Early and accurate Postoperative Diagnosis of infections, particularly sepsis, remains a significant challenge in healthcare. Sepsis, a life-threatening condition arising from the body’s dysregulated response to an infection, necessitates prompt intervention to improve patient outcomes. Current diagnostic methods often fall short in the crucial early stages, leading to delayed treatment and potentially adverse consequences. This article delves into groundbreaking research focused on leveraging host biomarker signatures for the presymptomatic detection of postoperative infection and sepsis, potentially revolutionizing postoperative diagnosis and patient management.
The Critical Need for Enhanced Postoperative Diagnosis
Sepsis is a leading cause of mortality and morbidity worldwide. The difficulty in achieving early and accurate diagnosis of infection and subsequent organ dysfunction contributes to the disappointing outcomes observed in numerous clinical trials over the past three decades. These failures are partly attributed to uncertainties surrounding the actual presence of infection and delays in intervention, often occurring after patients have progressed to multi-organ failure, beyond the optimal therapeutic window. Early intervention, potentially even preemptive strategies, is crucial for the success of immunomodulatory and other therapies, mirroring the effectiveness demonstrated in preclinical models. Conversely, the overuse of antibiotics, driven by diagnostic uncertainty, fuels antimicrobial resistance and can misdirect clinicians from considering non-infectious conditions.
The development of rapid and point-of-care biomarkers for infection and sepsis, characterized by high sensitivity and specificity, is a major area of focus. Current approaches encompass pathogen-centric techniques, such as molecular identification of pathogens or their unique components like prokaryotic DNA, and host-response investigations employing omics technologies, single or multiplex protein assays, or flow cytometry. However, many host-response studies lack robust comparator groups, particularly patients with non-infectious critical illnesses or definitive microbiological confirmation of infection. Ideally, patient cohorts should enable the accurate presymptomatic identification of individuals at risk of developing infection, especially those progressing to sepsis.
This pioneering study aimed to create a tool for presymptomatic identification of patients developing postoperative infections. The hypothesis was that transcriptional changes in small gene sets could serve as indicators. A secondary objective was to determine if these changes could also identify infected patients at risk of developing new-onset organ dysfunction, indicative of sepsis. To achieve these goals, a large-scale, prospective, multi-center study was conducted on patients undergoing elective major surgery. Daily blood samples and data were collected, commencing preoperatively and continuing for up to a week post-surgery. An independent clinical adjudication panel rigorously reviewed clinical and laboratory data to identify patients with confirmed postoperative infection, with or without sepsis. Samples from these patients were then analyzed and compared with postoperative day-, age-, sex-, and procedure-matched patients who experienced either non-infectious systemic inflammation or an uncomplicated postoperative recovery.
Methodology: Unveiling Biomarker Signatures
Study Design and Patient Cohort
This prospective study enrolled elective surgery patients across eight hospitals (seven in the UK and one in Germany) between November 2007 and February 2017. Ethical approval was secured through the Southampton and South-West Hampshire Multicentre Research Ethics Committee, and the protocol achieved US Federal Wide Assurance Independent Review Board status. Informed consent was obtained from all patients aged 18-80 years scheduled for elective major surgery deemed to increase the risk of postoperative infection.
Data and Sample Collection
Demographic data were collected at enrollment, and baseline blood samples were taken 1-7 days before surgery. Postoperatively, daily collections of relevant clinical, laboratory, and imaging data, along with blood samples, were performed until day seven, hospital discharge (if earlier), or a diagnosis of infection or sepsis by the treating clinician. Two 4ml blood aliquots were collected daily into sterile EDTA vacutainers and immediately transferred into RNAse-free vials containing 10.5ml RNAlater® to preserve the transcriptome integrity.
