1. Introduction
In recent years, Computer-Aided Diagnosis (CAD) has transitioned from a promising concept to an integral part of clinical practice, particularly in breast cancer detection through mammography across numerous screening centers and hospitals in the United States. This widespread adoption signals a significant shift towards leveraging Computerized Diagnosis across various medical imaging modalities and diagnostic applications. Indeed, computerized diagnosis has emerged as a pivotal research area within medical imaging and diagnostic radiology. While initial forays into computer-based medical image analysis began in the 1960s, the systematic and impactful development of computerized diagnosis took root in the 1980s. This era marked a crucial conceptual evolution, moving away from fully automated diagnosis towards computer-aided systems. This article explores the motivations and foundational principles behind the early evolution of computerized diagnosis systems, examines their current standing, and contemplates their future potential within Picture Archiving and Communication Systems (PACS).
2. A Historical Perspective on Computerized Diagnosis Development
Extensive research and development in computerized diagnosis began in the early 1980s at the Kurt Rossmann Laboratories for Radiologic Image Research, within the Radiology Department of the University of Chicago. This initiative was partly inspired by ongoing basic research into the impact of digital images on radiologic diagnosis and the burgeoning field of PACS. While PACS promised improvements in managing radiological images and potential economic benefits for hospitals, its direct clinical advantages for radiologists seemed less clear at the time. This prompted the critical question: “How can the benefits of digital images significantly enhance the daily diagnostic work of radiologists?” This inquiry directly led to the conception of computerized diagnosis.
The 1980s were already acquainted with the idea of automated diagnosis, a concept explored in the 1960s. However, these early attempts at full automation faced limitations. (The distinctions and commonalities between automated diagnosis and computerized diagnosis will be discussed in detail in the subsequent section.) The inherent complexities of analyzing medical images for lesions made it uncertain whether computerized diagnostic systems could be successfully developed. Given this uncertainty, the research focused on areas where successful computerized diagnosis could have a profound impact on healthcare. Cardiovascular diseases, lung cancer, and breast cancer were identified as critical areas. Consequently, three major research projects were initiated: vascular imaging analysis by Fujita and Hoffmann, lung nodule detection in chest radiographs by Giger et al., and clustered microcalcification detection in mammograms by Chan et al.
Our approach to computerized diagnosis development has consistently been guided by three core principles. Firstly, our methodology for lesion detection and quantification in medical images is rooted in understanding the cognitive processes radiologists employ during image interpretation. This approach recognizes radiologists as highly skilled professionals performing complex diagnostic tasks. Therefore, computer algorithms should ideally be developed based on mimicking or complementing these interpretive processes—understanding how radiologists detect abnormalities, why they might miss certain findings, and how they differentiate between benign and malignant conditions.
Secondly, we aimed to measure success by the widespread clinical adoption of computerized diagnosis in hospitals globally. Achieving this required commercialization by medical industries. Therefore, we focused on patenting fundamental technologies underlying computerized diagnosis to encourage industry partnerships and the development of commercial products. Our initial patent in this area, filed in 1987, has become a highly cited reference in computerized diagnosis technology.
Thirdly, promoting the widespread acceptance and global research collaboration in computerized diagnosis was crucial. Recognizing that the field’s advancement required extensive efforts across numerous lesion types, imaging modalities, clinical studies, and commercialization, we fostered a collaborative rather than competitive environment among researchers. For over two decades, we presented comprehensive scientific exhibits at the Radiological Society of North America (RSNA) annual meetings, showcasing computerized diagnosis research in chest, breast, and vascular imaging. In 1993, a real-time demonstration of computerized microcalcification detection on mammograms using a film digitizer and computer was set up at RSNA. This informal validation with 118 breast radiologists yielded promising results. From 1996 to 2001, observer performance studies at RSNA meetings allowed numerous radiologists to experience computerized diagnosis firsthand, demonstrating its practical utility in lesion detection on chest images. These demonstrations effectively promoted the computerized diagnosis concept broadly and rapidly.
In lung cancer research, we focused on developing computerized schemes for lung nodule detection in chest radiographs. It is well-documented that radiologists can miss up to 30% of lung nodules due to overlapping anatomical structures that obscure these lesions. This background “noise” in chest images was anticipated to be a significant obstacle for computerized detection as well. Therefore, the initial step in computerized lung nodule detection involved background structure suppression. The difference-image technique, which enhances nodules by subtracting a nodule-suppressed image from a nodule-enhanced one, proved effective. This technique has been successfully applied not only to lung nodules but also to microcalcifications and masses in mammograms, and nodules in CT scans.
