1. Introduction
Artificial Intelligence (AI) is rapidly transforming various fields, and medical diagnosis is no exception. From identifying tumors in medical images to detecting subtle signs of disease, AI’s capabilities are often touted as surpassing those of human professionals. This wave of technological advancement has naturally extended to the realm of mental health, with a surge of research exploring AI’s potential in psychiatric diagnosis, particularly for conditions like major depressive disorder (MDD). Publications on machine learning applications in depression diagnosis have become increasingly common, boasting impressive average accuracy rates, often exceeding 80% and sometimes even reaching above 90%. For instance, studies leveraging EEG feature transformation and machine learning have achieved high classification accuracy, suggesting the feasibility of portable, AI-driven systems for depression screening in the future. The accessibility of portable and wearable sensors further fuels the excitement, hinting at continuous, real-time mental health monitoring integrated into daily life.
This enthusiasm is partly driven by the recognized limitations of traditional psychiatric diagnosis. Psychiatrists, often facing heavy workloads, rely heavily on subjective evaluations and personal experience, leading to concerns about consistency and accuracy. Misdiagnosis rates can be alarmingly high. A study in Ethiopia revealed over a third of severe psychiatric disorder patients were misdiagnosed, and in primary care settings in Canada, misdiagnosis rates for conditions like major depressive disorder and anxiety disorders have been reported to be even higher, sometimes exceeding 90%. The current diagnostic process, heavily reliant on patient-doctor communication and questionnaires, is vulnerable to patient biases, inaccuracies, and subjective interpretations. Furthermore, the global shortage of psychiatrists, particularly in developing nations, exacerbates the problem of access to timely and accurate diagnosis. AI-driven diagnosis offers a tempting solution – a potentially objective, efficient, and scalable method to assist psychiatrists, reduce stigma, and improve access to mental health care.
However, despite the promising research and potential benefits, the widespread adoption of AI in psychiatric diagnosis remains limited. This raises a crucial question: Why, given the apparent progress, are we not seeing AI systems widely implemented in clinical settings for mental health? To understand this, we need to examine the underlying rationale for using AI in this field and then delve into the significant challenges that hinder its practical application.
2. The Logic Behind Machine Learning for Mental Disorder Identification
The fundamental principle behind using machine learning for mental disorder diagnosis rests on the idea that discernible differences exist between individuals with and without mental health conditions. Focusing on depressive disorders as a primary example, research has identified a range of differentiating factors. These include biochemical markers like brain oxygen consumption and neurotransmitter levels, electrophysiological signals captured by EEG, peripheral physiological responses such as heart rate and skin conductance, and non-verbal behavioral cues such as facial expressions, voice characteristics, and linguistic patterns. These features, which distinguish between groups with and without depressive disorders, form the basis for AI classifiers. By feeding these discriminatory metrics into machine learning algorithms, the goal is to train robust predictive models capable of automatically diagnosing depressive disorders.
Facial expressions, in particular, have garnered significant attention as predictors of depression. Being readily observable and strongly linked to emotional states, facial cues are considered a promising avenue for automated analysis. While this discussion will focus on facial expressions as an example, it’s important to note that numerous studies explore other cues, as detailed in various review articles. Facial expressions, categorized into emotions like anger, sadness, joy, surprise, disgust, and fear, are believed to be crucial indicators for depression detection. Individuals with depressive disorders often exhibit reduced facial expressiveness. One study by Gavrilescu and colleagues achieved an 87.2% accuracy in depression identification by analyzing facial expressions using the Facial Action Coding System. Beyond broad emotional categories, specific facial behaviors like the duration and intensity of spontaneous smiles, mouth movements, and the absence of smiling have also been identified as potentially valuable markers for depression. Even subtle cues like pupil changes are now being explored, with research suggesting that slower pupillary responses might be indicative of depression. Reduced eye contact, gaze direction, eyelid movements, and blinking patterns are also being investigated as potential features.