Patient Selection and Adjudication
The initial diagnosis of sepsis was based on the treating clinician’s interpretation of clinical and laboratory markers, using the ‘Sepsis-2’ definition prevalent at the time. This defined ‘sepsis’ as suspected or confirmed infection with two or more systemic inflammatory response syndrome (SIRS) criteria, and ‘severe sepsis’ as sepsis with poorly-characterized new-onset organ dysfunction. However, to align with the updated Sepsis-3 definition, published in 2016, which redefined sepsis as infection plus new organ dysfunction indicated by a ≥2 point increase in the Sequential Organ Failure Assessment (SOFA) score, subsequent analyses adopted this new definition.
Recognizing the potential variability in clinical diagnoses of infection and sepsis, a Clinical Advisory Panel independently adjudicated cases to ensure confident identification of patients with postoperative infection. Patients with definite infection were categorized into either an uncomplicated infection subgroup or a sepsis subgroup if they developed new organ dysfunction post-surgery, indicated by a SOFA score increase of ≥2 points from baseline. Postoperative day-, age-, sex-, and procedure-matched cohorts of non-infected patients experiencing uncomplicated postoperative recovery (SIRS-) or developing a systemic inflammatory response (SIRS+) were selected from the remaining study participants. Blood samples from these three groups, totaling 453 patients, were selected for transcriptomic and subsequent reverse transcription-quantitative polymerase chain reaction (RT-qPCR) analyses.
Transcriptomic and RT-qPCR Analysis
Infected patients were divided into Discovery (Gene Feature Selection) (n=63) and Training and Validation (Classification) (n=91) cohorts (Fig. 1).
Figure 1: Study schema outlining the Discovery (Gene Feature Selection) and Training/Validation (Classification) phases. RT-qPCR: Reverse Transcription quantitative polymerase chain reaction, SIRS: systemic inflammatory response syndrome, Inf+: infected patients, Inf-: non-infected patients; Inf+ OD-: uncomplicated infection patients. This image illustrates the rigorous approach taken to identify and validate biomarker signatures for postoperative diagnosis.
Discovery Cohort: Gene Feature Selection
Biomarker discovery was initially conducted in the 63-patient Discovery cohort at a pre-determined interim analysis point. Comprehensive transcriptomic analysis was performed using globin-reduced RNA from blood samples of 58 patients collected in the three days preceding the clinical presentation of definite infection, and from 55 matched non-infected (SIRS-) control patients (Fig. 1). Microarray analysis using Human HT-12v4 bead arrays (Illumina) was employed. Differentially expressed genes (DEGs) were identified using a linear model fit for each gene, a p-value cutoff of 0.05, and a fold change threshold of at least 1.2, with non-infected study patients serving as the reference group. The gene expression of the top 80 DEGs was validated using multiplex RT-qPCR (Fluidigm) and TaqMan Gene Expression Assays (Applied Biosystems).
The RT-qPCR data were subsequently utilized for down-selecting the 25 most promising biomarkers. Analysis was possible for 63 infection and 62 SIRS- patients, and RT-qPCR data were also obtained from 58 SIRS+ matched patients (Fig. 1; Table 1). Within the 63 infected patients, 37 who developed sepsis were compared to 26 with uncomplicated infection (Fig. 1; Table 1). Gene ontology enrichment analysis was performed using the R package cluster profiler and visualized using the R package GoPlot.
