Introduction
Misdiagnosis of tremor syndromes remains a significant challenge in clinical practice and research, impacting patient care and the reliability of clinical studies. Currently, the clinical evaluation by a movement disorders expert is the gold standard for diagnosis, as no validated neurophysiological technique has demonstrated consistently high classification performance. This article introduces the Tremor Stability Index (TSI), a groundbreaking neurophysiological measure derived from kinematic tremor recordings, which exhibits exceptional accuracy in differentiating between Parkinson’s disease tremor and essential tremor. This novel Differential Diagnosis Tool offers a robust, objective, and readily accessible method to improve diagnostic precision in these common tremor types.
The problem of misdiagnosis in tremor syndromes is not trivial. Studies have shown that even among movement disorder specialists, diagnostic accuracy for Parkinson’s disease is around 80%, dropping to 74% for general neurologists. Using strict diagnostic criteria, misdiagnosis still occurs in approximately 20% of Parkinson’s disease cases, and this figure may be even higher for patients presenting primarily with tremor. Essential tremor diagnosis, lacking a definitive gold standard, relies solely on clinical criteria, and misdiagnosis rates are reported as high as 37%, with Parkinson’s disease tremor being the most frequent misdiagnosis. The early stages of these diseases pose the greatest diagnostic difficulty, especially when typical Parkinsonian signs are subtle or absent and the disease course is yet to unfold. Age is also not a reliable differentiating factor due to the possibility of early-onset Parkinson’s disease and late-onset essential tremor.
The clinical differentiation between Parkinson’s disease tremor, typically a resting tremor, and essential tremor, usually postural or kinetic, is not always straightforward. Parkinson’s disease tremor can manifest as a postural tremor, known as ‘re-emergent’ tremor, which appears after a delay upon assuming a posture. However, this delay may be absent in some Parkinson’s patients, presenting as a pure postural tremor. Conversely, in essential tremor, muscle tension during examination can mimic cogwheel rigidity, a Parkinsonian sign.
While nuclear imaging techniques like 123I-FP-CIT and 123I-β-CIT DAT-SPECT have been employed to aid in differentiating Parkinson’s disease from essential tremor, their overall diagnostic accuracy may not surpass that of expert clinical evaluation. Furthermore, these techniques have led to the identification of SWEDD (scans without evidence of dopaminergic deficit), complicating diagnosis as some SWEDD patients later develop Parkinson’s disease. Nuclear imaging is also expensive, time-consuming, requires specialized agents, and is not universally available. For essential tremor, nuclear imaging has limited diagnostic utility.
Clinical neurophysiology offers a more accessible and cost-effective avenue for diagnostic support in tremor conditions. However, most neurophysiological techniques, with a possible exception of mean harmonic power (MHP), have not demonstrated robust classification performance validated across independent cohorts for differentiating Parkinson’s disease and essential tremor. The stability of tremor frequency over time has emerged as a potential diagnostic indicator. Research has shown that essential tremor frequency is stable over a narrow range, while Parkinson’s disease tremor frequency can remain stable across a broader range. The frequency tolerance index, however, might not fully utilize the entire tremor time series to characterize overall tremor stability.
This study focuses on analyzing the overall tremor stability characteristics of Parkinson’s disease and essential tremor to develop a new differential diagnosis tool: the Tremor Stability Index (TSI). We investigate the utility and diagnostic performance of TSI and validate its effectiveness in a separate patient cohort.
Materials and Methods
Patients
This study was conducted with informed consent from all patients and ethical approval from local research ethics committees, adhering to the Declaration of Helsinki. Data collection involved two primary, independent cohorts, each from multiple sources and using different recording devices to ensure the robustness and generalizability of the differential diagnosis tool. Patients were diagnosed by experienced movement disorder specialists based on established clinical criteria: the Queen Square Brain Bank criteria for Parkinson’s disease and the Movement Disorder Society Consensus statement for essential tremor. Clinical diagnoses served as the gold standard for evaluating neurophysiological measures.