While single-modality approaches, such as relying solely on facial cues, have shown encouraging results, the consensus is that combining multiple data streams – multimodality – could further enhance diagnostic accuracy. Combining visual and auditory cues, and further integrating physiological information, theoretically increases the robustness and precision of automated diagnosis. Multimodality is therefore a key direction in both algorithm development and the creation of comprehensive databases for AI in psychiatric diagnosis.
However, to truly understand the challenges facing AI in this domain, we must consider the nature of the conditions being diagnosed and the complexities inherent in the clinical diagnostic process itself. Do these factors present unique hurdles for AI that differ from its application in other areas of medicine? Using depressive disorders as our case study, let’s explore the specific challenges that arise from the diagnostic criteria and the very nature of mental illness.
3. Diagnostic Criteria: A Source of Challenges
One of the primary obstacles for AI in psychiatric diagnosis stems from the very nature of the diagnostic criteria used for mental disorders. Many diagnostic indicators are rooted in subjective experiences, qualitative descriptions, or concepts that are difficult to objectively measure and standardize. For instance, diagnostic criteria for depressive disorders rely on symptoms like “depressed mood” or “sleep problems.” Despite ongoing efforts to identify physiological biomarkers, there are currently no clinically validated biomarkers that can definitively confirm a diagnosis of major depressive disorder.
Furthermore, the presentation of symptoms in depressive disorders is highly variable across individuals (as illustrated in Figure 1). According to the DSM-5, the two core symptoms of major depressive disorder are (1) persistent depressed mood and (2) significant loss of interest or pleasure. However, a diagnosis requires meeting only a subset of additional symptoms from a list of seven, leading to a wide range of possible symptom combinations. Assessment tools like the PHQ-9, based on DSM-5 criteria, and the HAMD, which focuses on broader symptom categories, highlight this heterogeneity. Symptom presentation also varies across age groups, with irritability being a more prominent depressive symptom in adolescents than persistent sadness. Statistical analyses suggest that there could be over 1497 unique symptom profiles for depression. In fact, individuals with the same diagnosis may not share any single identical symptom.
Figure 1.
Depressive disorders present with a wide array of symptoms and frequently co-occur with other conditions such as generalized anxiety disorder and schizophrenia.
Adding to the complexity, depressive disorders encompass a spectrum of related conditions with subcategories and variations, including disruptive mood dysregulation disorder, major depressive disorder, and persistent depressive disorder.
Comorbidity, the presence of multiple disorders, is also highly prevalent in mental health (again, see Figure 1). Depressive disorders frequently co-exist with anxiety and personality disorders and can be easily confused with bipolar disorder or other mental illnesses. Symptom overlap, particularly in areas like sleep or appetite disturbances, necessitates careful differential diagnosis by clinicians, a process prone to subjective bias.
The DSM-5 criteria also stipulate that symptoms must lead to “clinically significant distress or impairment in social, occupational, or other important areas of functioning” and be culturally relevant. This emphasizes the functional impact of symptoms on daily life and the importance of considering sociocultural context.
Furthermore, depressive symptoms are not static or consistently present. Major depressive disorder is characterized by episodic periods of illness rather than a continuous state. Symptom severity can fluctuate, and periods of remission are common.
Finally, depressive disorders are understood to arise from complex interactions between genetic predispositions, environmental factors, physiological processes, and sociocultural influences. The exact causes of depression remain multifaceted and not fully understood. It’s not solely a neurophysiological issue but rather a result of interplay between genetic vulnerability and environmental stressors. This intricate interplay contributes to the diverse subjective experiences and behavioral manifestations of depression, making standardized, objective diagnosis exceptionally challenging.
4. Standard Diagnostic Approaches: Inherent Subjectivity
Given the variable and subjective nature of depressive disorders, the diagnostic process employed by clinicians is necessarily complex and time-consuming, with inherent subjectivity. Current assessments primarily rely on self-report scales and clinician-administered interviews, both largely guided by the DSM and ICD diagnostic manuals.