By the mid-1980s, the Rossmann Laboratories had developed foundational computerized schemes for lung nodule detection in chest images and clustered microcalcification detection in mammograms. While these early systems showed promising sensitivity, they also produced a high number of false positives. The clinical utility of these systems was uncertain. For example, the microcalcification detection system had an average of four false positives per mammogram, despite an 85% sensitivity rate. To assess clinical applicability, observer performance studies were conducted with and without computer assistance. Surprisingly, radiologists’ detection of microcalcifications significantly improved with computer assistance, even with the high false positive rate. This pivotal finding, published in 1990 by H.P. Chan et al., provided the first empirical evidence of the clinical benefits of computerized diagnosis, even in early-stage systems.
Figure 1: Performance improvement in computerized microcalcification detection. The graph compares the 1993 performance level (circles) with the improved performance of a commercial CAD system (square).
3. Differentiating Computerized Diagnosis from Automated Computer Diagnosis
Early research in quantitative medical image analysis in the 1960s explored the potential for computers to replace radiologists in abnormality detection. This era was characterized by the concept of automated computer diagnosis, driven by the assumption that machines could outperform humans in certain diagnostic tasks. While these initial efforts yielded interesting results, they were ultimately limited by the technology of the time: insufficient computing power, underdeveloped image processing techniques, and limited access to digital images. However, a fundamental flaw was an overestimation of what computers could realistically achieve in complex medical diagnosis. Despite decades of attempts across various medical fields, automated computer diagnosis struggled to replicate the nuanced decision-making of physicians. As R.L. Engle noted in a review of 30 years of experience in hematology-related automated diagnosis, the pursuit of computer programs to simulate physician decision-making showed limited promise.
The 1980s witnessed the rise of an alternative approach: computerized diagnosis. This concept recognized the computer as a tool to assist, not replace, radiologists. Computerized diagnosis quickly gained traction and widespread acceptance. However, initial skepticism from some computer scientists questioned its viability, a criticism that has since been disproven by the successful clinical implementation of CAD systems. This skepticism likely stemmed from the earlier failures of automated computer diagnosis, highlighting how past research setbacks can sometimes create biases against related but fundamentally different approaches.
The distinction between automated computer diagnosis and computerized diagnosis remains relevant today. Some researchers continue to pursue fully automated systems. Others believe that current computerized diagnosis technologies could evolve into primary diagnostic tools, essentially becoming automated. A 2002 panel discussion at CARS in Paris and AAPM in Montreal revealed divided opinions, with roughly half of participants predicting a shift towards automated computer diagnosis within 50 years, and half disagreeing. Understanding the commonalities and differences between these two concepts is therefore crucial. Both computerized diagnosis and automated computer diagnosis rely on quantitative analysis of digital medical images by computers, necessitating the development of sophisticated computer algorithms.
The primary divergence lies in how the computer output is used in diagnosis. Computerized diagnosis uses computer output as a “second opinion,” with radiologists retaining final decision-making authority. In confident diagnoses, radiologists might confirm or disregard computer findings. However, in uncertain cases, computer assistance can improve diagnostic accuracy, provided the computer’s analysis is correct. The effectiveness of computerized diagnosis doesn’t require computers to outperform radiologists; instead, it leverages a synergistic effect, combining human expertise with computational capabilities. This collaborative approach has been key to the practical clinical success of computerized diagnosis.
Automated computer diagnosis, conversely, demands very high computer performance. If a computer’s lesion detection sensitivity were lower than that of the average physician, its clinical utility would be questionable. Patients in developed countries expect diagnostic standards at least comparable to those of human physicians. Furthermore, insurance reimbursement for automated diagnosis might be problematic if performance benchmarks are not met. High false-positive rates would also hinder automated diagnosis, as physicians would still need to verify every computer-flagged case, negating the automation benefit. Therefore, automated computer diagnosis requires both high sensitivity and high specificity – a challenging benchmark for computer algorithm developers in medical image analysis. Despite the success of computerized diagnosis in mammography, current computer performance levels are generally insufficient for fully automated diagnosis in most advanced healthcare settings.
