Introduction
Accurate and timely diagnosis is the cornerstone of effective healthcare, profoundly influencing patient management and outcomes. In the complex landscape of modern medicine, clinicians are increasingly challenged by intricate case presentations and the sheer volume of rapidly evolving medical knowledge. This is particularly evident in specialties like General Internal Medicine (GIM), where diagnostic dilemmas are common, necessitating robust support systems. Studies reveal that diagnostic error rates in GIM settings can be significant, highlighting a critical need for innovative tools to assist physicians in achieving greater diagnostic precision. The pursuit of enhanced diagnostic support has led to the exploration of Clinical Decision Support (CDS) systems, and more recently, the transformative potential of AI in differential diagnosis.
Traditional CDS tools, including symptom checkers and differential diagnosis generators, have been around for decades. While symptom checkers are often geared towards public use, differential diagnosis generators are designed to aid healthcare professionals. The concept of computer-aided healthcare dates back to the early 1970s, driven by the ambition to leverage computational power for improved patient care. Early CDS tools often relied on complex algorithms, probability assessments, and heuristic methods. Some have found utility in outpatient GIM settings. However, despite their promise, these systems often add to clinician workload, mainly due to the requirement for structured data input, hindering widespread adoption.
Enter artificial intelligence (AI), and specifically, large language models, offering a paradigm shift in healthcare support, particularly through AI-driven differential diagnosis chatbots. Models like ChatGPT, developed by OpenAI, represent a significant leap in natural language processing and generative AI. Built upon generative pre-trained transformer (GPT) architecture, ChatGPT can generate human-like responses, understand complex queries, and process vast amounts of textual data. With each iteration, from the third-generation GPT (GPT-3.5) to the more advanced fourth-generation GPT (GPT-4), these models have demonstrated improved accuracy across various professional benchmarks and problem-solving tasks. While acknowledging the inherent limitations and potential risks associated with AI chatbots, including transparency issues, reliance on non-specialized or outdated medical knowledge, biases, and the risk of misinformation, the continuous advancements in AI systems like ChatGPT signal a promising trajectory towards achieving diagnostic excellence.
Recognizing the potential clinical applications of AI in differential diagnosis, it is crucial to rigorously evaluate their diagnostic accuracy, especially for complex cases frequently encountered in specialized departments like GIM. Generative AI, such as ChatGPT, has the potential to mitigate diagnostic errors stemming from the inherent complexity of GIM cases. This could lead to streamlined workflows within these departments, ultimately enhancing patient care and improving clinical outcomes. This investigation aims to shed light on the utility of generative AI, particularly ChatGPT, as a CDS tool, focusing on its application in the GIM setting.
Previous studies have explored the diagnostic accuracy of ChatGPT in generating differential diagnosis lists for clinical vignettes, reporting accuracies ranging from 64% to 83%. However, these studies often lacked a specific focus on cases originating from GIM departments, known for their diagnostic challenges. This research addresses this gap by evaluating the diagnostic accuracy of ChatGPT, specifically using clinical vignettes extracted from case reports published by a GIM department. By focusing on these challenging GIM case reports, this study provides a more stringent assessment of ChatGPT’s diagnostic capabilities compared to prior investigations. We anticipate that ChatGPT-4 will demonstrate a level of accuracy in generating correct diagnoses within its differential diagnosis lists that is consistent with or surpasses the previously reported range of 64%-83%. This study will delve into the efficacy of AI in differential diagnosis, paving the way for its responsible integration into clinical practice.
Methods
Study Design
This study meticulously evaluated the diagnostic accuracy of differential diagnosis lists generated by both ChatGPT-3.5 and ChatGPT-4. The evaluation was based on clinical vignettes derived from case reports published by the Department of General Internal Medicine (GIM), also known as the Department of Diagnostic and Generalist Medicine, at Dokkyo Medical University Hospital in Shimotsuga, Tochigi, Japan. The term “differential diagnosis,” central to this study, refers to a ranked list of possible medical conditions that could explain a patient’s presenting symptoms and signs. This list is formulated through a careful consideration of the patient’s medical history, physical examination findings, and the results of relevant investigations, serving as a critical step in the diagnostic process.
Ethical Considerations
As this research utilized anonymized clinical vignettes sourced from publicly available, published case reports, it was determined to be exempt from the requirement for ethics committee approval and individual patient consent. The use of de-identified, published data ensures patient privacy and aligns with ethical research standards for retrospective studies.
Clinical Vignettes: Real-World Case Scenarios for AI Diagnosis
The clinical vignettes used in this study were carefully curated from case reports published by the GIM Department of Dokkyo Medical University Hospital. Cases referred to this department are often diagnostically challenging, representing a high degree of clinical complexity. A subset of these particularly interesting and complex cases are documented and published as case reports in peer-reviewed medical journals. To identify relevant case reports published in English by the department, a comprehensive PubMed search was conducted on March 20, 2023. The search query employed the following keywords: “(Dokkyo Medical University [affil]) AND (Generalist Medicine [affil]) AND (2016/4/1:2022/12/31 [dp]) AND (Case Reports [PT]).”