The ‘Test’ cohort included 16 Parkinson’s disease patients with rest tremor from the University Campus Bio-Medico of Rome and 20 essential tremor patients with postural tremor from the University Hospital Cologne, Germany. The ‘Validation’ cohort comprised 42 Parkinson’s disease patients with rest tremor and 8 essential tremor patients with postural tremor, incorporating new data and data from previous publications. A third ‘Postural context’ cohort of Parkinson’s disease and essential tremor patients was used to assess TSI’s diagnostic performance across different postural conditions.
Table 1. Composition of Cohorts.
Cohort | Data source | Diagnosis | Number of tremor recordings | Recording state | Age (mean ± SD) | Disease duration (mean ± SD) | Sensor type – producer |
---|---|---|---|---|---|---|---|
Test | University Campus Bio-Medico of Rome | Parkinson’s disease | 16 | Rest | 66.4 ± 8.6 | 6.7 ± 7.7 | Triaxial accelerometer (Opal sensor APDM, Inc) |
University Hospital Cologne, Germany | Essential tremor | 20 | Posture | 49.5 ± 15.5 | 24.3 ± 13.9 | Triaxial accelerometer (Brainvision acceleration sensor) | |
Validation | Previously published [Schwingenschuh et al., 2010](#R26)[a] | Parkinson’s disease | 9 | Rest | 68.7 ± 8.2 | 6.5 ± 3.4 | Triaxial accelerometer (Biometrics Ltd) |
Essential tremor | 8 | Posture | 68.6 ± 7.8 | 20.8 ± 19.4 | Triaxial accelerometer (Biometrics Ltd) | ||
Previously published [Brittain et al., 2015] | Parkinson’s disease | 20 | Rest | 68.2 ± 8.4 | 12.5 ± 2 | Triaxial accelerometer (Twente Medical Systems International, Biometrics Ltd) | |
Previously published [Beuter et al., 2001](#R6)[b] | Parkinson’s disease | 6 | Rest | 58.5 ± 9.8 | 11.5 ± 5.3 | Velocity-transducing laser (Bruel and Kjaer) | |
Previously published [Beudel et al., 2015](#R5)[c] | Parkinson’s disease | 7 | Rest | 49.5 ± 9.5 | 12.5 ± 6.8 | Triaxial accelerometer (Twente Medical Systems International) | |
University of Seville | Essential tremor | 5 | Posture | 51.4 ± 16.4 | 23.8 ± 16.4 | Triaxial accelerometer EGAS3 (Entran Devices) | |
Postural context | University of Oxford | Parkinson’s disease | 4 | Re-emergent | 68.2 ± 7.8 | 10.0 ±8.0 | Triaxial accelerometer (Twente Medical Systems International) |
3 | Postural | ||||||
