41.7 | 35.9 | |
Obesity (%) | 23.9 | 32.7 | <0.001 |
Heart failure (%) | 37.3 | 48.3 | <0.001 |
Atrial fibrillation (%) | 19.4 | 18.4 | |
Ischemic cardiopathy (%) | 14.4 | 7.7 | <0.001 |
Obstructive sleep apnea (%) | 13.5 | 10.8 | |
Depression (%) | 10.0 | 27.2 | <0.001 |
Hiatal hernia (%) | 12.7 | 17.3 | <0.001 |
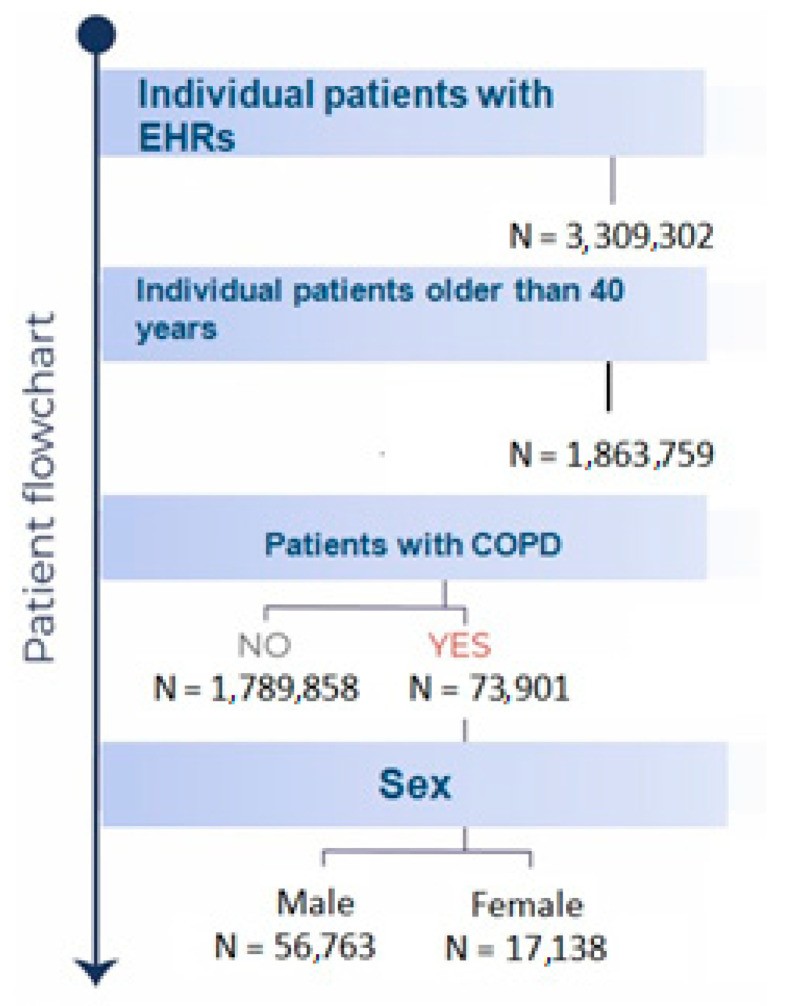
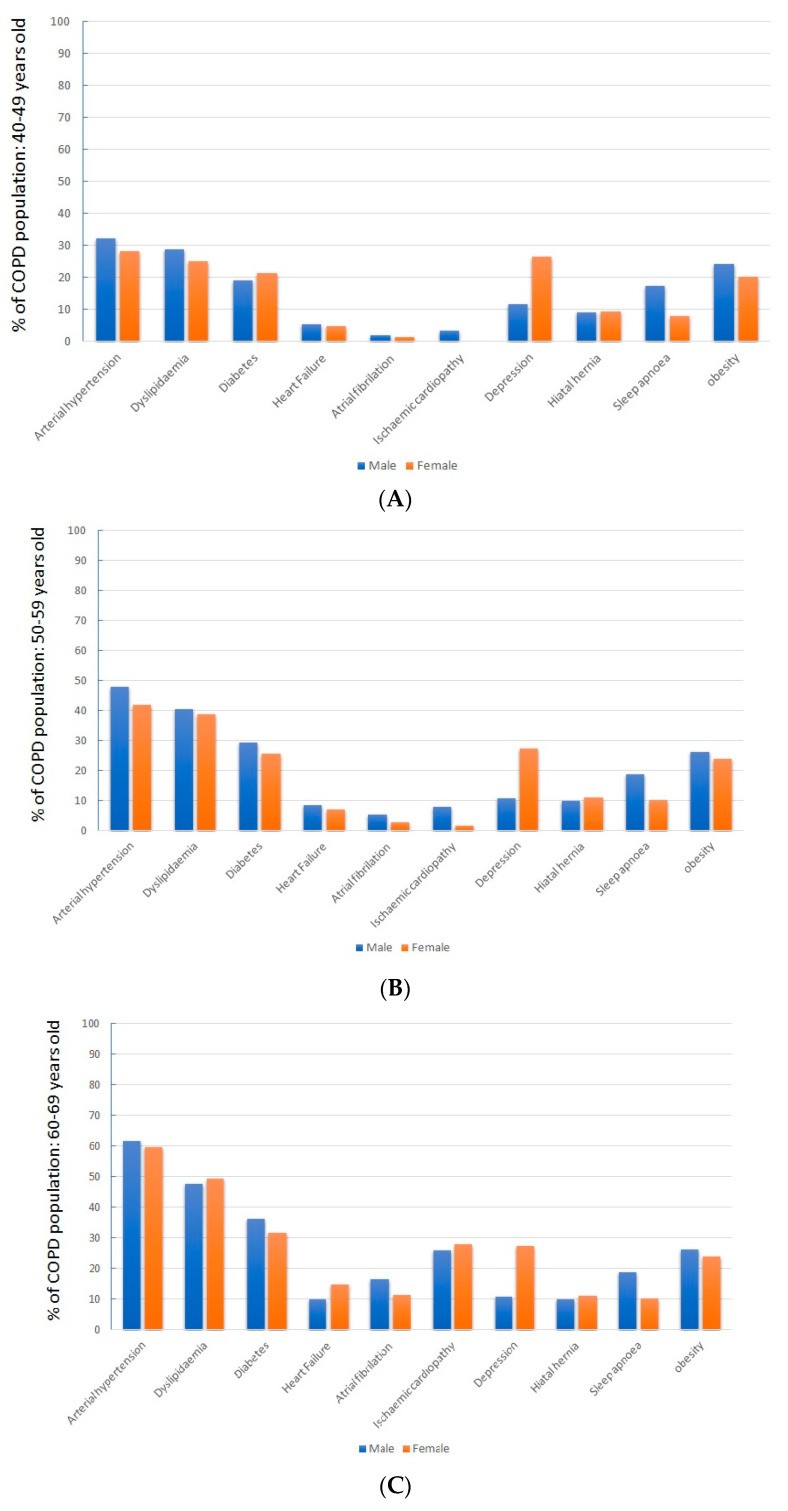
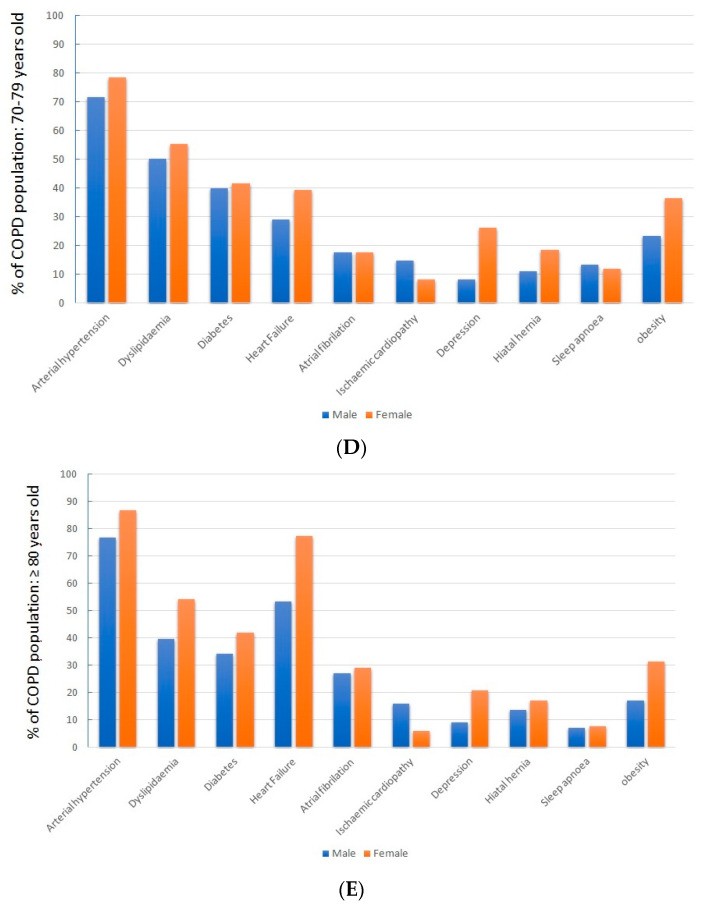
Analysis by sex revealed significant differences in comorbidity prevalence. Specifically, obesity, heart failure, depression, and hiatal hernia were significantly more prevalent in women (p < 0.05). Ischemic cardiopathy was more prevalent in men (p < 0.05).
When patient data was analyzed across different age ranges, a clear trend emerged: cardiovascular risk factors and associated diseases increased progressively with age, particularly cardiovascular diseases (Table 2).