Table 1: Patient Demographics and Clinical Metadata
Patient cohort | Infection (n = 154) | Non-Infective SIRS (SIRS+; n = 148) | Non-infected non-SIRS (SIRS-; n = 151) | |
---|---|---|---|---|
Organ Dysfunction+ (n = 98) | Organ Dysfunction− (n = 56) | |||
Feature Selection (n = 37) | Classification (n = 61) | Feature Selection (n = 26) | Classification (n = 30) | |
Age, median (IQR) | 69 (61–72) | 67 (58–74) | 65 (53–73) | 61 (52–73) |
Sex | ||||
Male | 25 | 54 | 15 | 21 |
Female | 12 | 7 | 11 | 9 |
Ethnicity | ||||
White | 37 | 53 | 25 | 29 |
Black | 0 | 3 | 1 | 1 |
South Asian | 0 | 1 | 0 | 0 |
Chinese | 0 | 4 | 0 | 0 |
Type of surgery | ||||
Abdominal | 28 | 50 | 18 | 24 |
Cardiac | 1 | 1 | 0 | 0 |
Gynaeco-urological | 1 | 4 | 3 | 0 |
Vascular | 5 | 4 | 4 | 2 |
Thoracic | 2 | 2 | 0 | 3 |
Other | 0 | 0 | 1 | 1 |
Cancer surgery | 27 | 55 | 18 | 24 |
Day of clinical presentation of infection, median (IQR) | 4 (3–6) | 3.5 (2–5) | 4 (3–6) | 3 (3–5) |
Source of infection | ||||
Skin and soft tissue | 1 | 1 | 3 | 1 |
Blood | 5 | 5 | 3 | 2 |
Chest | 15 | 33 | 10 | 12 |
Biliary | 1 | 1 | 2 | 0 |
Abdominal | 13 | 17 | 6 | 13 |
Urinary tract | 0 | 4 | 0 | 2 |
Unknown | 2 | 0 | 2 | 0 |
Postoperative SOFA score | ||||
Median SOFA score on day of diagnosis for infection or equivalent day post-surgery, Median (IQR) | 5 (2–10) | 4 (0–7) | 0 (0–0) | 0 (0–0) |
Patient outcome | ||||
Sepsis-related death | 2 | 7 | 0 | 0 |
Non-related sepsis death | 0 | 2 | 0 | 0 |
Discharged from hospital alive | 35 | 54 | 26 | 29 |
Remained in hospital >7 days | 29 | 30 | 12 | 16 |
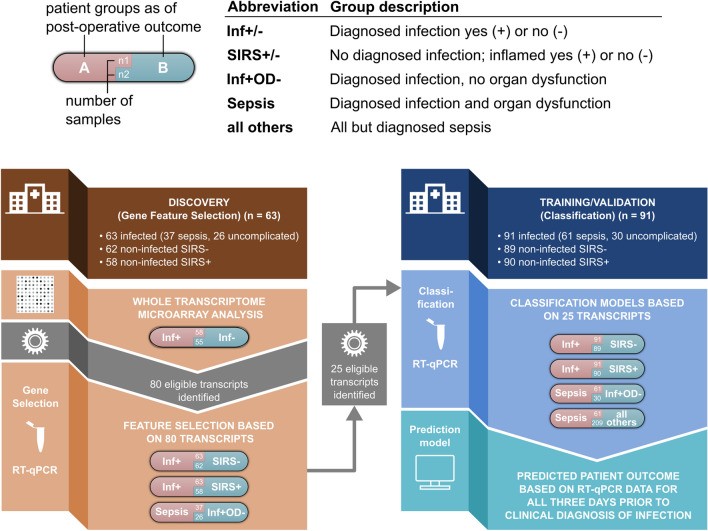
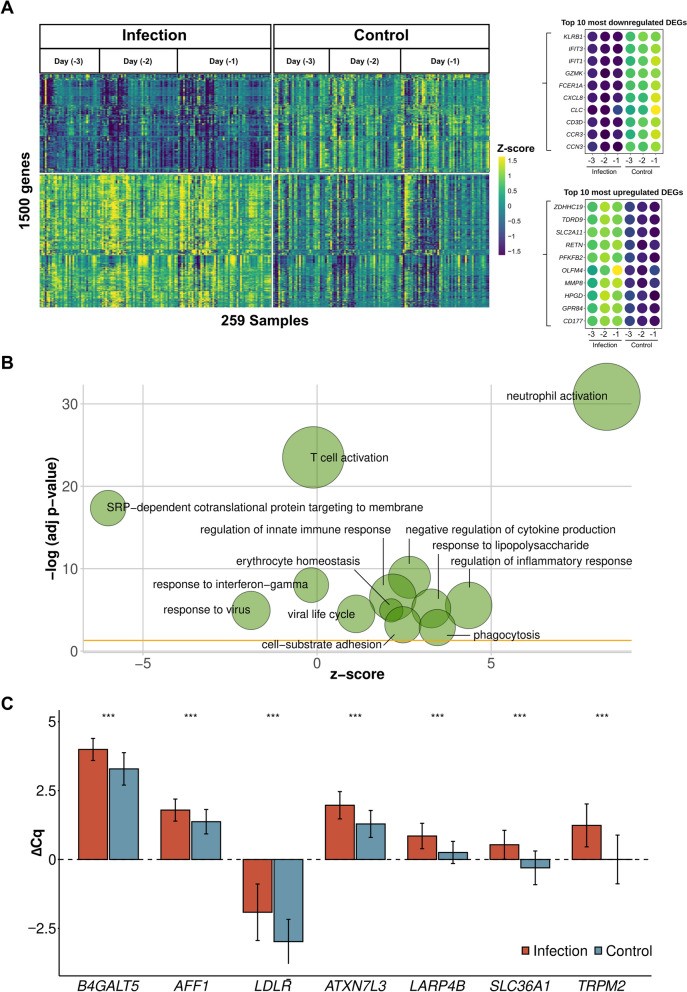
Table 1: Patient demographics and clinical metadata for the study cohorts. This table provides a detailed overview of the patient populations used in the biomarker discovery and validation phases, highlighting the meticulous matching process employed to ensure robust comparisons. IQR: interquartile range, SOFA: Sequential (sepsis-related) organ failure assessment score.