The initial search yielded 54 case reports on PubMed. Two experienced GIM physicians (TH and RK) then meticulously reviewed these reports to select cases that were diagnostically relevant and to ascertain the final diagnosis for each. They subsequently transformed these selected cases into clinical vignettes. Two cases were excluded as they were deemed non-diagnostic, resulting in a final set of 52 cases for inclusion in the study.
For example, the case report titled “Hepatic portal venous gas after diving,” identified as case number 3 in Supplementary Tables S1 and S2, provided the source material for one vignette. From the case description section of this report, the following clinical vignette was extracted: “A 68-year-old man with diabetes and…There was no evidence of pneumatosis intestinalis.” The established final diagnosis for this case was decompression sickness.
These case reports, having undergone rigorous peer review for journal publication, represent a high standard of clinical documentation. Experienced GIM physicians were responsible for their writing and selection. Each clinical vignette encompassed pertinent clinical history, physical examination findings, and investigation results. To maintain focus on diagnostic reasoning, elements such as the original title, abstract, introduction, clinical assessment, original differential diagnosis, final diagnosis as stated in the report, figures, figure legends, tables, and the broader context of the case reports were intentionally removed from the vignettes. The final diagnosis for each case, derived through standard clinical diagnostic processes and validated by publication in these reports, was independently confirmed by the two experienced GIM physicians involved in vignette selection. Any discrepancies between the physicians regarding the final diagnosis were resolved through detailed discussion and consensus. The publication date and open access status of each included case report were also recorded for subsequent analysis.
Differential Diagnosis Lists: Human vs. AI
To establish a human benchmark for comparison, differential diagnosis lists for each clinical vignette were independently generated by three GIM physicians (KT, YK, and T Suzuki) external to Dokkyo Medical University. Each physician was assigned a subset of the vignettes, averaging approximately 17 cases per physician. They were instructed to create their top 10 differential diagnosis lists in English, based solely on the information provided in the clinical vignettes. Crucially, they were prohibited from consulting with other physicians or utilizing any CDS tools during this process. The physicians relied exclusively on their clinical expertise and experience to generate these lists. Prior to commencing the task, it was confirmed that these physicians were unaware of the original case reports, the clinical vignettes themselves, the final diagnoses, and the differential diagnosis lists generated by ChatGPT-3.5 and ChatGPT-4. Furthermore, the physicians remained blinded to each other’s assessments throughout the study. A computer-generated randomization sequence determined the order in which the clinical vignettes were presented to each physician to minimize potential order effects.
For the AI component, ChatGPT-3.5 (March 14 version; OpenAI, LLC) was accessed on March 20, 2023, and ChatGPT-4 (March 23 version; OpenAI, LLC) was accessed on April 10, 2023. It is important to note that neither ChatGPT model had undergone specific training or reinforcement for medical diagnostic tasks. To elicit differential diagnoses from the AI models, a physician (TH) used the following prompt: “Tell me the top 10 suspected illnesses for the following symptoms: (copy and paste each clinical vignette).” This prompt was selected based on preliminary testing of various prompts, where it consistently yielded comprehensive and relevant lists of potential illnesses. Similar to the physician evaluation, the order of vignette presentation to ChatGPT-3.5 and ChatGPT-4 was randomized using a computer-generated sequence. To prevent any influence from previous interactions, the conversation history was cleared before each new clinical vignette was input. The initial responses generated by each ChatGPT model were taken as their respective top 10 differential diagnosis lists.
Evaluation Metric: Assessing Diagnostic Accuracy
The evaluation of both human-generated and AI-generated differential diagnosis lists was conducted by two additional GIM physicians (YH and KM). These evaluators, blinded to the origin of each list (physician-generated vs. AI-generated), assessed whether the established final diagnosis for each case was present within the provided differential diagnosis lists. A binary scoring system was employed. A diagnosis was scored as “1” if it accurately and specifically identified the correct condition or was sufficiently close to the definitive diagnosis to enable prompt and appropriate clinical management. Conversely, a diagnosis received a score of “0” if it significantly deviated from the actual diagnosis, indicating a clinically meaningful error. This scoring approach is consistent with established methods for evaluating diagnostic accuracy in clinical settings. In cases of disagreement between the two evaluators, discrepancies were resolved through detailed discussion and consensus agreement to ensure objective and reliable evaluation. When the final diagnosis was identified within a list, its rank within that list was also recorded for further analysis.