Table 2.
Prevalence of comorbidities in COPD patients across different age ranges, compared to a control group without COPD, highlighting the age-related increase in cardiovascular conditions.
Age Range, Years Mean (CI 95%) | >40 without COPD 62.1 (62–62.1) | Total COPD Population > 40 73 (72.9–73.1) | p | COPD 40–49 45 (44.9–45.1) | COPD 50–59 54 (54.7–54.8) | COPD 60–69 64 (64.3–64.4) | COPD 70–79 74.4 (74.4–74.5) | >COPD 80 85.3 (85.3–85.4) |
---|---|---|---|---|---|---|---|---|
Sex, male (%) | 51.4 | 76.8 | 66.7 | 71.2 | 79.4 | 82.1 | 77.1 | |
Comorbidities | ||||||||
Arterial hypertension (%) | 29.9 | 70.5 | <0.001 | 30.8 | 46.2 | 61.1 | 72.7 | 78.7 |
Dyslipidemia (%) | 21.0 | 50.3 | <0.001 | 27.4 | 39.9 | 47.8 | 51.0 | 47.2 |
Diabetes (%) | 14.4 | 38.1 | <0.001 | 19.6 | 28.2 | 35.3 | 40.0 | 37.2 |
Smoking (%) | 11.2 | 40.3 | <0.001 | 70.3 | 68.2 | 52.0 | 32.1 | 17.7 |