To refine the gene selection process based on RT-qPCR data, a two-step feature selection method was employed to enhance the predictive capacity of classification models used to distinguish patient groups. The Boruta algorithm, a wrapper method based on random forest, was used to select relevant features, followed by backward elimination to identify features with the strongest discriminatory power for specific classifications. Training of these models for feature selection involved 5-fold cross-validation repeated 25 times, tracking mean performances for Area Under the Receiver Operator Curve (AUC), Positive Predictive Value (PPV), and Negative Predictive Value (NPV). This procedure was repeated to assess the biomarkers’ ability to differentiate infected patients from controls (SIRS-) or non-infected but inflamed (SIRS+) patients, and to distinguish between infected patients who developed organ dysfunction (sepsis) and those who did not.
Training and Validation of Classification Models
From the initial 80 DEG biomarker candidates, 25 were identified as part of at least one classification model capable of differentiating patient classes using an orthogonal RT-qPCR approach. These 25 RT-qPCR transcripts were then evaluated in an independent patient cohort (Classification Cohort) to develop a predictive classifier model and assess its performance.
RT-qPCR analysis of gene expression was conducted on samples from 91 infected patients, 89 matched SIRS- comparator patients, and 90 similarly matched non-infected SIRS+ patients (Fig. 1). Further comparisons were made within the infection cohort, contrasting patients who did (sepsis, n=61) and did not develop organ dysfunction (n=30). A final comparison was performed between patients with sepsis (n=61) and all other matched patients with non-sepsis outcomes (n=209) within the validation cohorts (Fig. 1).
To evaluate signature performance, a similar random forest-based approach was utilized. Models were created (training phase) and subsequently validated (validation phase). Data were randomly split into an 80:20 ratio for training and validation, respectively. In the training phase, models were trained and cross-validated on the 80% subset using 5-fold cross-validation, repeated 25 times using the R package caret (‘train’ function). In the validation phase, trained models were tested on the remaining 20% of data not used in training. This process was repeated 10 times with different randomly selected subsets to mitigate bias from data splitting. For each comparison, 10 models (with 10 different test sets) were created, and the mean AUC, sensitivity, and specificity were reported.