Another critical distinction is in performance evaluation. Automated computer diagnosis performance is solely measured by computer accuracy. Computerized diagnosis, however, is evaluated by the overall performance of the physician using the computer output as an aid. The effectiveness of computerized diagnosis hinges on how well physicians can integrate and utilize computer-generated insights to improve their diagnostic accuracy. Even with imperfect computer performance (e.g., moderate sensitivity and specificity), physicians can still effectively leverage computerized diagnosis. For instance, computers might detect subtle lesions that are easily missed by human eyes, while physicians can readily dismiss obvious computer-generated false positives. However, poor computer performance would render automated computer diagnosis impractical.
Therefore, computerized diagnosis assessment requires evaluating not just computer performance, but also the performance of physicians using these tools. Receiver Operating Characteristic (ROC) analysis is essential for quantifying how computerized diagnosis enhances physician performance. Crucially, even if a computer’s ROC curve for microcalcification detection is lower than that of radiologists, radiologists using computerized diagnosis can achieve an improved ROC curve. This improvement, first demonstrated by H.P. Chan et al. in 1990, provided the first scientific validation of computerized diagnosis benefits in lesion detection. Subsequent studies have confirmed the value of computerized diagnosis in detecting various lesions, including microcalcifications and masses in mammograms, lung nodules and interstitial opacities in chest radiographs, lung nodules in CT scans, and intracranial aneurysms in MRA. These findings have fueled further research and development of computerized diagnosis across diverse medical imaging applications.