Previously published [Schwingenschuh et al., 2010](#R26)[a] | Parkinson’s disease | 5 | Re-emergent and rest | 68.7 ± 8.2 | 6.5 ± 3.4 | Triaxial accelerometer (Biometrics Ltd) | |
2 | Postural and rest | ||||||
2 | Rest and low amplitude postural | ||||||
Essential tremor | 8 | Posture and rest | 68.6 ± 7.8 | 20.8 ± 19.4 | Triaxial accelerometer (Biometrics Ltd) |
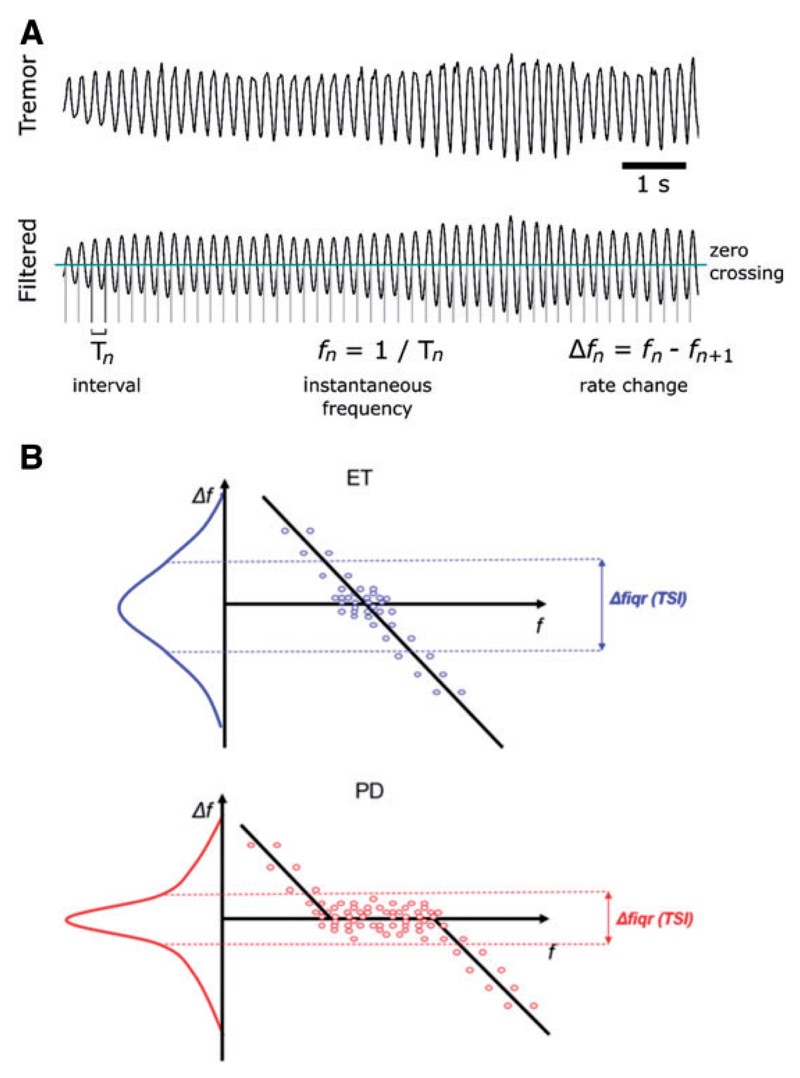
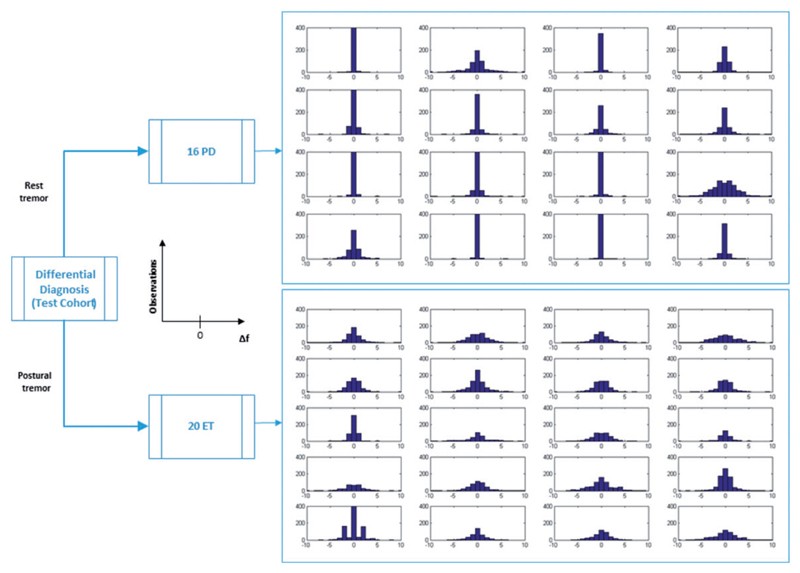
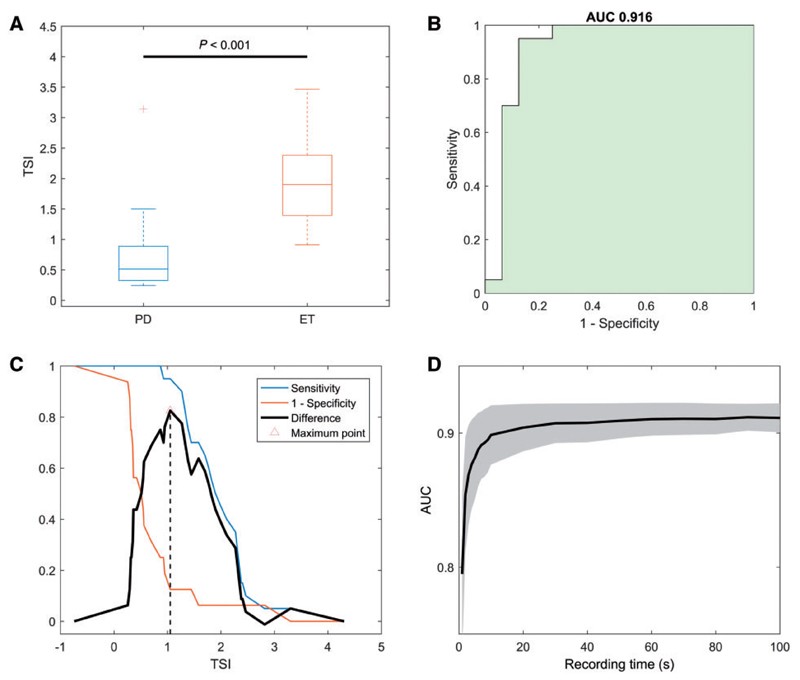
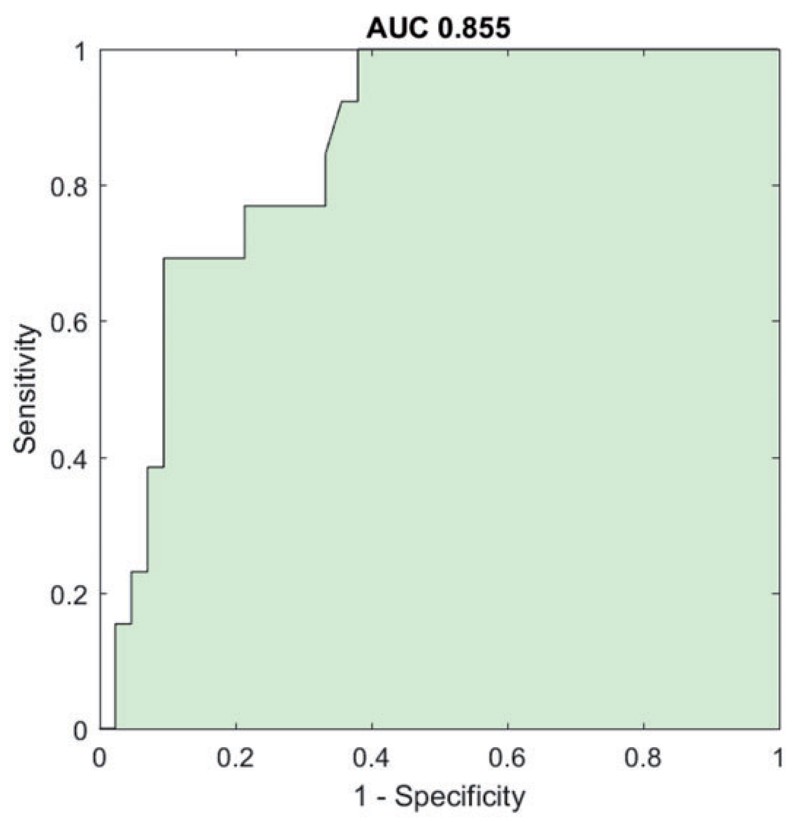
Tremor Recordings
Rest tremor recordings were taken with patients seated, forearms supported, and hands and wrists relaxed. Postural tremor recordings were obtained with arms outstretched horizontally. Triaxial accelerometers were placed over the wrist (test cohort), middle finger, thumb, or hand dorsum (validation and postural context cohorts), or a velocity-transducing laser was used (validation cohort). For each patient, the initial 100 seconds of tremor recording were analyzed. EMG recordings from wrist muscles were also collected in a subset of patients to evaluate TSI performance with EMG data.