Statistical Analysis
Sample size prediction followed the method by Figueroa et al., detailed in Supplement S4. Random forest-based classifier performance was assessed with increasing numbers of simulated patients in each cohort to determine performance plateau. Nonparametric Wilcoxon tests were used for all qRT-PCR data analysis, and all data and statistical analyses were performed using R (v.3.6.3).
Key Findings: Predictive Gene Signatures for Postoperative Diagnosis
Patient Recruitment and Subgroup Identification
Clinical data and blood samples were collected from 4385 high-risk elective surgery patients. Clinical panel adjudication identified 154 patients with high certainty of infection, of whom 98 developed sepsis (Fig. 1). These were compared to samples from postoperative day-, age-, sex-, and operation-matched patients without infection, including 148 who developed a systemic inflammatory response (SIRS+) and 151 who did not (SIRS-). Each patient group was divided into Discovery and Training/Validation cohorts. Demographic and clinical data are presented in Table 1. Microbiological confirmation of infection was obtained in 81 septic and 45 uncomplicated infection patients. A diverse range of Gram-negative, Gram-positive, and fungal organisms were isolated. Strong clinical evidence of infection was used for the remaining 15 and 11 patients, respectively. Of the 98 septic patients, 80 required organ support (mechanical ventilation, vasopressors, or renal replacement therapy). Nine patients died from sepsis, and two from unrelated causes. Approximately 60% of non-infected patients did not receive postoperative antibiotics.
Discovery Cohort Results: Microarray Biomarker Identification
Initial biomarker discovery, using microarray analysis, compared blood samples from 58 infected patients in the three days before clinical presentation of postoperative infection with 55 matched non-infected, non-inflamed (SIRS-) patients (Fig. 1, Table 1).
A total of 2594 differentially expressed genes (DEGs) were identified between infection and control groups in the three days preceding infection diagnosis. Of the top 1500 DEGs with the highest fold change (Fig. 2A, Supplement S8), 863 (57.5%) were upregulated and 637 (42.5%) downregulated. Functional enrichment analysis revealed immune-relevant pathways primarily involving neutrophils and T cells (Fig. 2B, Supplement S8).
Figure 2: Gene expression analysis comparing infection versus healthy postoperative controls. A: Expression profile of 1500 differentially expressed genes with the highest absolute fold change. Top ten up- and downregulated genes are indicated. B: Top enriched Gene Ontology categories based on 1500 differentially expressed genes (Fig. 2A). Node sizes indicate gene number per category. Fold change is indicated for each gene node. C: RT-qPCR values for 8 genes classifying infection versus no infection based on microarray data. ΔCq value indicates PCR cycle quantifications (ΔCq = Cq(reference) – Cq(target gene)). Wilcoxon test significance level indicated (**p≤0.001, ns: not significant). Error bars show standard error of the mean. N(samples of infected patients) = 139, N(samples of control patients) = 144. This figure visually summarizes the key gene expression changes observed in the discovery cohort, providing critical insights for postoperative diagnosis.
A random forest-based algorithm identified the best-performing DEGs for all three days before infection/sepsis diagnosis and their respective non-infected controls. Initial analysis indicated that different sets of eight genes could identify postoperative infection with high AUCs.
RT-qPCR Verification and Biomarker Down-Selection
Differential expression analysis and random forest-based classifiers identified 80 top-performing genes from the microarray data. These were validated by RT-qPCR using the same 58 infected patient samples and samples from five additional patients, compared to 62 non-infected SIRS- patients. A representative 8-gene set showed significant differential expression between the groups, confirming compatibility between microarray and RT-qPCR data (Fig. 2C). To leverage all patient subgroups, the study explored whether subsets of the 80-gene set could differentiate the 63 infected patients from 58 SIRS+ patients, and within the infection cohort between those with (n=37) or without (n=26) organ dysfunction (Fig. 1; Table 1). Random forest-based classification with Boruta feature selection yielded strong model performances. Specifically, a 7-gene set (B4GALT5, AFF1, LDLR, ATXN7L3, LARP4B, SLC36A1, TRPM2, AUC >0.85) for infection vs. SIRS- models, a 12-gene set (ATXN1, SLC41A3, MED13L, STOM, B4GALT5, MIDN, HVCN1, LDLR, CFLAR, SPATA13, EIF4G3, METTL7B, AUC >0.9) for infection vs. SIRS+ models, and an 8-gene set (DOK3, ICAM2, IL1R1, LGALS2, LSG1, RPL13A, RPS13, SGSH, AUC >0.75) for sepsis vs. uncomplicated infection effectively classified postoperative outcomes. These 25 genes were selected for predictive model building in a larger, independent patient cohort.