In summary, while sharing the quantitative image analysis aspect, computerized diagnosis and automated computer diagnosis differ significantly in their approach and performance requirements. Automated computer diagnosis is algorithm-centric, whereas computerized diagnosis is physician-centric, equally valuing the roles of both human and machine. This fundamental difference leads to vastly different performance expectations. Automated systems must match or exceed physician performance, while computerized diagnosis systems need only be complementary, enhancing rather than replacing human expertise. The widespread clinical adoption of computerized diagnosis in mammography, despite computer performance levels being below average physician performance, underscores its practical utility as a valuable assistive tool.
4. Current Status of Computerized Diagnosis Research
The volume of research in computerized diagnosis is evident in the number of related papers presented at RSNA meetings between 2000 and 2005 (Table 1). While chest, breast, and colon applications dominated, research extended to other organs including the brain, liver, and skeletal and vascular systems. Notably, cancer detection in breast, lung, and colon, areas undergoing screening programs, has been a major focus. Screening programs often yield predominantly normal results, making the detection of rare suspicious lesions challenging and time-intensive for radiologists. This context makes computerized diagnosis particularly valuable in screening settings. Indeed, commercial computerized diagnosis systems for these cancers are now clinically available. Figure 1 illustrates the performance improvement in computerized microcalcification detection, comparing the 1993 performance (87% sensitivity at 1.0 false positive per image) with the estimated current level (98% sensitivity at 0.25 false positive per image) of commercial systems. This substantial progress demonstrates the impact of industrial development in translating academic research into clinically effective tools.