Statistical Measurements and Exploratory Analysis
The primary outcome measures were the rates of correct diagnoses achieved by ChatGPT-3.5, ChatGPT-4, and the physician group. These rates were calculated for the top 10 differential diagnosis lists, the top 5 differential diagnosis lists, and the top-ranked diagnosis in each list. For each category (top 10, top 5, top diagnosis), the presence or absence of the final diagnosis was treated as a binary variable (present=1, absent=0).
In addition to the primary analysis, exploratory analyses were conducted to investigate potential factors influencing AI diagnostic performance. First, the study examined whether the open access status of the original case reports affected diagnostic accuracy. Given that GPT-3.5 and GPT-4 are trained on publicly accessible internet data, it was hypothesized that open access materials might be better represented in their training data, potentially leading to improved performance on vignettes derived from these sources. Second, the study explored the impact of publication date. ChatGPT-3.5 and ChatGPT-4 have knowledge cutoffs in early 2021. Therefore, it was hypothesized that the models might exhibit higher diagnostic accuracy for case reports published prior to this cutoff date compared to more recent publications (e.g., 2022).
Categorical and binary variables are presented as counts and percentages. Comparisons between groups were performed using the chi-square test. To address the increased risk of type I error associated with multiple comparisons, the Bonferroni correction was applied, a conservative method chosen for its strict control over false positives. The Bonferroni-corrected significance level was set at a P value of <.0167 (0.05 divided by 3 comparisons per each set of comparisons – top 10, top 5, and top diagnosis).
Results
Case Report Characteristics
The study included a total of 52 case reports. Of these, 39 (75%) were published as open access articles. Regarding publication timing, 24 (46%) case reports were published before 2021, while 12 (23%) were published in 2021, and 16 (31%) were published in 2022. A comprehensive list of the included case reports is provided in Multimedia Appendix 1.
Diagnostic Accuracy of AI and Physicians
Representative examples illustrating the differential diagnosis lists generated by ChatGPT-3.5, ChatGPT-4, and physicians, along with the corresponding final diagnoses, are presented in Table 1.
The diagnostic performance metrics for ChatGPT-4, ChatGPT-3.5, and physicians are summarized in Table 2. ChatGPT-4 achieved correct diagnoses in 83% (43/52) of cases within its top 10 differential diagnosis lists, 81% (42/52) within its top 5 lists, and 60% (31/52) as its top-ranked diagnosis. ChatGPT-3.5 demonstrated slightly lower accuracy, with correct diagnoses in 73% (38/52) of cases within the top 10 lists, 65% (34/52) within the top 5, and 42% (22/52) as the top diagnosis.
Statistical comparisons revealed that the diagnostic accuracy of ChatGPT-4 was comparable to that of physicians across all measured metrics. There were no statistically significant differences between ChatGPT-4 and physicians in the rates of correct diagnoses within the top 10 differential diagnosis lists (83% vs 75%, P=.47), top 5 lists (81% vs 67%, P=.18), or as the top diagnosis (60% vs 50%, P=.43). Similarly, while ChatGPT-4 showed numerically higher accuracy than ChatGPT-3.5, these differences were not statistically significant for the top 10 lists (83% vs 73%, P=.34), top 5 lists (81% vs 65%, P=.12), or top diagnosis (60% vs 42%, P=.12). Multimedia Appendix 2 provides a detailed compilation of the final diagnoses, along with the complete top 10 differential diagnosis lists generated by ChatGPT-3.5, ChatGPT-4, and the physician group for all cases.
Exploratory Analysis: Open Access and Publication Date
The exploratory analysis investigating the impact of open access status and publication date on AI diagnostic performance is presented in Table 3. No statistically significant differences were observed in the rates of correct diagnoses for either ChatGPT-3.5 or ChatGPT-4 when comparing open access case reports to non-open access reports, or when comparing case reports published before 2021 to those published in 2022. This lack of statistically significant difference suggests that, within this dataset, open access status and publication date (pre- vs post- knowledge cutoff) did not substantially influence the diagnostic accuracy of the ChatGPT models. However, the analysis is limited by sample size, particularly after stratification.