Obesity (%) | 8.2 | 25.9 | <0.001 | 22.8 | 25.5 | 26.2 | 25.7 | 20.4 |
Heart failure (%) | 6.9 | 40.1 | <0.001 | 5.1 | 8.0 | 10.6 | 30.9 | 58.7 |
Atrial fibrillation (%) | 4.5 | 19.1 | <0.001 | 1.9 | 4.5 | 9.2 | 17.4 | 27.5 |
Ischemic cardiopathy (%) | 2.7 | 12.9 | <0.001 | 2.6 | 6.4 | 10.3 | 13.5 | 14.7 |
Obstructive sleep apnea (%) | 2.5 | 12.9 | <0.001 | 14.1 | 16.3 | 15.4 | 11.0 | 7.1 |
Depression (%) | 6.9 | 14.0 | <0.001 | 16.4 | 15.5 | 12.0 | 11.2 | 11.7 |
Hiatal hernia (%) | 5.1 | 13.8 | <0.001 | 9.1 | 10.2 | 10.8 | 12.4 | 14.3 |
Notably, heart failure prevalence was particularly high in older age groups, affecting 30.9% of patients aged 70–79 and 58.7% of those over 80. Compared to the general population aged 40 and above, COPD patients showed a significantly higher incidence of cardiovascular risk factors, cardiovascular disease, depression, and hiatal hernia.