Table 1. CAD Papers at RSNA Meetings (2000-2005)
Year | 2000 | 2001 | 2002 | 2003 | 2004 | 2005 |
---|---|---|---|---|---|---|
Chest | 22 | 37 | 53 | 94 | 70 | 48 |
Breast | 23 | 28 | 32 | 37 | 48 | 49 |
Colon | 4 | 10 | 21 | 17 | 15 | 30 |
Brain | – | 4 | 2 | 10 | 9 | 15 |
Liver | 3 | – | 5 | 9 | 9 | 9 |
Skeletal | 2 | 7 | 7 | 9 | 8 | 5 |
Vascular etc | 5 | – | 12 | 15 | 2 | 7 |
Total | 59 | 86 | 134 | 191 | 161 | 163 |
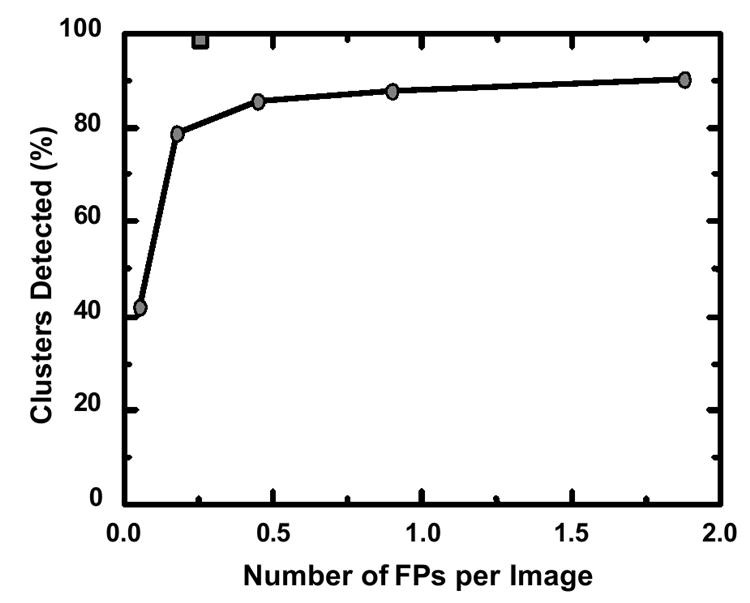
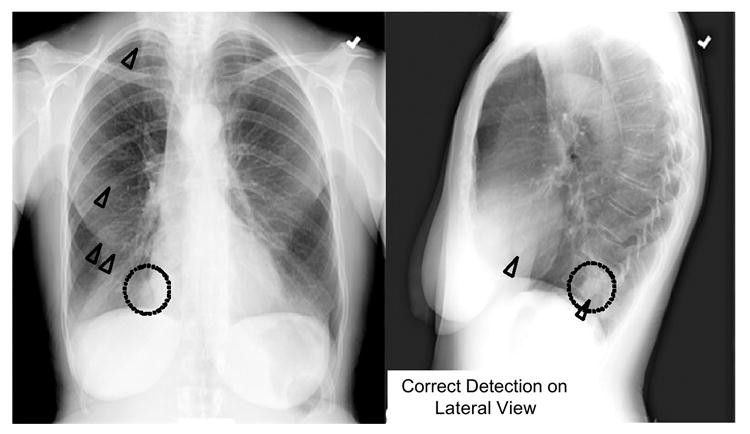
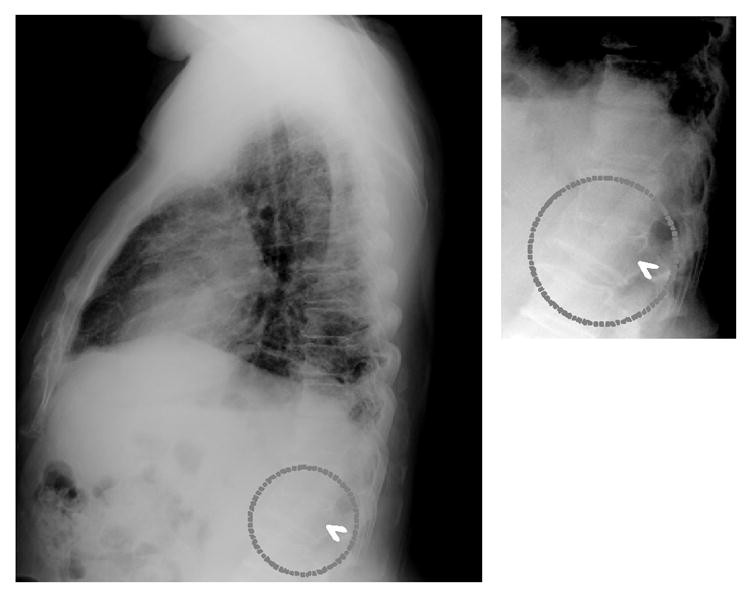
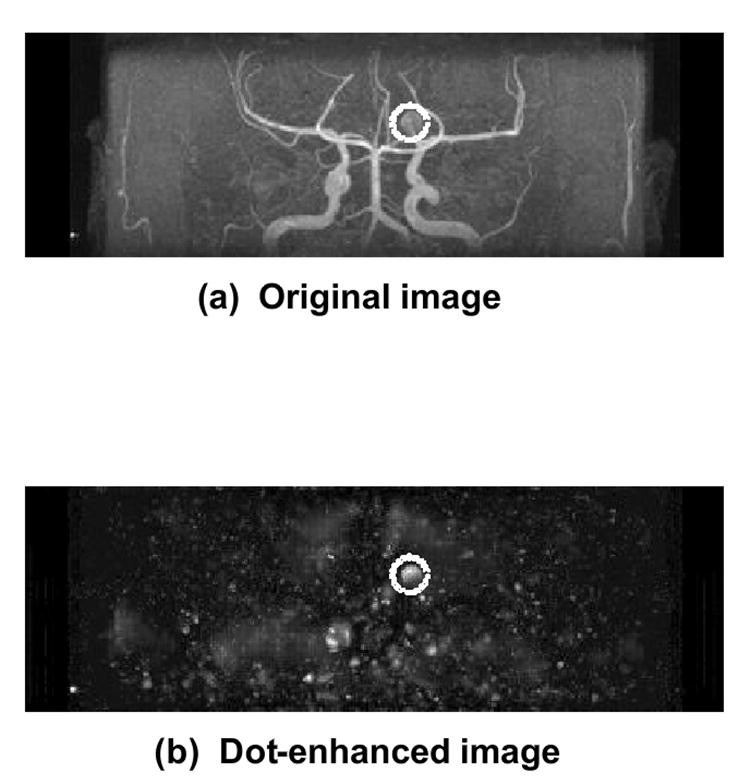
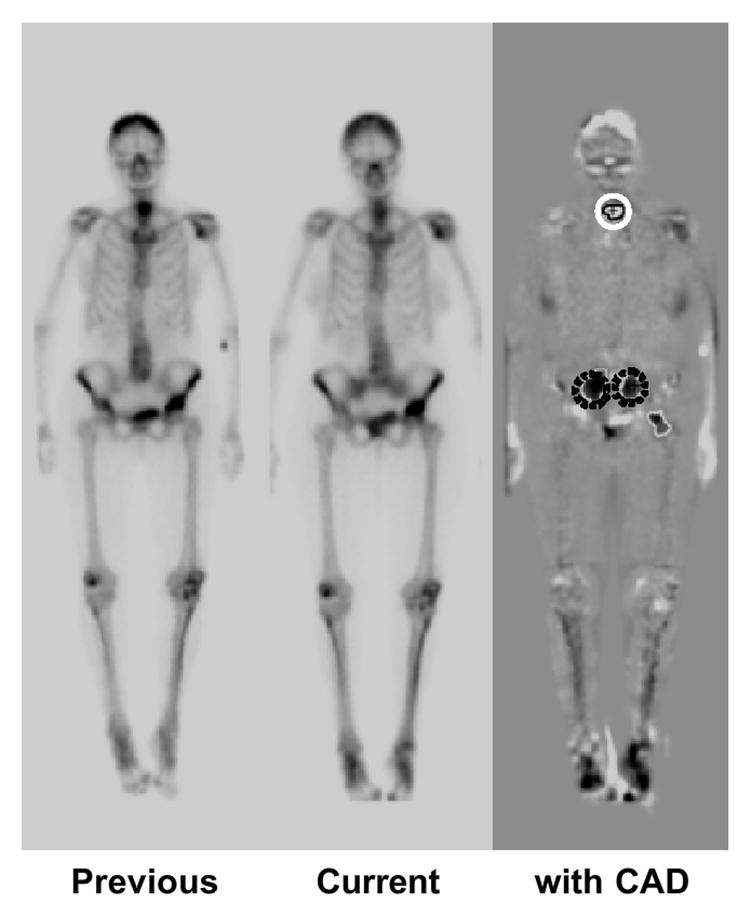
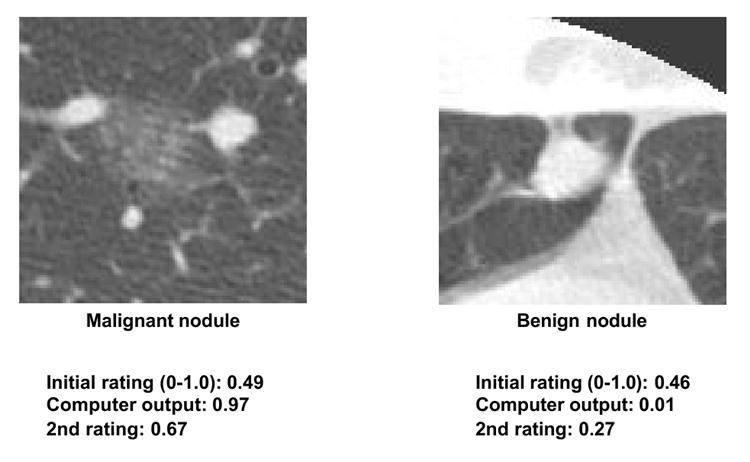
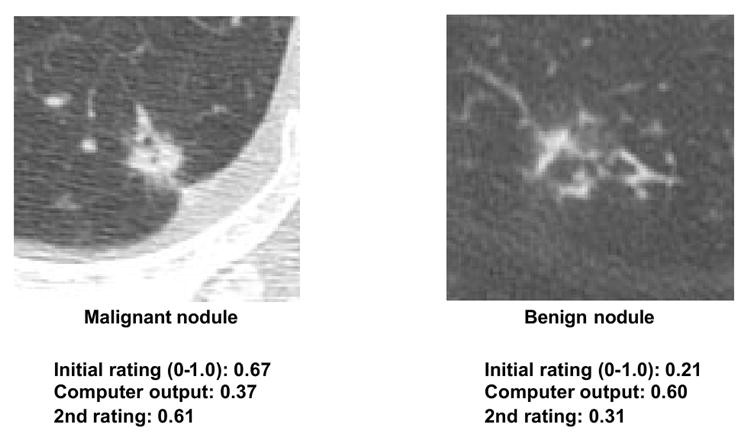
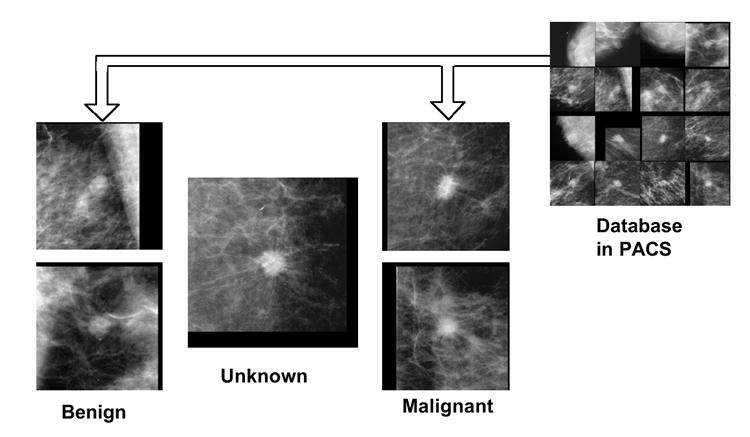
Prospective mammography studies involving large screening populations (8,682 to 115,571 women) have examined the impact of computerized diagnosis on breast cancer detection rates (Table 2). While results vary, all studies showed an increase in cancer detection with computerized diagnosis. Cupples et al. reported a 164% increase in detecting small invasive cancers (<1 cm) and a 5.3-year reduction in the average patient age at diagnosis when computerized diagnosis was used in mammography.
Table 2. Prospective Studies of CAD in Screening Mammography
Authors | Cases | Cancer Detection Rate Gain | Recall Rate Increase |
---|---|---|---|
Freer et al. | 12,860 | 19.5% | 18.8% |
Gur et al. | 115,571 | 1.7% | 0.1% |
Birdwell et al. | 8,682 | 7.4% | 7.6% |
Cupples et al. | 27,274 | 16.1% | 8.1% |
Morton et al. | 18,096 | 7.6% | 10.8% |
Dean et al. | 9,520 | 10.8% | 26.0% |