Table 1. Representative Examples of Differential Diagnosis Lists with Final Diagnoses
Final Diagnosis | Differential Diagnosis Lists |
---|---|
ChatGPT-3.5 | |
Tarsal Tunnel Syndrome | – Peripheral neuropathy – Tarsal tunnel syndrome – Lumbar spinal stenosis – Diabetes – Alcohol abuse – Vitamin B12 deficiency – Hypothyroidism – Chronic kidney disease – Multiple sclerosis – Charcot-Marie-Tooth disease |
Nonepisodic Angioedema Associated with Eosinophilia | – Eosinophilic fasciitis – Acute interstitial nephritis – Hypereosinophilic syndrome – Churg-Strauss syndrome – Idiopathic hypereosinophilia syndrome – Lymphoma – Systemic lupus erythematosus – Sarcoidosis – Infectious mononucleosis – Allergic reactions |
Decompression Sickness | – Arterial gas embolism – Decompression sickness – Carbon monoxide poisoning – Nitrogen narcosis – Hypothermia – Acute pancreatitis – Mesenteric ischemia – Gastroenteritis – Gastric ulcer – Acute cholecystitis |
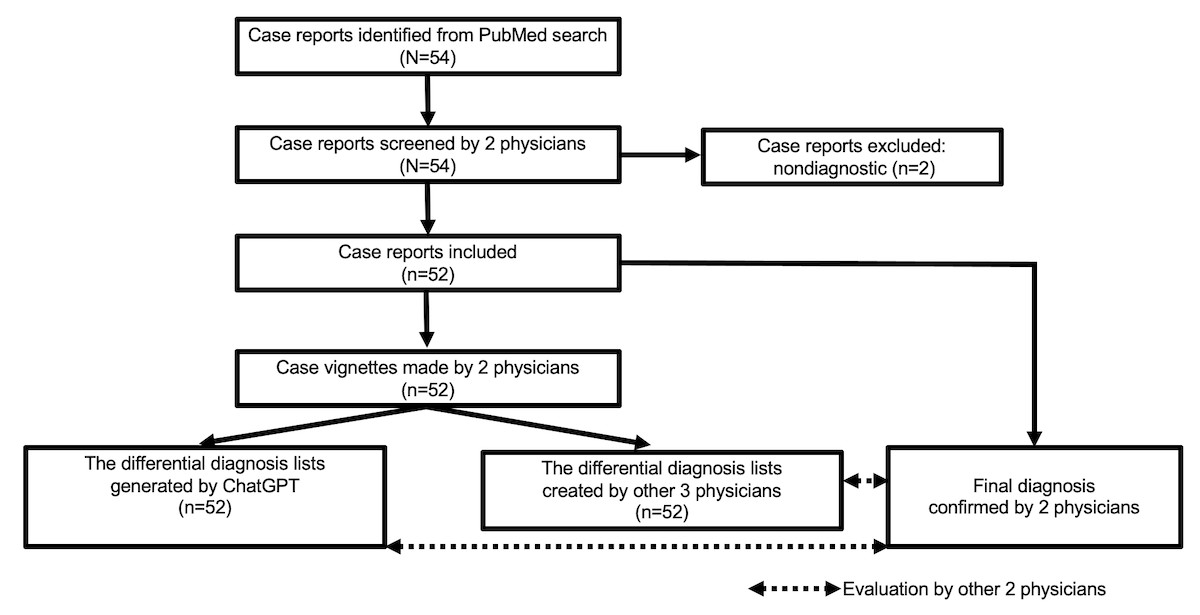
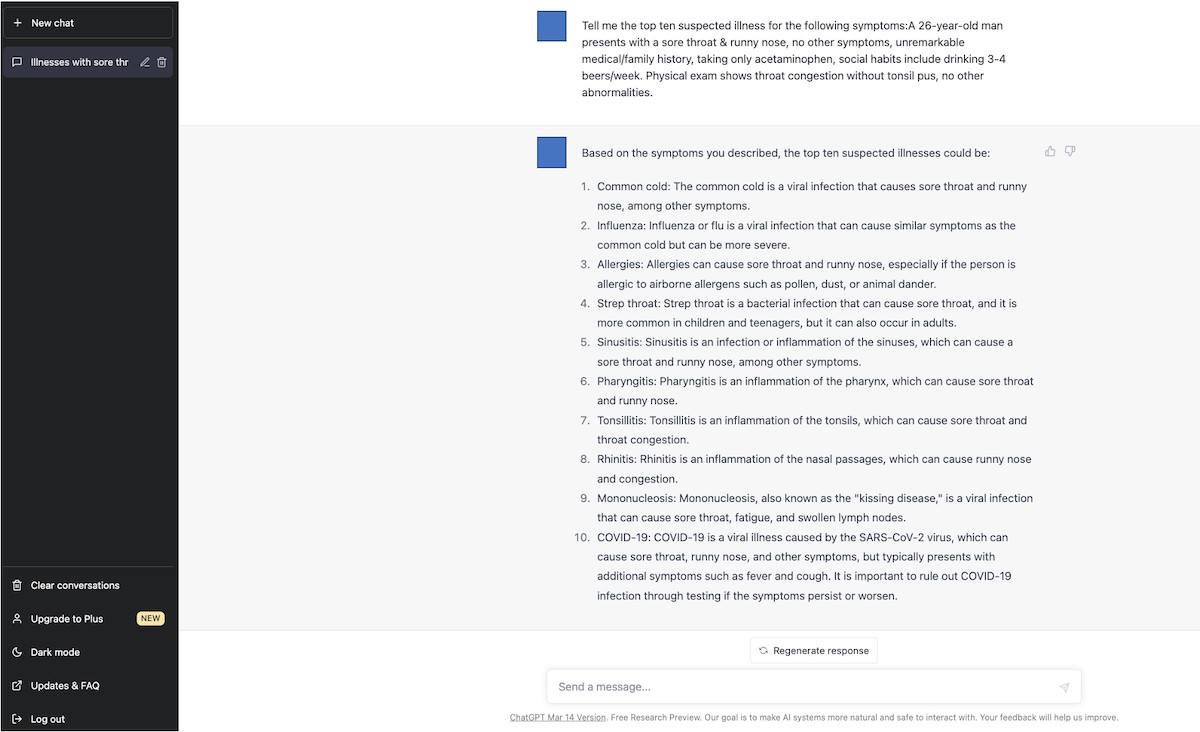
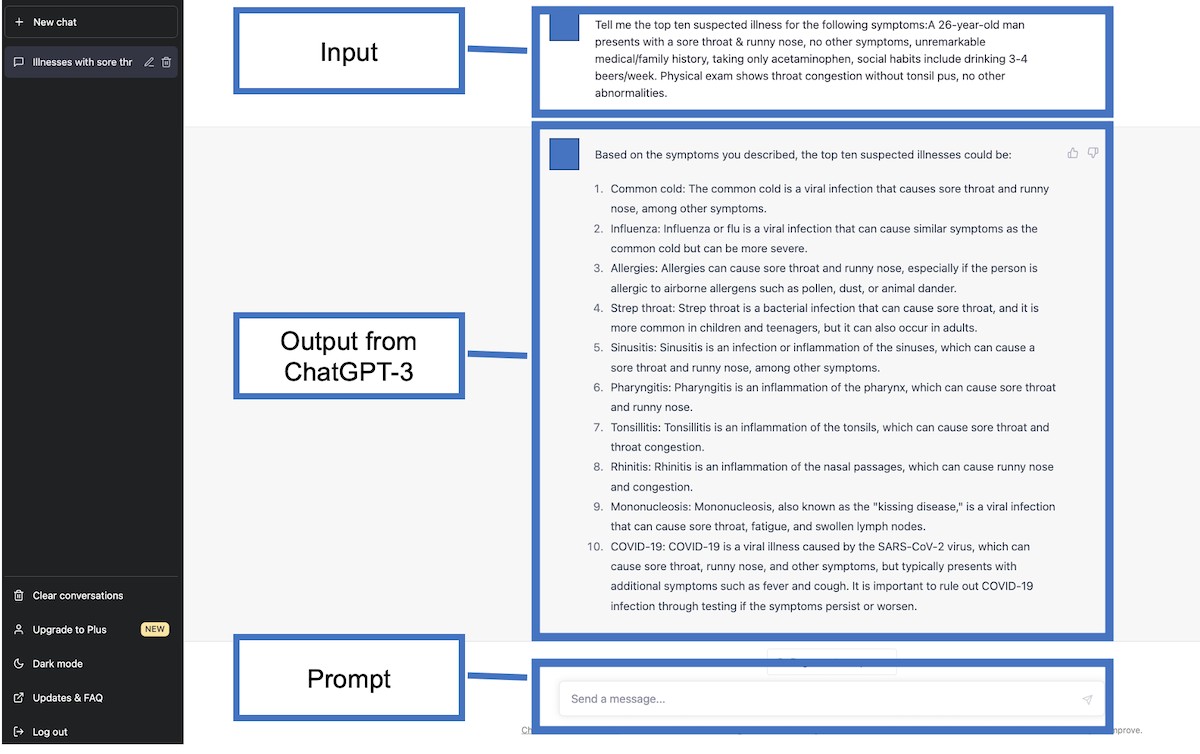
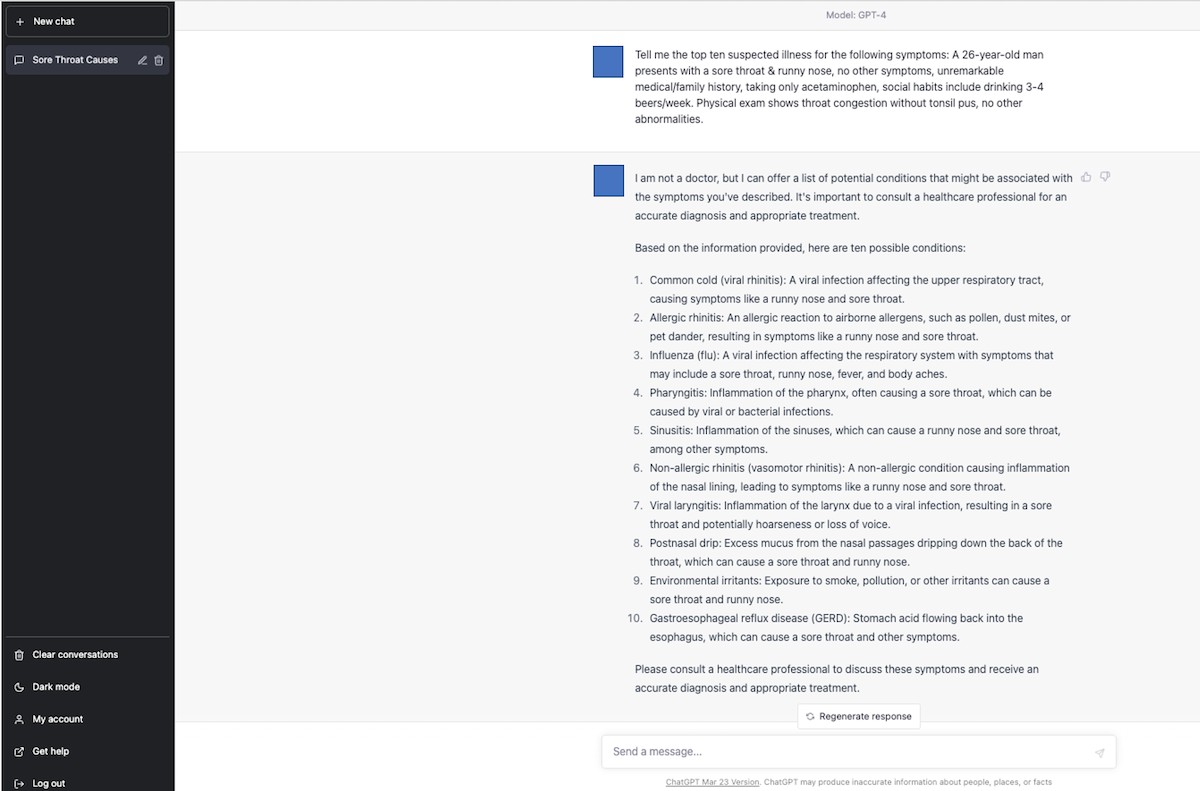
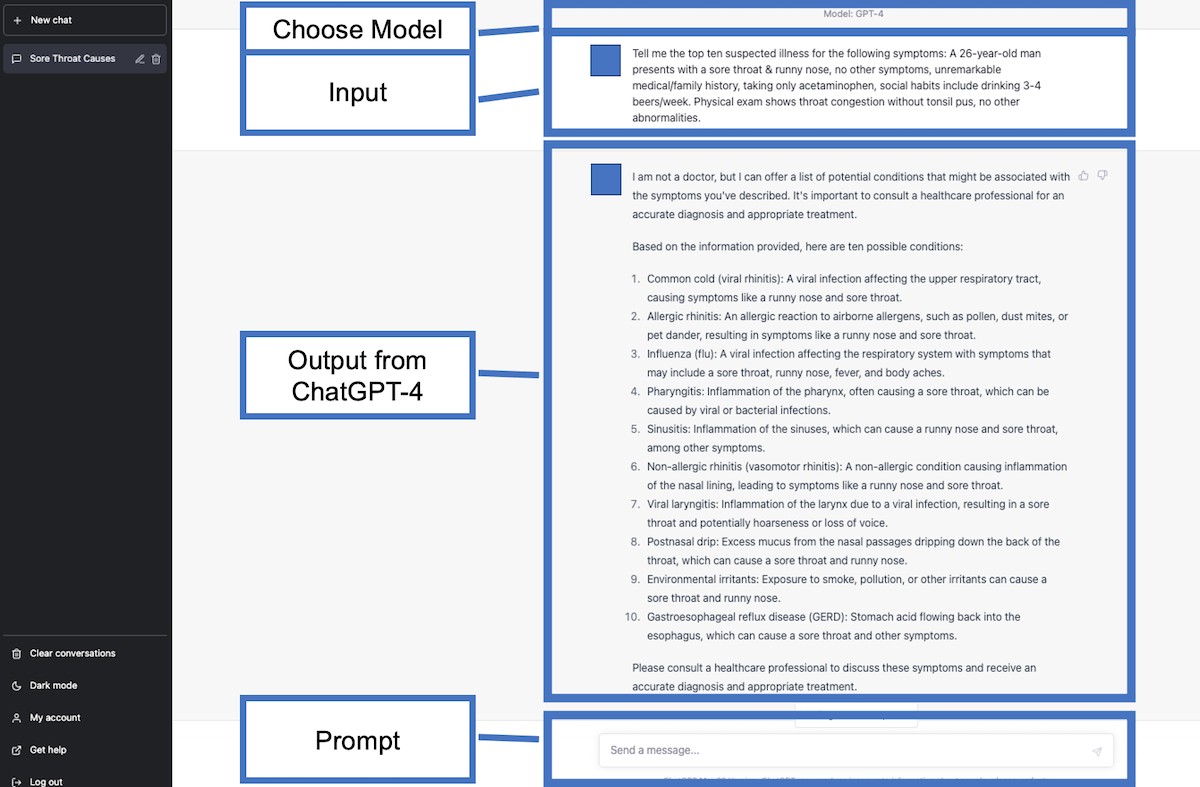
Table 2. Diagnostic Accuracy Comparison: ChatGPT-4, ChatGPT-3.5, and Physicians
Variable | ChatGPT-4 (n=52), n (%) | ChatGPT-3.5 (n=52), n (%) | Physicians (n=52), n (%) | P value |
---|---|---|---|---|
ChatGPT-4 vs Physicians | ChatGPT-3.5 vs Physicians | ChatGPT-4 vs ChatGPT-3.5 | ||
Within Top 10 | 43 (83) | 38 (73) | 39 (75) | .47 |