Training/Validation Cohort: Machine Learning Predictive Models
In independent patient cohorts, classification performance was evaluated for the 7, 12, and 8-gene signatures in discerning: (i) infection (n=91) from non-infected SIRS- (n=89) patients, (ii) infection from non-infected SIRS+ (n=90) patients, and (iii) sepsis (n=61) from uncomplicated infection patients (n=30) (Fig. 1; Table 1).
To prevent overfitting, classification training with nested cross-validation was performed 10 times on randomly selected 80% data subsets. Models were validated on 20% randomly selected sample subsets not used for training. Mean performance across 10 runs remained high using random forest-based classification. For postoperative infection vs. SIRS- controls, the 7-gene signature achieved an AUC of 0.871 (Fig. 3). The 12-gene signature for infection vs. SIRS+ patients achieved an AUC of 0.897. Sepsis vs. uncomplicated infection differentiation yielded an AUC of 0.703. Sepsis vs. all other clinical presentations using all 25 transcripts achieved an AUC of 0.843. Sensitivity was high across comparisons (0.785-0.942), while specificity was high for infected vs. non-infected SIRS+ (0.838) and SIRS- (0.776) patients, but lower for sepsis vs. uncomplicated infection (0.217).
Figure 3: RT-qPCR based classification performance using random forest across all available patient samples and days prior to infection diagnosis. ROC curve and statistical metrics for (i) infection versus non-inflamed postoperative controls (SIRS-) [blue], infection versus non-infected and inflamed (SIRS+) [red], sepsis versus infection without organ dysfunction (OD-) [mauve] and sepsis versus all others [green]. The table reports mean statistics for classification models based on different numbers of transcripts. N: number, AUC: area under the curve, PPV: positive predictive value, NPV: negative predictive value, Sens: sensitivity, Spec: specificity. This figure highlights the robust performance of the identified gene signatures in accurately classifying different postoperative conditions, demonstrating their potential for improving postoperative diagnosis.
Discussion: Implications for Postoperative Diagnosis and Patient Care
This study provides compelling evidence that patients undergoing elective major surgery who subsequently develop postoperative infection, with or without sepsis-related organ dysfunction, can be reliably identified and differentiated from non-infected patients using host transcriptomics up to three days before clinical diagnosis.
Accurate and early postoperative diagnosis of infection and sepsis is paramount for improving patient care and outcomes, and for minimizing unnecessary antimicrobial use. Prior transcriptomic studies in patients with infection and sepsis have primarily focused on those with established clinical features requiring hospitalization or critical care admission. These studies have enhanced our understanding of sepsis pathogenesis, demonstrated prognostic potential, and shown reasonable discrimination between infectious and non-infectious systemic inflammation. Presymptomatic diagnosis, as demonstrated in this study, offers the potential to facilitate earlier investigation and treatment, which is critical for improving patient outcomes.
To the best of our knowledge, this is the first study to specifically investigate patient samples collected before the clinical onset of infection. Elective surgical populations represent an ideal, albeit somewhat inefficient, study group, as most patients experience uncomplicated postoperative courses. To ensure data integrity, only samples from the 3.5% of patients with highly confident postoperative infection diagnoses, as determined by clinical adjudication, were used. Intermediate cases with diagnostic uncertainty were excluded. The large patient cohort also allowed for the selection of postoperative day-, age-, sex-, and operation-matched patients with uncomplicated courses or postoperative systemic inflammation confidently deemed non-infectious and untreated.