Several review articles in this journal issue cover computerized diagnosis for mammography, chest radiography, thoracic CT, CT colonography, and multi-organ applications. The following sections highlight recent advancements in selected areas.
4.1 Lung Nodule Detection in PA and Lateral Chest Radiographs
Computerized diagnosis schemes are prevalent in chest radiography and thoracic CT, with some commercial systems available. These schemes address lung nodule detection and differential diagnosis, interstitial lung disease diagnosis, cardiomegaly detection, pneumothorax detection, and detection of interval changes. However, many systems have historically focused solely on posterior-anterior (PA) chest images, even when lateral views were available. Lateral chest views, commonly acquired alongside PA views, offer complementary diagnostic information. Despite past debates on the necessity of both PA and lateral views, integrating lateral views into computerized diagnosis can enhance lung nodule detection and improve radiologist decision-making.
Shiraishi et al. recently developed a computerized scheme for lung nodule detection specifically in lateral chest radiographs to complement PA view analysis. Using PA views alone, nodule detection sensitivity was 70.5% with 4.9 false positives per image. While the lateral view system had a lower sensitivity (60.7% with 1.7 false positives per image), combining both views improved the overall sensitivity to 86.9% (6.6 false positives per two views). Notably, 16.4% of nodules in their study were detected only on lateral views. Figure 2 illustrates subtle lung nodules in the mediastinum region, missed by computerized diagnosis in the PA view but correctly identified in the lateral view. Thus, lateral view computerized diagnosis can significantly improve overall lung nodule detection when combined with PA view systems.
Figure 2: A subtle lung nodule (dotted circles) correctly identified by CAD (triangles) in the lateral view, but missed in the PA view.