Within Top 5 | 42 (81) | 34 (65) | 35 (67) | .18 |
Top Diagnosis | 31 (60) | 22 (42) | 26 (50) | .43 |
Table 3. Exploratory Analysis: Impact of Open Access and Publication Date on AI Diagnostic Accuracy
Variable | ChatGPT-4 | ChatGPT-3.5 |
---|---|---|
Open Access (n=39), n (%) | Non–Open Access (n=13), n (%) | |
Within Top 10 | 32 (82) | 11 (85) |
Within Top 5 | 31 (80) | 11 (85) |
Top Diagnosis | 22 (56) | 9 (69) |
Discussion
Key Findings and Implications for AI in Differential Diagnosis
This study presents several significant findings regarding the application of AI in differential diagnosis. Firstly, it robustly demonstrates the considerable diagnostic accuracy of differential diagnosis lists generated by both ChatGPT-3.5 and ChatGPT-4 when presented with complex clinical vignettes derived from real-world case reports. Notably, ChatGPT-4 achieved a correct diagnosis rate exceeding 80% within both its top 10 and top 5 differential diagnosis lists. This level of accuracy suggests that ChatGPT-4 holds substantial promise as a supplementary diagnostic tool for physicians, particularly when confronted with diagnostically challenging cases. The study’s results indicate that GPT-4’s diagnostic capabilities are not only significant but also comparable to those of experienced physicians, suggesting its potential to function as a form of “collective intelligence” capable of cross-validating clinical diagnoses made by human practitioners.