Self-rating scales, such as the PHQ-9, Zung Self-rating Depression Scale, and Beck Depression Inventory, offer a convenient and efficient method for initial screening. While widely used in research and clinical practice, and achieving reported sensitivity and specificity rates of 80% to 90%, these scales are not without limitations. Clinician-rated scales, like the Hamilton Rating Scale for Depression (HAMD), are also utilized to support diagnostic evaluations.
Clinical interviews remain the gold standard and final step in diagnosis. These in-depth assessments, while more accurate and comprehensive, are also more resource-intensive. The accuracy of diagnosis through interviews depends heavily on the patient’s ability to articulate their experiences, their cognitive abilities, honesty, and crucially, the clinician’s experience, training, and motivation. Accurately assessing the severity of depression requires comprehensive information gathering and thorough clinical expertise. While biological markers like serotonin levels, neurotransmitter dysfunction, and brain structural differences have been investigated as potential indicators of depression, they are not currently definitive diagnostic tools in routine clinical practice.
The complexity of depressive disorders necessitates a holistic approach to diagnosis. Recognizing that depression is not just a mood disorder but also intertwined with sociocultural factors and often associated with significant functional impairment highlights the challenges clinicians face. This complexity also raises questions about the datasets used to train AI models – are they truly representative of the real-world complexities of depression? Can objectively measured features reliably predict such nuanced and multifaceted conditions? Are the annotations used to train AI models truly valid reflections of clinical reality?
5. The Logical Fallacy in Mental Disorder Diagnosis
A fundamental challenge in mental disorder diagnosis, often overlooked, lies in a potential logical fallacy inherent in the diagnostic process itself. When a clinician diagnoses depression based on reported symptoms like persistent low mood and suicidal thoughts, they are essentially reasoning from symptoms to diagnosis. The implicit assumption is that “If depressive disorder, then depressive symptoms.” However, diagnosing by symptoms reverses this logic, employing “If depressive symptoms, then depressive disorder.” This form of reasoning, known as “affirming the consequent,” is a logical fallacy (as outlined in Table 1).
Table 1.
The four formats of conditional reasoning.
Logical Format | Diagnosis | Logical Value |
---|---|---|
DD *→DS ** Modus Ponens | If depressive disorder then depressive symptom | Valid |
Non-DD→non-DS Denying the antecedents | If no depressive disorder then no depressive symptom | Invalid |
DS→DD Affirming the Consequent | If depressive symptom then depressive disorder | Invalid |
Non-DS→non-DD Modus Tollens | If no depressive symptom then no depressive disorder | Valid |
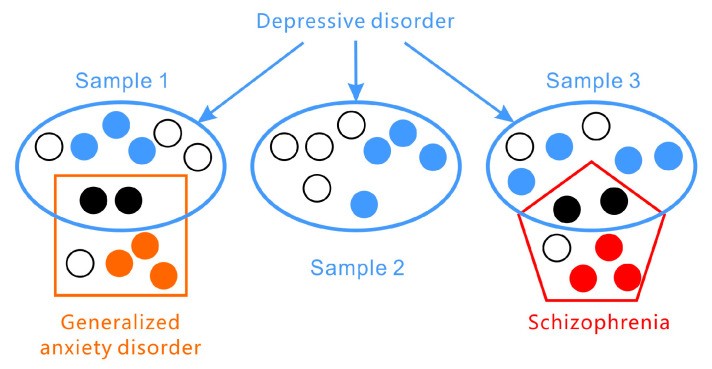
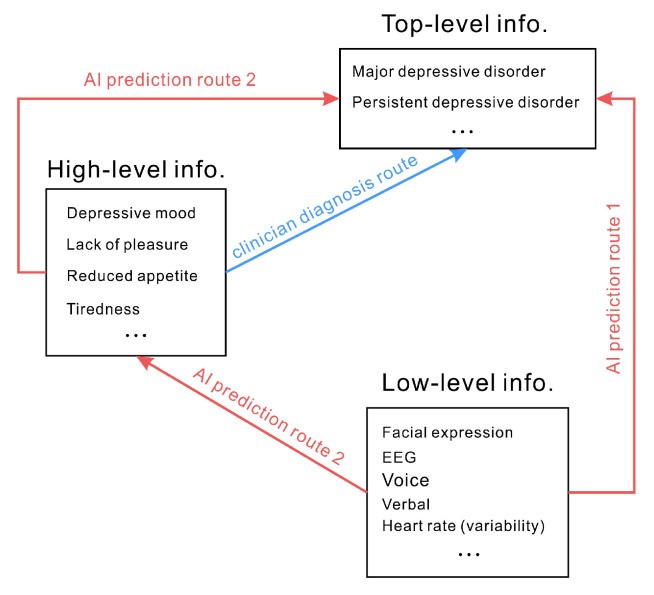
* DD = depressive disorder; ** DS = depressive symptom.