4.2 Vertebral Fracture Detection on Lateral Chest Radiographs
Vertebral fractures, a common consequence of osteoporosis, are a major public health concern. Early detection is crucial for timely intervention to reduce subsequent fracture risk. Lateral chest radiographs can reveal vertebral fractures, but studies show that about 50% of visible fractures are underdiagnosed or underreported, even severe cases. Kasai et al. developed a computerized method for vertebral fracture detection on lateral chest radiographs to aid radiologists and improve early osteoporosis diagnosis. Their method automatically extracts a curved area containing vertebral endplates and straightens it for horizontal orientation. A horizontal line-enhancement filter highlights edge candidates, and multiple thresholding and feature analysis identify vertebral endplates. Vertebral height is then measured and compared to expected height to detect fractures.
Initial results showed 95% sensitivity in fracture case detection (19/20) with 1.03 false positives per image in a dataset of 20 fracture and 118 non-fracture patients. Validation on 32 independent fracture cases yielded 75% sensitivity at 1.03 false positives per image. Figure 3 shows correct computerized detection of a fractured vertebra. Computerized vertebral fracture detection can serve as a “second opinion,” enhancing radiologist accuracy and improving early osteoporosis diagnosis.
Figure 3: Computerized detection (arrowhead) of a fractured vertebra (dotted circles) on a lateral chest radiograph.
4.3 Intracranial Aneurysm Detection in MRA
Computed tomographic angiography (CTA) and magnetic resonance angiography (MRA) have become important “less invasive” modalities for intracranial aneurysm detection. However, detecting small aneurysms, or even medium-sized ones in challenging locations or obscured by vessel overlap on maximum-intensity-projection (MIP) images, remains difficult and time-consuming. Computerized diagnosis can assist radiologists in detecting these aneurysms, especially smaller ones, in MRA. Arimura et al. developed a computerized scheme for automated unruptured intracranial aneurysm detection in MRA, using a 3D selective enhancement filter for dot-like structures (aneurysms). Isotropic 3D MRA images are processed with a multi-scale enhancement filter (Figure 4). Initial candidates are identified through gray-level thresholding and region-growing techniques. Candidates are then classified by size and local structure into large, short-branch, single-vessel, and bifurcation types. False positives are reduced using rules based on localized image features and linear discriminant analysis.
Figure 4: (a) Original 3D MRA image; (b) Dot-enhanced image after processing with a selective filter for aneurysm detection (dotted circles).
Evaluation on 207 aneurysm cases from three institutions using different MR equipment showed an average sensitivity of 96% for aneurysms ranging from 1mm to 23mm, with 3.2 false positives per patient. An observer study with 15 radiologists using 22 aneurysm and 28 non-aneurysm cases showed that computerized diagnosis improved the average ROC curve area (Az value) from 0.931 to 0.983 (p=0.001). This demonstrates the utility of computerized diagnosis in assisting intracranial aneurysm detection in MRA.
4.4 Interval Change Detection in Successive Whole-Body Bone Scans
Bone scintigraphy is a common nuclear medicine procedure for diagnosing osseous metastases and monitoring tumor response to therapy. While highly sensitive, identifying multiple lesions and subtle interval changes between successive bone scans is time-consuming and challenging due to variations in patient conditions, radioisotope accumulation, and image quality. Shiraishi et al. developed a computerized diagnosis scheme for interval change detection in successive bone scans using temporal-subtraction images obtained with nonlinear image warping. This scheme involves image normalization, image matching, temporal subtraction, initial change detection, feature extraction, rule-based false positive reduction, and computer output display.
In a study of 58 bone scan pairs, 107 “gold-standard” interval changes (71 hot, 36 cold lesions) were identified. Computerized diagnosis achieved 95.3% sensitivity with 5.97 false positives per view (resubstitution method) and 83.2% sensitivity with 6.02 false positives (leave-one-case-out method). Figure 5 shows a temporal subtraction image and correct computerized detection of cold and hot lesions. Temporal subtraction images enhance interval changes, and computerized diagnosis can effectively assist radiologists in interpreting successive bone scans.
Figure 5: Temporal subtraction image from successive bone scans, showing correct computerized detection of a cold lesion (white circle) and hot lesions (dark circles).