Secondly, the exploratory analysis revealed no statistically significant impact of either the open access status or the publication date of the original case reports on the diagnostic accuracy of ChatGPT-3.5 and ChatGPT-4. While it was hypothesized that open access status and recency (prior to the models’ knowledge cutoff) might positively influence performance, the absence of such an effect suggests that the models’ knowledge base is sufficiently broad and robust to perform consistently across these variables, at least within this dataset. However, the limited sample size in the stratified analysis warrants cautious interpretation of these null findings.
Clinical and Educational Applications of AI-Driven Differential Diagnosis
The successful integration of generative AI, such as ChatGPT, into clinical practice has the potential to revolutionize patient care and optimize physician workflows. Given ChatGPT-4’s demonstrated pretraining diagnostic accuracy exceeding 80%, clinicians could gain immediate and valuable support in navigating complex and ambiguous cases. This could lead to a reduction in diagnostic errors, ultimately enhancing patient safety and improving clinical outcomes. Furthermore, by augmenting diagnostic processes, AI in differential diagnosis tools could free up clinicians’ time, allowing them to dedicate more attention to the nuanced and humanistic aspects of patient care, potentially boosting overall healthcare efficiency and job satisfaction.
In medical education, AI in differential diagnosis tools like ChatGPT can play a transformative role in training future physicians. Engaging with these AI systems can expose medical learners to a wider range of potential diagnoses, sharpening their clinical reasoning skills and expanding their medical knowledge base. This exposure can better prepare them to confidently and effectively manage complex clinical scenarios they will encounter in their practice. ChatGPT could serve as a valuable educational resource, facilitating interactive learning and providing immediate feedback on diagnostic reasoning.
Study Limitations and Future Directions for AI in Medical Diagnosis
Despite the compelling findings, it is essential to acknowledge the limitations of this study. Firstly, the study’s case materials were exclusively derived from complex case reports published by a single GIM department at a single medical center. While these cases are representative of the diagnostic challenges encountered in GIM, they may not fully encompass the breadth of clinical presentations across all medical specialties or even within GIM departments at different institutions. The cases were also not randomly selected but rather chosen for their complexity and educational value, potentially introducing selection bias. Therefore, the generalizability of these findings to other clinical settings and more routine clinical presentations may be limited. Future research should aim to validate these findings using more diverse and representative datasets.