Data Analysis
Data were converted to Matlab for analysis. Accelerometer data underwent trend correction and principal component analysis (PCA) to isolate the dominant tremor axis. The first principal component was filtered around the peak tremor frequency (fc) between 2-9 Hz. Instantaneous frequencies (fn) were calculated as the inverse of the interval between zero-crossings, and tremor amplitude was computed using the Hilbert envelope. The cycle-by-cycle frequency variation (Δf = fn − fn+1) was then determined. Signal-to-noise ratio was also calculated. The Tremor Stability Index (TSI) is defined as the interquartile range of Δf.
The diagnostic accuracy of TSI as a differential diagnosis tool was evaluated using binary logistic regression and receiver operating characteristic (ROC) analysis in IBM SPSS Statistics. Sensitivity, specificity, accuracy, likelihood ratios, and area under the ROC curve (AUC) were calculated. A TSI threshold was determined in the test dataset to maximize the combination of sensitivity and specificity and was then cross-validated and verified in the validation and postural context datasets. Bootstrapping was used to assess the minimum recording duration required for reliable TSI estimation. Mean harmonic power (MHP) was also computed for comparison.
Figure 1. Schematic illustration of the procedure used to extract (A) the instantaneous frequency and variation in frequency, and (B) the TSI.
Results
Discriminating Parkinson’s Disease and Essential Tremor
Test Cohort
Analysis of the Δf distribution in the test cohort showed that Parkinson’s disease patients exhibited a narrower and sharper distribution compared to essential tremor patients.
Figure 2. Test cohort: ∆f distribution.
The TSI, derived from the Δf interquartile range, was significantly different between the groups (p<0.001), with Parkinson’s disease showing a lower TSI. Binary logistic regression indicated that a unit increase in TSI increased the odds of essential tremor diagnosis by 14.8 times. ROC analysis for TSI as a differential diagnosis tool yielded an AUC of 0.916 (95% CI 0.797–1.000), outperforming other frequency variability measures.
Figure 3. Test cohort: TSI diagnostic performance.
The optimal TSI threshold of 1.05 achieved excellent diagnostic performance in the test cohort, with 95% sensitivity and 88% specificity for differentiating essential tremor from Parkinson’s disease. Reliable TSI estimation was possible with tremor recordings as short as 10 seconds, and follow-up recordings confirmed TSI stability over time in Parkinson’s disease patients.
Table 2. ROC AUC values of tremor neurophysiological parameters.
AUC[a] | Asymptotic 95% CI |
---|---|
Lower bound | |
TSI | 0.916 |
Mean frequency | 0.694 |
ΔfSD | 0.784 |
Δfcov | 0.409 |
FSD | 0.781 |
Fcov | 0.791 |
Table 3. TSI diagnostic performance on test cohort.
Diagnosis | Essential tremor versus Parkinson’s disease | Parkinson’s disease versus essential tremor |
---|---|---|
Sensitivity | 95% | 88% |
Specificity | 88% | 95% |
Accuracy | 92% | 92% |
Likelihood ratio positive | 7.60 | 17.50 |
Likelihood ratio negative | 0.06 | 0.13 |
Validation Cohort
The validation cohort also showed a significant difference in TSI between Parkinson’s disease and essential tremor (p<0.001). ROC analysis confirmed the high diagnostic accuracy of TSI as a differential diagnosis tool, with an AUC of 0.855 (95% CI 0.754–0.957).
Figure 4. Validation cohort: ROC curve.