5. Future Potential of Computerized Diagnosis in PACS
In the future, computerized diagnosis schemes are likely to be integrated into modality-specific workstations (e.g., digital mammography, CT, MRI) and PACS. Comprehensive chest computerized diagnosis packages might include detection of lung nodules, interstitial opacities, cardiomegaly, vertebral fractures, interval changes, and also lesion classification (benign/malignant) and differential diagnosis of interstitial lung diseases. All chest images could be automatically screened for various abnormalities, with potential lesion sites and associated information (malignancy likelihood, disease probability) displayed on workstations. Crucially, reducing false positives will be essential for clinical utility, ensuring radiologists are alerted primarily to clinically significant findings.
Radiologists could utilize these packages in two modes: reading images first, then reviewing computer output, or viewing computer output initially. The first mode might prevent computer-induced oversight of subtle lesions initially suspected by the radiologist but could increase reading time. The second mode could reduce reading time, but risks missing lesions not flagged by the computer (false negatives). High sensitivity across a comprehensive computerized diagnosis package could mitigate this risk. For instance, while individual schemes might miss nodules or opacities, temporal subtraction from prior images can highlight subtle interval changes. This synergistic effect of combining multiple complementary computerized diagnosis schemes within PACS is a significant advantage.
Computerized diagnosis research has focused on both lesion detection and differential diagnosis (benign/malignant classification, disease differentiation). While lesion detection systems (e.g., mammography) are clinically implemented, differential diagnosis systems are less mature commercially. However, reported performance levels for differential diagnosis are promising. For example, a thoracic CT lung nodule classification system estimates malignancy likelihood (%). Observer studies have shown that radiologists using this system outperform both radiologists alone and computer output alone. Radiologists generally adjust their confidence levels based on the computer’s malignancy likelihood, with beneficial effects. Computerized diagnosis assists in subtle cases, while radiologists maintain their correct judgments in obvious cases, even with incorrect computer outputs. This synergistic improvement highlights the value of computerized diagnosis as a “second opinion,” enhancing decision-making in challenging cases while preserving human expertise in clear-cut diagnoses.
Figure 6: Subtle lung nodules in HRCT where CAD output assists radiologists in diagnosis.
Figure 7: Obvious lung nodules in HRCT where radiologists maintain correct judgments despite CAD output.
To further enhance differential diagnosis, providing similar benign and malignant images from a PACS database could be beneficial. Visual comparison with similar known cases can bolster diagnostic confidence. Studies have demonstrated the utility of similar image retrieval in improving diagnostic accuracy for lung nodules in CT and lesions in mammography. Figure 8 shows a comparison of an unknown mammogram mass with similar benign and malignant masses. In this example, observers can correctly identify the unknown case as more similar to malignant masses.
Figure 8: Comparison of an unknown mammogram mass (center) with similar benign (left) and malignant (right) masses retrieved from PACS.
Two key challenges for similar image retrieval are creating a comprehensive image database within PACS and developing sensitive tools for identifying similar images. While demanding, a valuable database can be built from PACS archives. Currently, most PACS images are underutilized beyond immediate clinical needs. “Awakening” these “sleeping” images for daily clinical use through similar image retrieval holds significant potential. Developing reliable methods for quantifying image similarity for visual comparison is crucial. Recent research suggests psychophysical measures, using neural networks trained with image features and radiologist ratings, can quantify image similarity for lung nodules in CT and mammogram lesions. Further research is needed to validate and refine these tools for PACS-based similar image retrieval.
6. Conclusion
Computerized diagnosis has established itself in breast cancer detection via mammography but is still in the early stages of realizing its full potential across diverse lesions and imaging modalities. As a physician-computer partnership, computerized diagnosis differs fundamentally from automated computer diagnosis. Future integration into PACS, with comprehensive packages for lesion detection and differential diagnosis, will solidify computerized diagnosis as a valuable tool in daily clinical practice, enhancing diagnostic precision and patient care.
Acknowledgments
The author expresses gratitude to over 200 individuals at the University of Chicago who contributed to computerized diagnosis research; to Charles E. Metz, PhD, and Shigehiko Katsuragawa, PhD, for manuscript review; and to Mrs. E. Lanzl for manuscript editing. This work was supported by USPHS Grants CA62625 and CA 98119. K.D. is a shareholder of R2 Technology, Inc. CAD technologies from the Kurt Rossmann Laboratories have been licensed to various companies. The University of Chicago policy mandates public disclosure of potential financial interests related to research activities.
Footnotes
Publisher’s Disclaimer: This is an early manuscript version accepted for publication and will undergo further editing and review before final publication. Errors may be present and legal disclaimers apply to the journal.