Table 3 presents data on the burden of COPD, as measured by hospital admissions and mortality rates across different age groups.
Table 3.
The burden of COPD across age ranges, measured by hospitalization rates and in-hospital mortality, highlighting the increased impact in older populations.
Age Range, Years | 40–49 | 50–59 | 60–69 | 70–79 | >80 |
---|---|---|---|---|---|
% of total COPD population | 3.2 | 11.9 | 20.8 | 28.0 | 36.1 |
% COPD patients requiring hospitalization for deterioration | 11.9 | 14.7 | 19.9 | 24.2 | 30.7 |
Number of hospitalizations among those hospitalized | 2.3 | 2.5 | 2.9 | 3.0 | 3.0 |
In-hospital death (%) | 3.7 | 2.6 | 3.2 | 4.3 | 6.2 |
The data indicate a significant increase in both hospital admissions and mortality with advancing age. Older age groups not only constitute a larger proportion of the COPD population but also experience a greater burden in terms of healthcare utilization and mortality.
Sex-based differences in comorbidity prevalence, observed in the overall population, were consistent across all age ranges (Figure 2).
Figure 2.
Comparative analysis of comorbidity prevalence by sex across different age groups above 40 years, demonstrating consistent sex-related differences in conditions like obesity, depression, and heart failure within each age bracket.
4. Discussion
The findings of this study underscore that most COPD patients face a complex web of associated health issues, largely driven by comorbidities that accumulate with age. As COPD patients age, their clinical profiles shift, leading to increased healthcare resource utilization, particularly hospitalizations, and higher mortality rates.
It is crucial to recognize COPD not as a singular disease entity, but rather as a syndrome encompassing a range of functional and structural lung alterations that manifest as chronic respiratory symptoms. This complexity arises from the interplay of environmental and genetic factors, with time or aging acting as a significant contributor. The ultimate clinical and biological outcomes are shaped by these genetic-environmental interactions, as well as cumulative exposures experienced by both the patient and potentially previous generations. Time, or aging, emerges as a critical dimension in understanding COPD [13]. A deeper understanding of how age influences COPD could pave the way for identifying new targets for early therapeutic and preventive interventions.
Certain comorbidities, notably cardiovascular diseases like heart failure, can profoundly impact the clinical presentation of COPD. Agustí et al., using data from the ECLIPSE study, proposed a shared pathogenic mechanism linking COPD and cardiovascular diseases [20]. However, whether this relationship is causal or simply an association due to shared risk factors remains unclear. Regardless, our study robustly demonstrates that COPD patients exhibit a high prevalence of comorbidities, which significantly influence the clinical expression of COPD. Some of these comorbidities are strongly associated with increased morbidity and mortality [21]. Numerous observational studies have previously reported a higher prevalence of comorbidities in COPD patients compared to the general population, a finding corroborated in our study [8, 22]. The key contribution of our work is quantifying the magnitude of this comorbidity burden in a real-world clinical setting, minimizing selection biases inherent in many prior observational studies, and highlighting its age-adjusted significance.
Several studies have established an inverse correlation between a patient’s overall health status and the presence of comorbidities, especially when three or more conditions coexist, irrespective of lung function [23, 24, 25]. Furthermore, the number of comorbidities is linked to increased risks of exacerbations, hospitalizations, mortality, and greater economic strain on healthcare systems [26, 27, 28].
Some studies have shown that COPD patients in real-world settings have a higher incidence of obesity, depression, obstructive sleep apnea (OSA), and hiatal hernias compared to the general population [29, 30, 31]. Interestingly, the prevalence of these conditions does not necessarily intensify with patient age. However, other comorbidities, particularly cardiovascular diseases, show a marked age-related increase. Heart failure, for instance, is a common comorbidity in COPD, but its impact becomes particularly pronounced in patients over 70. In this age group, heart failure can significantly worsen the baseline clinical condition and mimic or exacerbate COPD exacerbations.