Applying the 1.05 TSI threshold to the validation cohort demonstrated excellent diagnostic performance, with high sensitivity for Parkinson’s disease and specificity for essential tremor, despite the heterogeneity within the validation dataset. In a sub-cohort with SPECT-DaTSCAN imaging confirmation, TSI accuracy remained high at 82%.
Table 4. TSI diagnostic performance on validation cohort.
Diagnosis | Essential tremor versus Parkinson’s disease | Parkinson’s disease versus essential tremor |
---|---|---|
Sensitivity | 69% | 90% |
Specificity | 90% | 69% |
Accuracy | 85% | 85% |
Likelihood ratio positive | 7.27 | 2.94 |
Likelihood ratio negative | 0.34 | 0.14 |
Comparison with State-of-the-Art Neurophysiology
In comparison with MHP, the current gold standard neurophysiological measure, TSI demonstrated superior performance. Bootstrap analysis across combined datasets showed TSI outperformed MHP in 72% of iterations, indicating TSI’s enhanced discriminatory power as a differential diagnosis tool.
TSI Independence of Posture and Recording Device
Further analysis indicated that TSI’s diagnostic accuracy was not affected by whether tremor was recorded at rest or during postural contraction. However, EMG was found to be less effective than kinematic sensors for TSI estimation, suggesting kinematic data better captures the temporal variability of tremor relevant for differential diagnosis.
Discussion
Accurate differentiation between Parkinson’s disease and essential tremor is crucial for appropriate clinical management and research validity. The Tremor Stability Index (TSI) emerges as a significant advancement, offering a robust, objective, and highly accurate differential diagnosis tool. Derived from brief, simple tremor recordings, TSI effectively distinguishes between Parkinson’s disease tremor and essential tremor with high sensitivity, specificity, and overall diagnostic accuracy.
The validation of TSI in a large, independent cohort, encompassing diverse datasets and recording methodologies, underscores its reliability and practical utility. Its consistent performance across different postural contexts further strengthens its clinical applicability. The diagnostic accuracy of TSI, approximately 90% in our study, rivals that of costly and less accessible radionucleotide imaging for early Parkinson’s disease diagnosis. Notably, TSI outperformed MHP, the previously most accurate neurophysiological measure for this differential diagnosis.
The rapid estimation of TSI from just 10-second tremor recordings, using various accelerometer devices or laser techniques, highlights its ease of use and broad applicability. Being a quantitative and automatically derived measure, TSI minimizes operator dependency, unlike clinical evaluations or radionucleotide studies. Its independence from postural context makes it a versatile tool for different clinical scenarios.
The Tremor Stability Index holds substantial promise as a valuable differential diagnosis tool in clinical practice and clinical trials, potentially reducing misdiagnosis rates and improving patient selection for research. Beyond its diagnostic utility, TSI may offer deeper insights into the central oscillatory mechanisms underlying different tremor types. Future research should explore TSI’s ability to differentiate dystonic tremor and its robustness across various treatments.
Conclusion
The Tremor Stability Index represents a novel and effective neurophysiological measure for the differential diagnosis of Parkinson’s disease and essential tremor. Its high diagnostic accuracy, objective derivation from short, inexpensive, and non-invasive tremor recordings, and independence from postural context establish TSI as a valuable asset for improving diagnostic precision and clinical management of these common tremor disorders.
Supplementary Material
Supplementary material is available at Brain online.
NIHMS72707-supplement-Suppl.pdf
Funding
This study was funded by the Medical Research Council, the Rosetrees Trust, and the National Institute of Health Research Oxford Biomedical Research Centre. Additional funding was provided by the German Research Council and the Medical Research Council.
Abbreviations
AUC Area Under the Curve
MHP Mean Harmonic Power of Tremor
ROC Receiver Operating Characteristic
SWEDD Scans Without Evidence of Dopaminergic Deficit
TSI Tremor Stability Index
References
[References List from Original Article – Please refer to the original article for the complete list]
Associated Data
Supplementary Materials
Supplementary material