Secondly, the differential diagnosis lists generated by physicians represent the expertise of a specific group of GIM physicians. The diagnostic accuracy of physicians can vary based on specialty, training level, and individual experience. Future studies should incorporate a broader range of physician participants, including those from different specialties and with varying levels of experience, to provide a more comprehensive benchmark for comparison.
Thirdly, while the exploratory analysis on open access and publication date did not reveal significant effects, the possibility of indirect learning or familiarity with these cases by both AI models and physicians cannot be entirely excluded. Although the physicians generating differential diagnoses were blinded to the case reports, and the AI models are assumed to have broad internet-based training, subtle biases related to publicly available medical knowledge cannot be definitively ruled out.
Finally, a potential time lag existed between the generation of differential diagnosis lists by ChatGPT-3.5 and ChatGPT-4. While efforts were made to minimize temporal bias, the rapidly evolving nature of AI models warrants consideration of this factor in interpreting the comparative performance.
Addressing these limitations, future research should focus on evaluating the diagnostic accuracy of ChatGPT models using meticulously curated case materials that are demonstrably outside of the models’ training data. Furthermore, future investigations should delve into the “literacy level” of AI models in the medical domain, assessing their comprehension of nuanced medical texts and their alignment with established medical knowledge. Direct comparative studies between ChatGPT and other existing CDS systems are also warranted to better understand the relative strengths and weaknesses of different AI in differential diagnosis approaches.
The Broader Impact of AI in Healthcare and the Future of Differential Diagnosis
As AI systems become increasingly integrated into healthcare, their influence is expected to extend far beyond diagnostic support. Generative AIs have the potential to reshape the dynamics of patient-physician interactions, fostering more informed and collaborative relationships. They can also play a vital role in democratizing access to medical knowledge, potentially extending expert-level medical advice to underserved populations in remote areas. Given these profound implications, ongoing and rigorous investigation into the multifaceted ramifications of AI in differential diagnosis and its broader integration into healthcare is not just beneficial, but essential for ensuring responsible and equitable implementation. The future of medical practice will likely be significantly shaped by the continued evolution and refinement of AI in differential diagnosis tools, promising a new era of enhanced accuracy, efficiency, and accessibility in healthcare.
Authors’ Contributions
TH, RK, YH, KM, KT, YK, T Suzuki, and T Shimizu contributed to the study concept and design. TH performed the statistical analyses. TH contributed to the drafting of the manuscript. RK, YH, KM, KT, YK, T Suzuki, and T Shimizu contributed to the critical revision of the manuscript for relevant intellectual content. All authors have read and approved the final version of the manuscript. We would like to specially thank Dr. Kenjiro Kakimoto, Department of Psychiatry, Nihon University School of Medicine, for his assistance with the analysis. This study was conducted using resources from the Department of Diagnostics and Generalist Medicine at Dokkyo Medical University.
Conflicts of Interest
None declared.
Multimedia Appendix 1. Case reports included in this study.
[PDF File (Adobe PDF File), 52 KB]
Multimedia Appendix 2. Final diagnosis and the differential diagnosis lists generated by ChatGPT and those created by physicians.
[PDF File (Adobe PDF File), 203 KB]
References
[1] {{ original reference 1 }}
[2] {{ original reference 2 }}
[3] {{ original reference 3 }}
[4] {{ original reference 4 }}
[5] {{ original reference 5 }}
[6] {{ original reference 6 }}
[7] {{ original reference 7 }}
[8] {{ original reference 8 }}
[9] {{ original reference 9 }}
[10] {{ original reference 10 }}
[11] {{ original reference 11 }}
[12] {{ original reference 12 }}
[13] {{ original reference 13 }}
[14] {{ original reference 14 }}
[15] {{ original reference 15 }}
[16] {{ original reference 16 }}
[17] {{ original reference 17 }}
[18] {{ original reference 18 }}
[19] {{ original reference 19 }}
[20] {{ original reference 20 }}
[21] {{ original reference 21 }}
[22] {{ original reference 22 }}
[23] {{ original reference 23 }}
[24] {{ original reference 24 }}
[25] {{ original reference 25 }}
[26] {{ original reference 26 }}
[27] {{ original reference 27 }}
Abbreviations
AI: | Artificial Intelligence |
---|---|
CDS: | Clinical Decision Support |
GIM: | General Internal Medicine |
GPT: | Generative Pretrained Transformer |
GPT-3.5: | Third-generation Generative Pretrained Transformer |
GPT-4: | Fourth-generation Generative Pretrained Transformer |