Many sepsis biomarker studies have relied on knowledge-based selection approaches based on known biological functions and pathways. In contrast, this study adopted a target selection strategy based on statistical rather than purely biological features. A machine learning approach was used to select targets and classify patients based on host gene expression, comparing against clear-cut clinical diagnoses to determine predictive accuracy. The initial biomarker discovery phase identified 80 genes with confirmed differential expression using RT-qPCR. Re-classification based on RT-qPCR aimed at feature reduction led to the identification of 25 key biomarkers. Subsequent classification analysis demonstrated the potential for clinical application with even smaller biomarker sets, enabling the development of rapid and cost-effective point-of-care tests.
The machine learning algorithm approach successfully classified patients based on host gene expression, achieving high AUC, sensitivity, and specificity values when comparing patients developing infection versus uncomplicated (SIRS-) controls, and also in differentiating infection from the more challenging group of patients with postoperative (SIRS+) inflammation not driven by infection. Reasonable discrimination (high sensitivity but lower specificity) was also achieved in preemptively identifying infected patients who would develop organ dysfunction compared to those with uncomplicated infection.
The unique approach of this study is further highlighted by the limited overlap (six out of 80 genes) between the identified classification model genes and those in previously published gene signatures for sepsis and community-acquired pneumonia. The data suggest that changes in host biomarker expression are predictive of later infection-related complications. Limitations in model performance may arise from the complex interplay of individual genes, transcripts, post-translational modifications, and protein interactions, further complicated by therapeutic interventions and the dynamic nature of host response signaling.
By sampling at multiple time points, this study addressed the challenging question of predicting when an individual will develop infection or sepsis. While a common gene set could detect changes over a 3-day period before clinical presentation, day-specific gene transcripts showed even stronger signals (data not shown). However, using separate gene sets in clinical practice would be impractical due to the lack of prior knowledge about the precise timing of infection or sepsis development.
Several limitations warrant consideration. Cost constraints limited broader testing, particularly within the indeterminate subgroup with diagnostic uncertainty. Internal model validation used 20% patient subsets, repeated randomly 10 times. Prospective external validation is crucial to confirm predictive utility in surgical and non-surgical populations, specific patient subsets (e.g., children, hematology-oncology), diverse racial backgrounds, and non-bacterial infections. A validation study in acute lower respiratory tract infection patients is ongoing.
If validated as reliable tools, the clinical implementation of these gene panels will depend on cost and result turnaround time. Point-of-care PCR-based devices and multiplex protein analyses can provide results within 1-2 hours or minutes, respectively, at relatively low costs, with prices expected to decrease further with technological advancements. If cost-comparable to routine inflammation biomarkers like C-reactive protein and procalcitonin, such tests could offer (near-) daily ‘rule-out’ screening. Alternatively, with adjusted cut-off values, they could enhance specificity for diagnostic ‘rule-in’ in clinically suspected but uncertain infection cases, or serve as a ‘rule-out’ test in patients with non-specific symptoms. Furthermore, the high sensitivity in discriminating future uncomplicated infection from sepsis offers potential for early, safe discharge of low-risk patients from higher dependency settings.
Conclusion: Paving the Way for Presymptomatic Postoperative Diagnosis
In conclusion, this study demonstrates that postoperative infection can be identified by a panel of gene transcripts up to three days before clinical presentation. This identification can differentiate infection from both uncomplicated postoperative courses and postoperative systemic inflammation unrelated to infection. Furthermore, infected patients at risk of developing sepsis-related organ dysfunction can also be identified in advance. Subject to prospective validation, these identified gene sets offer a promising approach to reliably diagnose infection and sepsis presymptomatically, potentially improving patient outcomes and reducing inappropriate antibiotic use, marking a significant advancement in postoperative diagnosis.