Mental disorder labels, like “depressive disorder,” are essentially descriptive categories for clusters of symptoms. Symptoms, therefore, do not explain the underlying cause of the disorder, nor does the disorder itself fully explain the occurrence of the symptoms. Attributing a diagnosis solely based on symptom presentation can be misleading. For example, persistent low mood might be a reaction to recent negative life events, which may resolve naturally without a depressive disorder being present. Alternatively, depressive symptoms could be secondary to another underlying psychological condition, such as a personality disorder.
The uncertain relationship between mental disorders and their associated symptoms creates significant diagnostic challenges. If AI systems are trained to mimic current clinical diagnostic practices, they may inadvertently inherit this same logical flaw.
6. Dataset Limitations: Real-World Validity and Scope
The performance of AI models heavily relies on the quality and characteristics of the datasets used for training. In the context of mental disorder diagnosis, the accuracy of AI systems is significantly constrained by the limitations of available datasets, particularly concerning sample validity and annotation quality.
6.1. Example Datasets for Depression Recognition
Several datasets have been developed for research in depression recognition, though many remain private and not publicly accessible. Notable publicly available datasets include the AVEC (Audio/Visual Emotion and Depression Recognition Challenge) series (AVEC2013, AVEC2014) and DAIC-WOZ (Distress Analysis Interview Corpus-Wizard of Oz).
AVEC datasets are derived from the Audio-Visual Depression Language Corpus. AVEC2013 features German-language videos of participants performing human-computer interaction tasks while being recorded. Depression severity scores were annotated using the BDI-II. AVEC2014 is a subset with shorter video clips. DAIC-WOZ, annotated using PHQ-8, uses a virtual interviewer with controlled emotional responses. It includes audio, video, and physiological data like galvanic skin response and electrocardiogram information. E-DAIC, an extension of DAIC-WOZ, includes semi-clinical interview data designed for diagnosing anxiety and depression. These datasets, while valuable for research, highlight several limitations.
6.2. Ecological Validity: Are Recorded Data Representative?
Many datasets consist of recordings of individuals in interview settings, either with clinicians or virtual interviewers. While some datasets also capture physiological data and depression scale scores, these recordings are inherently artificial. The interview setting is a specific, performative context. Individuals aware they are being assessed for depression may consciously or unconsciously alter their behavior. A patient in a clinical setting needs to communicate their distress, potentially exaggerating symptoms to convey their condition to the clinician. This “performance” may not accurately reflect their typical emotional state or daily life. Major depressive disorder is episodic, and symptom presentation can vary significantly. The recorded data might capture a specific, potentially heightened, expression of symptoms rather than a representative sample of their everyday behavior. Therefore, AI models trained on such datasets might be optimized for detecting depression in similar interview-like scenarios but may not generalize well to real-world, unscripted settings. Furthermore, factors like language, culture, and specific interview protocols can further limit the generalizability of these datasets.