Our data, reflecting current clinical practice and derived from a real-world setting, confirms that COPD predominantly affects older individuals. This demographic group not only constitutes a larger patient population but also presents with more complex disease profiles, placing a greater demand on healthcare services. The application of big data methodologies and artificial intelligence has provided a realistic overview of the treatment needs of COPD patients within a defined region. Our findings indicate that over 50% of COPD patients in our study setting were older than 70 years. These data align with the EPISCAN2 study, which recently reported on COPD prevalence in Spain [32]. That study found that only 6.03% of COPD patients were in the 40–49 age range, while 30.08% were between 70 and 79 years old. Notably, the EPISCAN2 study did not analyze the over-80 age group, which our study found to represent a substantial 36% of the total COPD population.
A limitation of our study is that COPD diagnoses were not always confirmed by post-bronchodilator spirometry, especially in patients over 80. However, the fact that these patients are diagnosed and treated for COPD underscores the significant healthcare needs of this population. Given that most prior studies do not specifically include this older demographic, it is essential to develop targeted strategies for these age groups. Personalized treatment approaches that account for individual patient characteristics are crucial for effectively managing COPD in elderly patients.
Multimorbidity and comorbidity are aspects of clinical complexity, but they do not fully encapsulate a patient’s overall health status. Some patients with a single disease may require highly complex management, while others with multiple conditions might be relatively straightforward to manage. Therefore, comorbidity assessments alone cannot replace comprehensive functional evaluations for diagnosis, prognosis, or treatment planning, particularly in older populations where the impact of individual diseases may be overshadowed by the cumulative effect of multiple age-related physiological changes. Despite these considerations, the use of a big data approach is a significant strength of this study. We believe this approach can overcome the historical exclusion of elderly patients from studies on chronic diseases and multimorbidity, despite this group being the most prevalent and accounting for a significant portion of healthcare expenditures. They represent the true patient base of our healthcare systems [33, 34].
5. Conclusions
The primary conclusion of this study, strengthened by its large-scale, real-world setting, is that COPD is predominantly a disease affecting older adults who frequently present with comorbidities that significantly influence the disease’s progression. Effective management of COPD necessitates a holistic understanding of the patient, not just the disease itself. Utilizing frailty assessment tools developed by geriatric specialists can provide a more nuanced understanding of older COPD patients, enabling the application of tailored interventions to meet their specific needs. The oldest age groups bear the greatest burden of COPD, impacting both individual patients and public healthcare systems. Further research into the role of time and aging in COPD, as suggested by the GETomicis concept [13], could lead to the identification of novel early therapeutic and preventive targets, complementing our understanding of genetic and environmental risk factors.
Author Contributions
D.M., J.L.I., J.R., and J.M.R. were responsible for the study design, development, data extraction, analysis, and manuscript preparation. J.R., J.C., M.B., and A.P. contributed to data extraction, analysis, and manuscript revision. All authors have reviewed and approved the final manuscript.
Institutional Review Board Statement
This study was conducted in accordance with the Declaration of Helsinki and approved by the Ethics Committee of Guadalajara’s Hospital for studies involving humans (Ref: 1/2023, date 17 January 2023).
Informed Consent Statement
Informed consent was waived as the study was anonymous, observational, and retrospective, using de-identified data.
Data Availability Statement
Data are available upon request from the corresponding author, subject to data sharing agreements with the National Health System of Castilla La Mancha. Data are not publicly available due to privacy restrictions.
Conflicts of Interest
The authors declare no conflicts of interest, financial compensations, or links to tobacco manufacturers or the tobacco industry.
Funding Statement
This research was supported by the Chair of Inflammatory Diseases of the Airways, University of Alcalá.
Footnotes
Disclaimer/Publisher’s Note: The opinions expressed in this article are those of the authors and do not necessarily reflect the views of MDPI or the journal editors. MDPI and the editors are not responsible for any consequences arising from the content of this publication.
References
[List of references as in the original article]
Associated Data
Data Availability Statement
The data presented in this study are available upon request from the corresponding author. The data are not publicly available because they belong to the National Health System of Castilla La Mancha.