6.3. Small Sample Size and Data Heterogeneity
Datasets for mental disorder research often suffer from small sample sizes. Ethical considerations and the sensitive nature of mental health data limit the feasibility of collecting large, publicly available datasets. The limited number of subjects restricts the statistical power of AI models and their ability to learn complex patterns and generalize effectively.
Data heterogeneity also poses a significant challenge. Variations in data collection methods, recording equipment, participant demographics, and diagnostic criteria across different datasets make cross-dataset validation and generalization difficult. For example, variations in imaging data acquisition and processing can hinder the transferability of models trained on one dataset to another. This lack of standardization and the limited size of datasets make it challenging to draw robust, generalizable conclusions from AI-based diagnostic studies.
6.4. Oversimplification of Diagnostic Categories
Many datasets simplify the complex reality of mental disorders by categorizing samples into binary groups: “depressed” versus “healthy.” This binary classification, while convenient for machine learning, is a gross oversimplification. In reality, mental health exists on a spectrum, and diagnostic categories are not always clear-cut. Furthermore, datasets often focus solely on depression, neglecting the complexities of comorbidity and differential diagnosis. Patients presenting in clinical settings have diverse and often overlapping symptoms, making accurate diagnosis a nuanced process. The simplified categories in many datasets fail to capture this clinical complexity. The use of continuous BDI scores, as in the AVEC series, represents an improvement, but even these datasets primarily focus on the depression dimension in isolation.
The artificiality, small sample sizes, limited ecological validity, and simplified categories of existing datasets contribute to a potential “logical paradox” in AI-driven diagnosis. AI models trained on these datasets may achieve high accuracy within the confines of the dataset but may fail to perform effectively when faced with the complexities and variability of real-world clinical data. This raises serious concerns about the practical applicability of AI diagnostic systems trained on current datasets.
7. Annotation Challenges: Subjectivity and Interpretation
Data annotations are the bedrock of supervised machine learning. However, the validity and interpretability of annotations in mental disorder datasets raise critical concerns, particularly regarding the subjective nature of mental illness and the mapping between objective measures and subjective experiences.
7.1. Annotation Validity: What Are We Actually Measuring?
In AVEC2013, for instance, researchers were tasked with predicting self-reported depression levels, as indicated by BDI scores, based on video recordings. This annotation approach is problematic from a clinical perspective. Assigning a depression score based solely on observing a person’s performance in a video is questionable. The BDI, a self-report scale, relies heavily on internal subjective experiences and symptoms not directly observable by external observers. Furthermore, as previously discussed, depressive symptoms are not constant. An individual’s behavior during a recorded session may not accurately reflect their overall depression severity or typical symptom presentation. Therefore, using self-report scores derived from questionnaires as the ground truth for video-based AI depression recognition raises concerns about the validity of the annotation itself. If the annotations are not truly representative of the underlying clinical construct of depression, models trained on these annotations may be learning to predict something other than actual clinical depression.
7.2. Mapping Subjective Feelings from Objective Measures: A Fundamental Gap?
Diagnostic criteria for depressive disorders often rely on subjective experiences and qualitative descriptions – “high-level information.” AI approaches, conversely, often focus on measuring objective signals – “low-level information” – such as physiological responses or behavioral mannerisms. Predicting depression from these objective measures implies a direct mapping from low-level signals to high-level subjective experiences (Route 1 in Figure 2). For example, assuming that reduced smiling directly indicates depression and that depression, in turn, signifies a depressive disorder leads to a direct prediction of depressive disorder from smiling behavior. However, the relationship between facial expressions and emotions is complex and not always consistent. Attributing depression directly to a lack of smiling is a significant oversimplification. Similarly, physiological indicators like heart rate or skin conductance are not directly and unequivocally linked to specific mood states or depressive disorders. Using objective verbal and physical indicators to infer mood or diagnose depression is fraught with potential inaccuracies.
Figure 2.
Clinical diagnosis relies on subjective experiences and qualitative descriptions (high-level information). AI often attempts to directly predict high-level information from low-level objective measures (Route 1). An alternative, less common approach (Route 2) involves AI predicting intermediate high-level information before inferring the top-level diagnosis.
A potentially more nuanced approach (Route 2 in Figure 2), less frequently explored, would involve AI first predicting intermediate, higher-level constructs like emotional states or cognitive biases from low-level signals, and then inferring diagnoses based on these intermediate predictions. However, even this approach faces the fundamental challenge of reliably mapping objective measures to subjective experiences and complex clinical constructs. The core issue remains: many objectively measurable signals may have weak, indirect, or even no direct relationship with the complex, subjective reality of mental disorders.
8. Multimodality: A Solution to Complexity or a Source of Noise?
The limitations of relying on single data modalities to capture the complexity of mental disorders have led to the widespread belief that multimodality – integrating information from multiple sources – could be a more effective strategy. The rationale is that by combining different types of data, such as facial expressions, voice patterns, and physiological signals, AI systems could capture a more comprehensive picture of an individual’s mental state and overcome the limitations of any single modality. The idea is that individual variability might be addressed by different modalities being informative for different individuals – facial expressions for person A, voice for person B, and heart rate variability for person C. Multimodality, in this view, could provide the key features needed for accurate depression prediction.
However, the effectiveness of multimodality hinges on the assumption that the different signals are genuinely valid and provide complementary, rather than conflicting, information. In reality, multimodal data can also introduce noise and inconsistencies. For example, individuals experiencing depression can present with vastly different outward expressions – some may become withdrawn and subdued, while others may become irritable and agitated. These diverse presentations can lead to conflicting signals across different modalities. If AI models are to effectively adapt to variations in gender, age, personality, and individual symptom profiles, the required dataset size would need to be enormous, and the feasibility of achieving robust generalization remains questionable. Simply adding more data modalities does not automatically solve the fundamental challenges related to the subjective nature of mental disorders, dataset limitations, and the mapping between objective measures and clinical diagnoses.
9. Conclusions
The desire for objective and reliable diagnostic tools in mental health care has fueled interest in AI-driven psychiatric diagnosis. AI, with its capacity for processing large datasets and identifying subtle patterns, offers the promise of overcoming the inherent subjectivity and limitations of traditional diagnostic methods. While AI holds considerable potential, significant challenges currently impede its widespread clinical application.
These challenges arise from both technical limitations, such as dataset size and ecological validity, and more fundamental issues related to the very nature of mental disorder diagnosis. Current diagnostic criteria rely heavily on symptom-based descriptions that are often subjective and qualitative. AI training data, conversely, are primarily based on objectively measured, low-level information. The attempt to directly predict high-level diagnostic categories from low-level data, without fully understanding the complex relationship between them, may lead to flawed models and inaccurate diagnoses. Despite the advancements in AI techniques for data collection and analysis, a potentially flawed understanding of mental disorders at the conceptual level can undermine the entire AI system development process.
Ultimately, the primary hurdle for AI-based mental disorder diagnosis is not purely technical, nor solely data-related. It is rooted in our incomplete understanding of mental disorders themselves. Addressing the fundamental challenges related to diagnostic criteria, data validity, and the subjective-objective gap is crucial for realizing the true potential of AI in revolutionizing psychiatric diagnosis and improving mental health care.
Author Contributions
Conceptualization, W.-J.Y. and K.J.; writing, Q.-N.R. and W.-J.Y. All authors have read and agreed to the published version of the manuscript.
Conflicts of Interest
The authors declare no conflict of interest.
Funding Statement
This research was funded by the Wenzhou Science and Technology Project of Zhejiang, China (G20210027) and the Zhejiang Medical and Health Science and Technology Project (2023RC273).
Footnotes
Disclaimer/Publisher’s Note: The statements, opinions and data contained in all publications are solely those of the individual author(s) and contributor(s) and not of MDPI and/or the editor(s). MDPI and/or the editor(s) disclaim responsibility for any injury to people or property resulting from any ideas, methods, instructions or products referred to in the content.